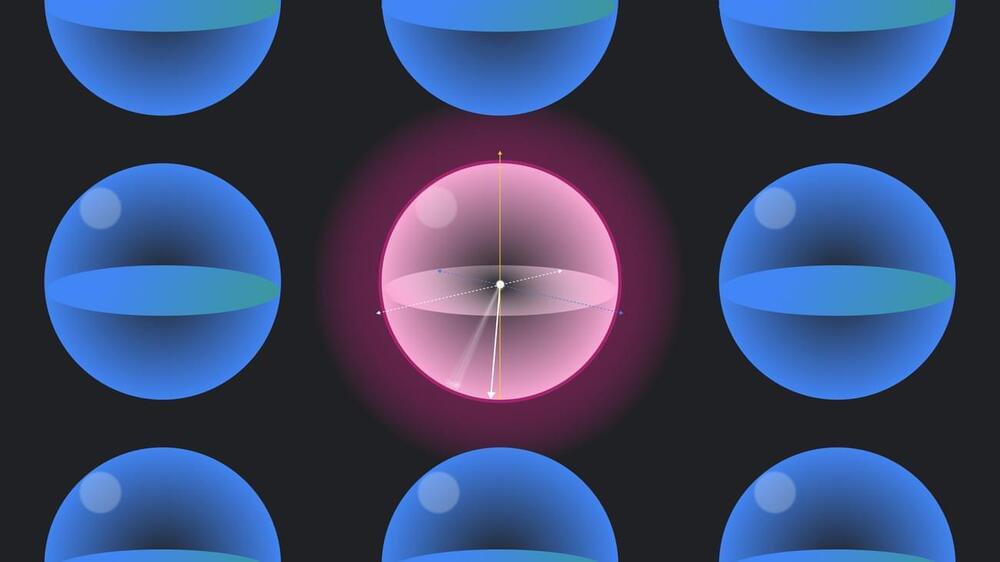
AlphaQubit: an AI-based system that can more accurately identify errors inside quantum computers.
AlphaQubit is a neural-network based decoder drawing on Transformers, a deep learning architecture developed at Google that underpins many of today’s large language models. Using the consistency checks as an input, its task is to correctly predict whether the logical qubit — when measured at the end of the experiment — has flipped from how it was prepared.
We began by training our model to decode the data from a set of 49 qubits inside a Sycamore quantum processor, the central computational unit of the quantum computer. To teach AlphaQubit the general decoding problem, we used a quantum simulator to generate hundreds of millions of examples across a variety of settings and error levels. Then we finetuned AlphaQubit for a specific decoding task by giving it thousands of experimental samples from a particular Sycamore processor.
When tested on new Sycamore data, AlphaQubit set a new standard for accuracy when compared with the previous leading decoders. In the largest Sycamore experiments, AlphaQubit makes 6% fewer errors than tensor network methods, which are highly accurate but impractically slow. AlphaQubit also makes 30% fewer errors than correlated matching, an accurate decoder that is fast enough to scale.