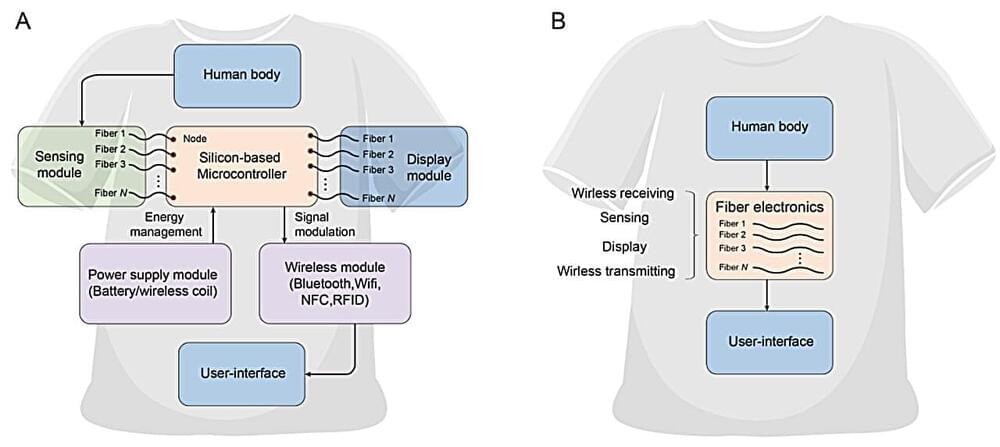
A team of materials scientists and engineers from Donghua University, in China, and the National University of Singapore, has developed a type of fiber that does not rely on chips or batteries to convert visual signals to digital transmissions as it interacts with the human body.
The paper is published in the journal Science. Yunzhu Li and Yiyue Luo with the University of Illinois Urbana-Champaign and MIT, respectively, have published a Perspective piece in the same journal issue outlining the work done by the team on this new effort.
Over the past several years, scientists have been trying to find a way to integrate electronic devices with textiles for such applications as clothes that can display colors, patterns or even messages. Unfortunately, previous efforts involve adding stiff batteries and chips to materials, making them too uncomfortable to wear. In this new effort, the researchers have found a way to get around these problems.