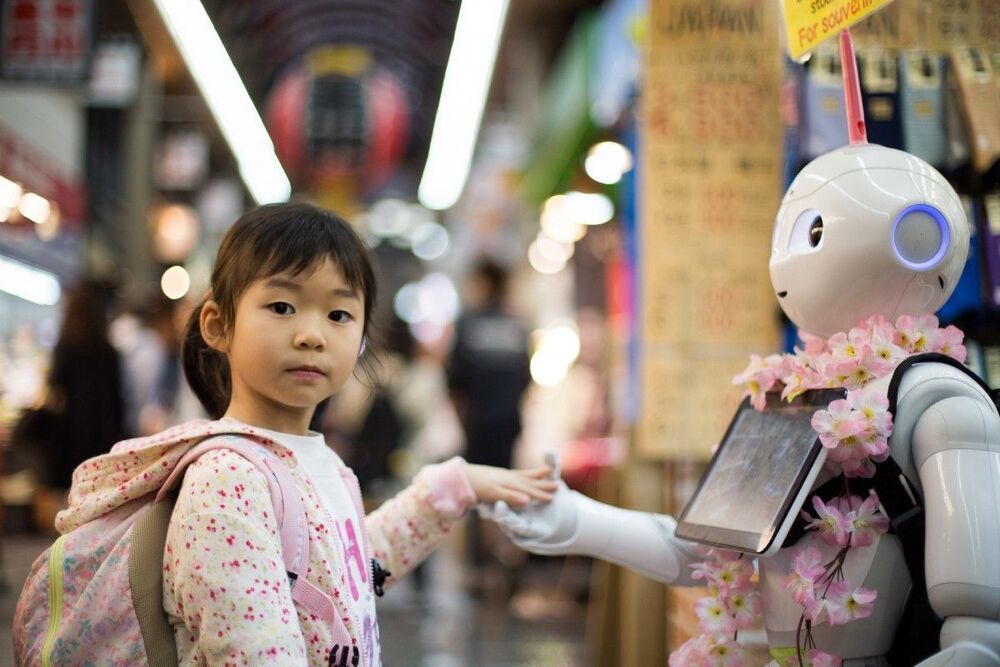
How do you *feel* about that?
Much of today’s discussion around the future of artificial intelligence is focused on the possibility of achieving artificial general intelligence. Essentially, an AI capable of tackling an array of random tasks and working out how to tackle a new task on its own, much like a human, is the ultimate goal. But the discussion around this kind of intelligence seems less about if and more about when at this stage in the game. With the advent of neural networks and deep learning, the sky is the actual limit, at least that will be true once other areas of technology overcome their remaining obstructions.
For deep learning to successfully support general intelligence, it’s going to need the ability to access and store much more information than any individual system currently does. It’s also going to need to process that information more quickly than current technology will allow. If these things can catch up with the advancements in neural networks and deep learning, we might end up with an intelligence capable of solving some major world problems. Of course, we will still need to spoon-feed it since it only has access to the digital world, for the most part.
If we desire an AGI that can consume its own information, there are a few more advancements in technology that only time can deliver. In addition to the increased volume of information and processing speed, before any AI will be much use as an automaton, it will need to possess fine motor skills. An AGI with control of its own faculty can move around the world and consume information through its various sensors. However, this is another case of just waiting. It’s also another form of when not if these technologies will catch up to the others. Google has successfully experimented with fine motor skills technology. Boston Dynamics has canine robots with stable motor skills that will only improve in the coming years. Who says our AGI automaton needs to stand erect?