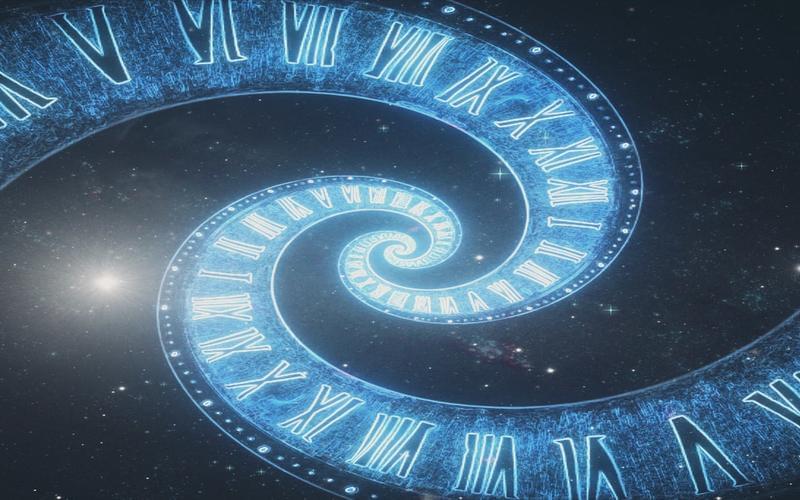
The received view in physics is that the direction of time is provided by the second law of thermodynamics, according to which the passage of time is measured by ever-increasing disorder in the universe. This view, Julian Barbour argues, is wrong. If we reject Newton’s faulty assumptions about the existence of absolute space and time, Newtonian dynamics can be shown to provide a very different arrow of time. Its direction, according to this theory, is given by the increase in the complexity and order of a system of particles, exactly the opposite of what the received view about time suggests.
Two of the most established beliefs of contemporary cosmology are that the universe is expanding and that the direction of the arrow of time in the universe is defined by ever-increasing disorder (entropy), as described by the second law of thermodynamics. But both of these beliefs rest on shaky ground. In saying that the universe is expanding, physicists implicitly assume its size is measured by a rod that exists outside the universe, providing an absolute scale. It’s the last vestige of Newton’s absolute space and should have no place in modern cosmology. And in claiming that entropy is what gives time its arrow, physicists uncritically apply the laws of thermodynamics, originally discovered through the study of steam engines, to the universe as a whole. That too needs to be questioned.
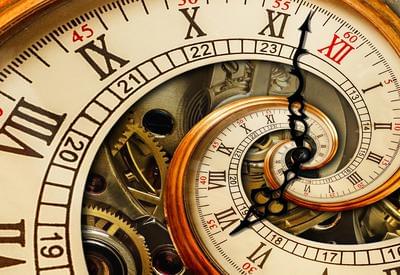
Einstein and why the block universe is a mistake Read more In the absence of an absolute space and external measuring rods, size is always relative — relative to a measure of distance internal to the system. Starting from the simplest case, a triangle, what we find is that the internal measure of size produces a ratio which also happens to be related to a mathematical measure of complexity that intriguingly plays the central role in Newtonian universal gravitation. Applying these findings to the universe as a whole, we find that Newton’s theory of gravity, contrary to what physicists believe, contains within it an intrinsic arrow of time. This provides a strong hint that the direction of time is not defined by an increase in entropy, but by an increase in structure and complexity.