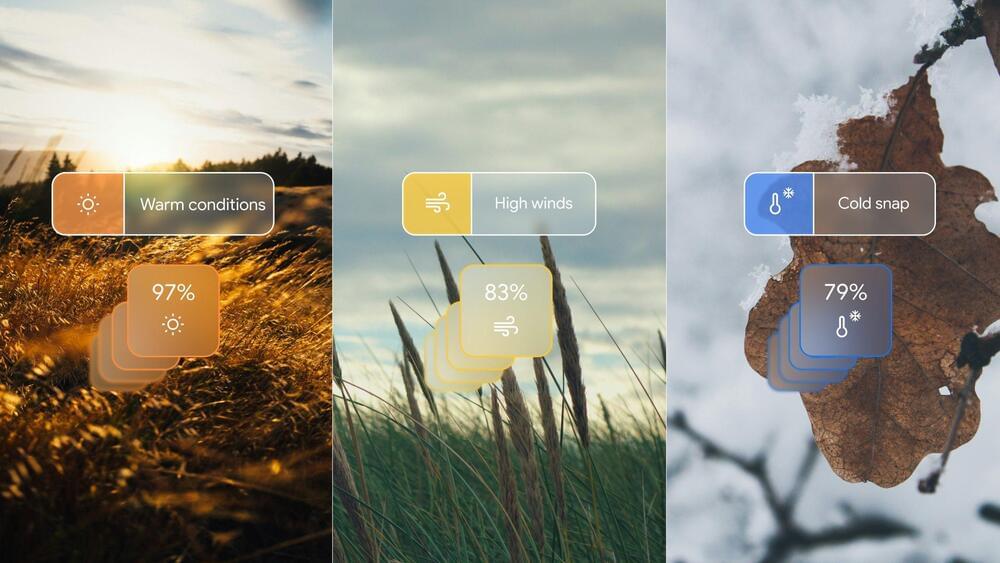
Accurately forecasting weather remains a complex challenge due to the inherent uncertainty in atmospheric dynamics and the nonlinear nature of weather systems. As such, methodologies developed ought to reflect the most probable and potential outcomes, especially in high-stakes decision-making over disasters, energy management, and public safety. While numerical weather prediction (NWP) models offer probabilistic insights through ensemble forecasting, they are computationally expensive and prone to errors. Although ML models have been very promising in giving faster and more accurate predictions, they fail to represent forecast uncertainty, especially in extreme events. This makes ML-based models less useful in actual real-world applications.
The physics-based ensemble models, for example, the ENS from the European Centre for Medium-Range Weather Forecasts (ECMWF), rely on these simulations to produce probabilistic forecasts. These models properly represent the forecast distributions and joint spatiotemporal dependencies and require high computational resources and manual engineering. Conversely, the ML-based method, like GraphCast or FourCastNet, focuses only on deterministic forecasts and will minimize the errors in the mean outcome without considering any uncertainty. None of the attempts to generate probabilistic ensembles by MLWP produced realistic samples or competed with the accuracy of operational ensemble forecasts. Hybrid approaches like NeuralGCM embed ML-based parameterizations within traditional frameworks but have poor resolution and limited performance.
Researchers from Google DeepMind released GenCast, a probabilistic weather forecasting model that generates accurate and efficient ensemble forecasts. This machine learning model applies conditional diffusion models to produce stochastic trajectories of weather, such that the ensembles consist of the entire probability distribution of atmospheric conditions. In systematic ways, it creates forecast trajectories by using the prior states through autoregressive sampling and uses a denoising neural network, which is integrated with a graph-transformer processor on a refined icosahedral mesh. Utilizing 40 years of ERA5 reanalysis data, GenCast captures a rich set of weather patterns and provides high performance. This feature allows it to generate a 15-day global forecast at 0.25° resolution within 8 minutes, which is state-of-the-art ENS in terms of both skill and speed.