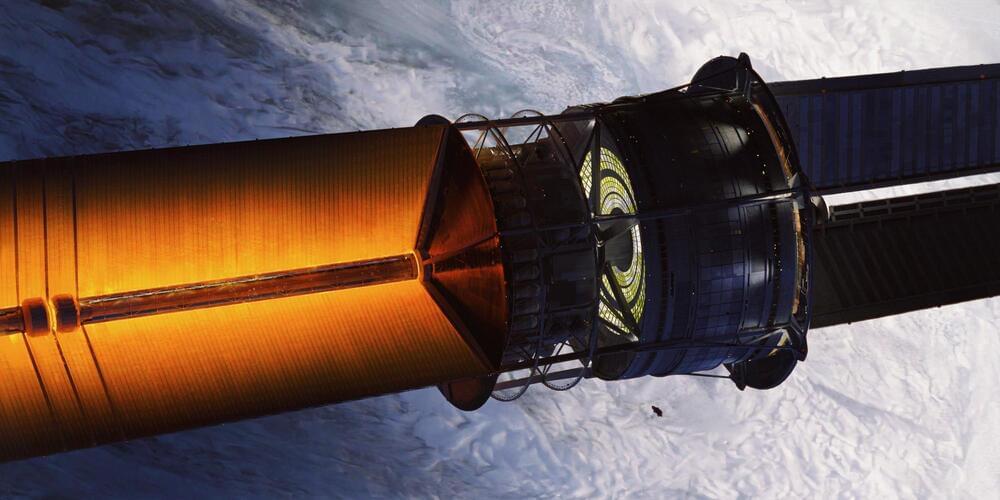
Humanity’s fascination with the unknown is a timeless impulse, rooted in curiosity and the desire to push boundaries, uncover mysteries, and open doors to new frontiers. What were once represented by voyages and the discovery of new islands and continents are now pursued in the vastness of the Universe. As we seek answers, provoke new questions, and open doors to endless possibilities, this drive continues to inspire. It has shaped countless literary and cinematic works, transforming interstellar exploration from a science fiction concept into a vision increasingly grounded in reality. One such visionary project is Project Hyperion, spearheaded by the Initiative for Interstellar Studies (i4is), which challenges humanity to develop practical solutions for interstellar travel through a design competition. By envisioning generation ships—vast, self-sustaining habitats capable of supporting multigenerational societies on journeys spanning centuries—the project not only pushes the boundaries of technology but also sparks social innovation, stretching the limits of our collective imagination.
The exploration of outer space, which began during the Cold War space race with milestones like the launch of Sputnik in 1957 and the Apollo 11 Moon landing in 1969, has driven advances in science, technology, and geopolitics. Since then, continuous efforts such as the International Space Station (ISS), launched in 1998, have provided platforms for microgravity experiments essential to research in biomedicine and physics, as well as preparation for lunar and Martian missions. Simultaneously, spacecraft have evolved from orbital missions to interplanetary exploration and, more recently, space tourism, with vehicles like SpaceX’s Crew Dragon and Blue Origin’s New Shepard offering unique experiences in space.
However, the challenge of interstellar exploration—journeys beyond our solar system to distant stars—presents far greater complexity and requires a radical reimagining of space technology. An interstellar spacecraft would not simply be a scaled-up version of today’s spaceships but a structure capable of sustaining journeys lasting centuries, traversing immense distances. To endure such long voyages, these ships must be self-sustaining, with closed-loop life support systems, food production, and resource recycling, creating an environment where people are born, live, and die. Beyond technological challenges, there are also social and psychological hurdles to prolonged space travel. Such a ship must be not only a high-performance machine but also a viable habitat for living, working, and fostering a society across generations. This requires rethinking how we organize coexistence, social relationships, and power dynamics in an isolated and confined environment.