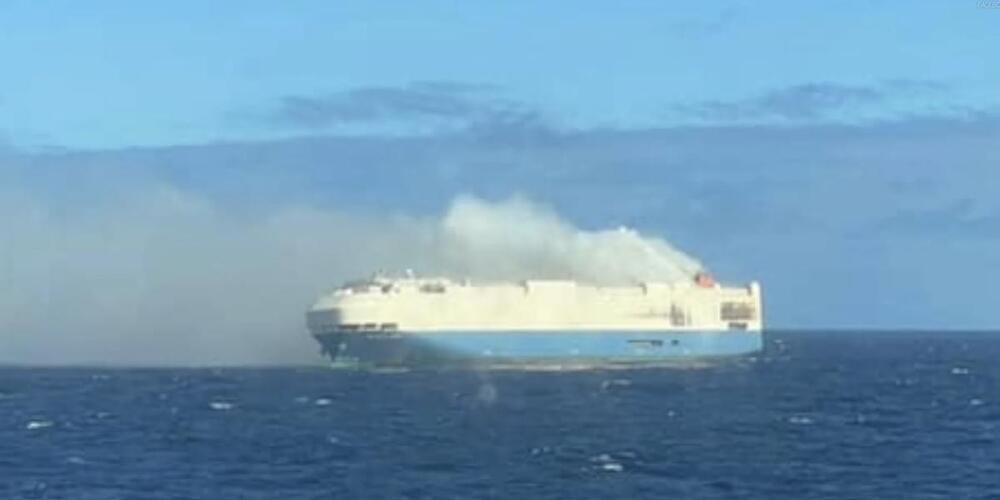
Thousands of cars—including Porsches, Volkswagens, and Lamborghinis—have gone down with the giant cargo ship Felicity Ace, which had been on fire in the Atlantic Ocean for nearly two weeks. A salvage operation was underway to take the roll-on-roll-off car carrier to a safe area off the Azores when it sank on Tuesday morning. The fire was out by the time the ship went under the waves.
The weather had been rough at the time, a spokesperson for Felicity Ace’s Japanese operator, Mitsui O.S.K. Lines transportation company, told Bloomberg. The ship sank after listing to starboard around 220 nautical miles off the Portuguese Azores archipelago at around 9 a.m. local time on March 1. Salvage craft remain posted at the site of the sinking to monitor the situation, according to a press release from the Felicity Ace Incident Information Centre.