Apple patched zero-day flaws exploited to deploy Paragon’s Graphite spyware targeting journalists and civil society, raising global spyware concerns.
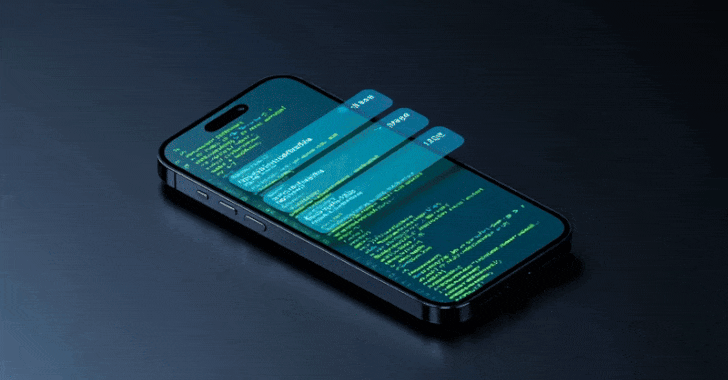
Satya Nadella talks with NVIDIA CEO Jensen Huang at Microsoft Build 2025.Subscribe to Microsoft on YouTube here: https://aka.ms/SubscribeToYouTubeFollow us o…
In a new study, researchers carried out the most extensive coordinated comparison of optical clocks to date by operating clocks and the links connecting them simultaneously across six countries. Spanning thousands of kilometers, the experiment represents a significant step toward redefining the second and ultimately establishing a global optical time scale.