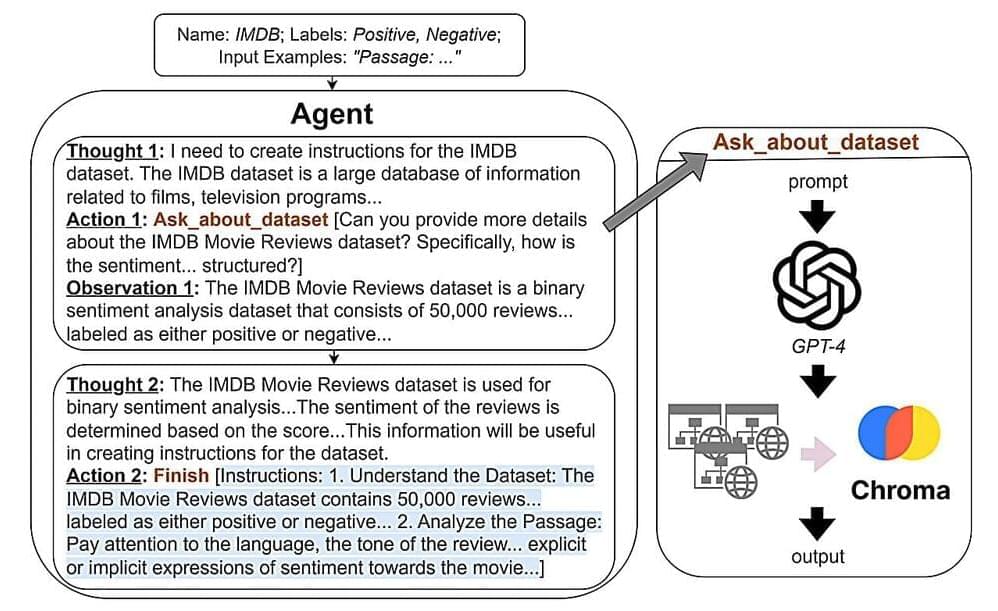
The large language models that have increasingly taken over the tech world are not “cheap” in many ways. The most prominent LLMs, such as GPT-4, took some $100 million to build in the form of legal costs of accessing training data, computational power costs for what could be billions or trillions of parameters, the energy and water needed to fuel computation, and the many coders developing the training algorithms that must run cycle after cycle so the machine will “learn.”
But, if a researcher needs to do a specialized task that a machine could do more efficiently and they don’t have access to a large institution that offers access to generative AI tools, what other options are available? Say, a parent wants to prep their child for a difficult test and needs to show many examples of how to solve complicated math problems.
Building their own LLM is an onerous prospect for costs mentioned above, and making direct use of the big models like GPT-4 and Llama 3.1 might not immediately be suited for the complex reasoning in logic and math their task requires.