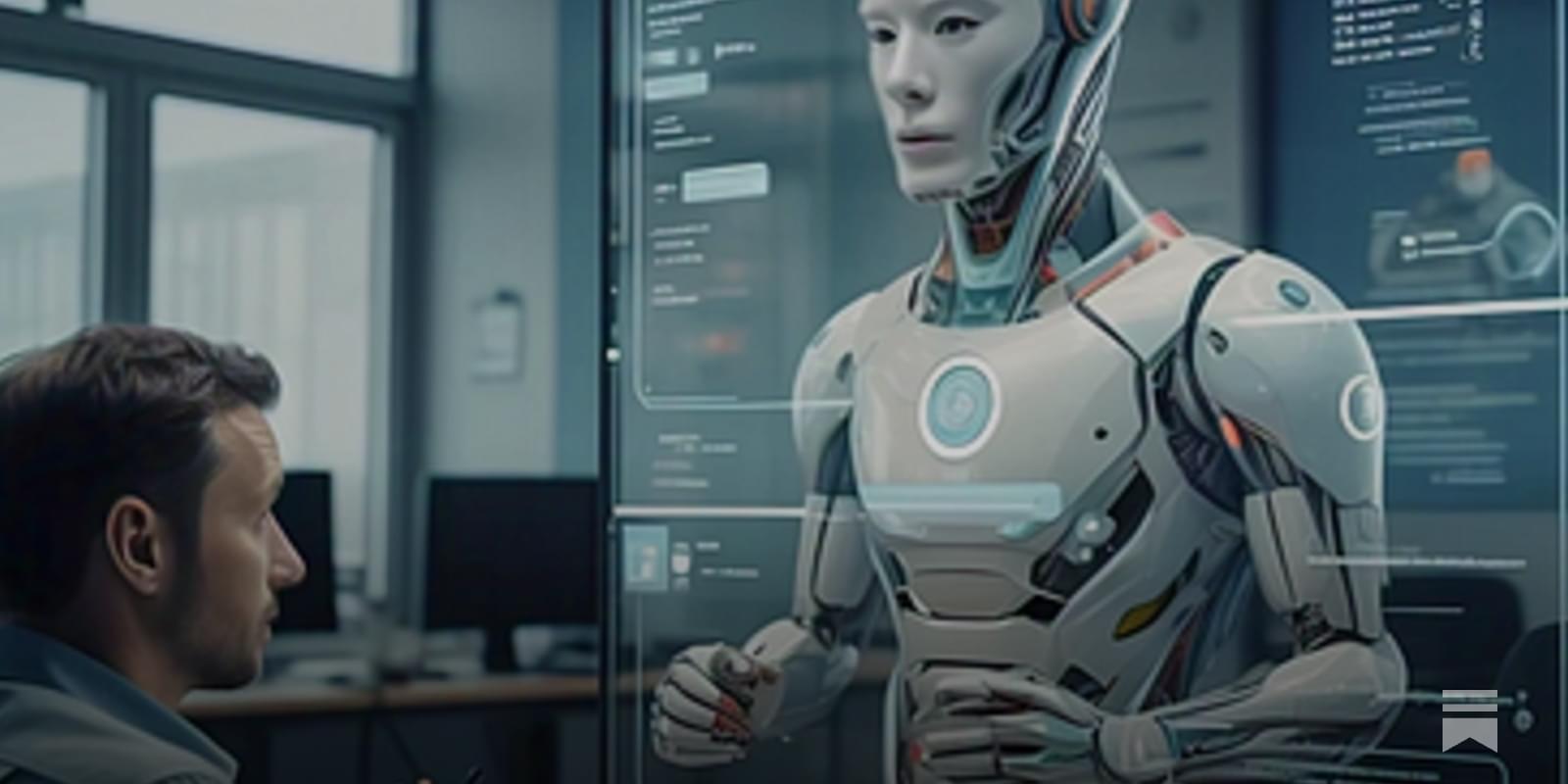
In today’s AI news, OpenAI released its o3-mini model one week ago, offering both free and paid users a more accurate, faster, and cheaper alternative to o1-mini. Now, OpenAI has updated the o3-mini to include an updated chain of thought.
In other advancements, Hugging Face and Physical Intelligence have quietly launched Pi0 (Pi-Zero) this week, the first foundational model for robots that translates natural language commands directly into physical actions. “Pi0 is the most advanced vision language action model,” said Remi Cadene, a research scientist at Hugging Face.
S Luxo Jr., Apple And, one year later the Rabbit R1 is actually good now. It launched to reviews like “avoid this AI gadget”, but 12 months have passed. Where is the Rabbit R1 now? Well with a relentless pipeline of updates and novel AI ideas…it’s actually pretty good now!?
In videos, Moderator Shirin Ghaffary (Reporter, Bloomberg News) leads a expert panel which includes; Chase Lochmiller (Crusoe, CEO) Costi Perricos (Deloitte, Global GenAI Business Leader) Varun Mohan (Codeium, Co-Founder and CEO) that ask, how are we building the infrastructure to support this massive global technological revolution?
Meanwhile, Humans are terrible at detecting lies, says psychologist Riccardo Loconte… but what if we had an AI-powered tool to help? He introduces his team’s work successfully training an AI to recognize falsehoods.