This book explores the psychological impact of advanced forms of artificial intelligence. How will it be to live with a superior intelligence? How will the exposure to highly developed artificial intelligence (AI) systems change human well-being? With a review of recent advancements in brain–computer interfaces, military AI, Explainable AI (XAI) and digital clones as a foundation, the experience of living with a hyperintelligence is discussed from the viewpoint of a clinical psychologist. The theory of universal solicitation is introduced, i.e. the demand character of a technology that wants to be used in all aspects of life. With a focus on human experience, and to a lesser extent on technology, the book is written for a general readership with an interest in psychology, technology and the future of our human condition. With its unique focus on psychological topics, the book offers contributions to a discussion on the future of human life beyond purely technological considerations.
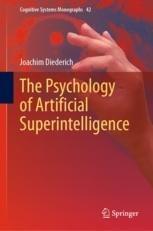