A recently granted South African patent seems fairly mundane, but, he inventor is not a human being – it is an AI system named DABUS. property= description.
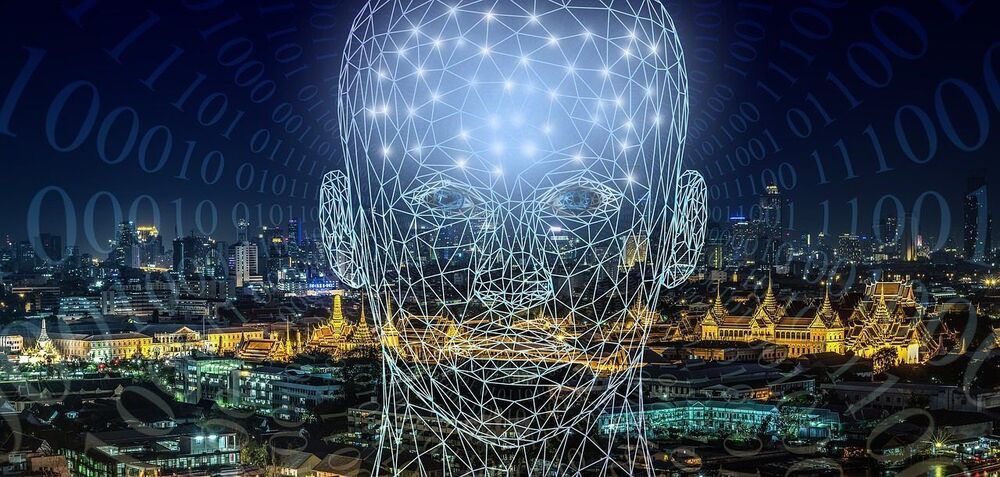
Chameleons have long been a symbol of adaptation because of their ability to adjust their iridophores—a special layer of cells under the skin—to blend in with their surroundings.
In a new study published today in Nature Communications, researchers from South Korea have created a robot chameleon capable of imitating its biological counterpart, paving the way for new artificial camouflage technology.
Since 1,988 and formation of the Posthuman Movement, and articles by early adopters like Max Moore were a sign our message was being received — although I always argued on various Extropian & Transhuman bulletin boards & Yahoo groups &c that “Trans” was a redundant middle and we should move straight to Posthuman, now armed with the new MVT knowledge (also figures on the CDR). There will be a new edition of World Philosophy, the first this millennium, to coincided with various Posthuman University events later this year. Here is the text:
THE EXTROPIAN PRINCIPLES V. 2.01 August 7 1992.
Max More Executive Director, Extropy Institute.
1. BOUNDLESS EXPANSION — Seeking more intelligence, wisdom, and.
personal power, an unlimited lifespan, and removal of natural, social.
biological, and psychological limits to self-actualization and self-realization. Overcoming limits on our personal and social.
progress and possibilities. Expansion into the universe and infinite existence.
2. SELF-TRANSFORMATION — A commitment to continual moral.
intellectual, and physical self-improvement, using reason and critical.
thinking, personal responsibility, and experimentation. Biological and.
neurological augmentation.
3. INTELLIGENT TECHNOLOGY — Applying science and technology to.
transcend “natural” limits imposed by our biological heritage and environment.
4. SPONTANEOUS ORDER — Promotion of decentralized, voluntaristic.
For drone racing enthusiasts. 😃
If you follow autonomous drone racing, you likely remember the crashes as much as the wins. In drone racing, teams compete to see which vehicle is better trained to fly fastest through an obstacle course. But the faster drones fly, the more unstable they become, and at high speeds their aerodynamics can be too complicated to predict. Crashes, therefore, are a common and often spectacular occurrence.
But if they can be pushed to be faster and more nimble, drones could be put to use in time-critical operations beyond the race course, for instance to search for survivors in a natural disaster.
Now, aerospace engineers at MIT have devised an algorithm that helps drones find the fastest route around obstacles without crashing. The new algorithm combines simulations of a drone flying through a virtual obstacle course with data from experiments of a real drone flying through the same course in a physical space.
VLADIVOSTOK, Russia — To see Russia’s ambitions for its own version of Silicon Valley, head about 5,600 miles east of Moscow, snake through Vladivostok’s hills and then cross a bridge from the mainland to Russky Island. It’s here — a beachhead on the Pacific Rim — that the Kremlin hopes to create a hub for robotics and artificial intelligence innovation with the goal of boosting Russia’s ability to compete with the United States and Asia.
On Russia’s Pacific shores, the Kremlin is trying to build a beachhead among the Asian tech powers.