Have you heard of the Dead Internet Theory that’s been circling online (ironically) lately? If not, brace yourself…
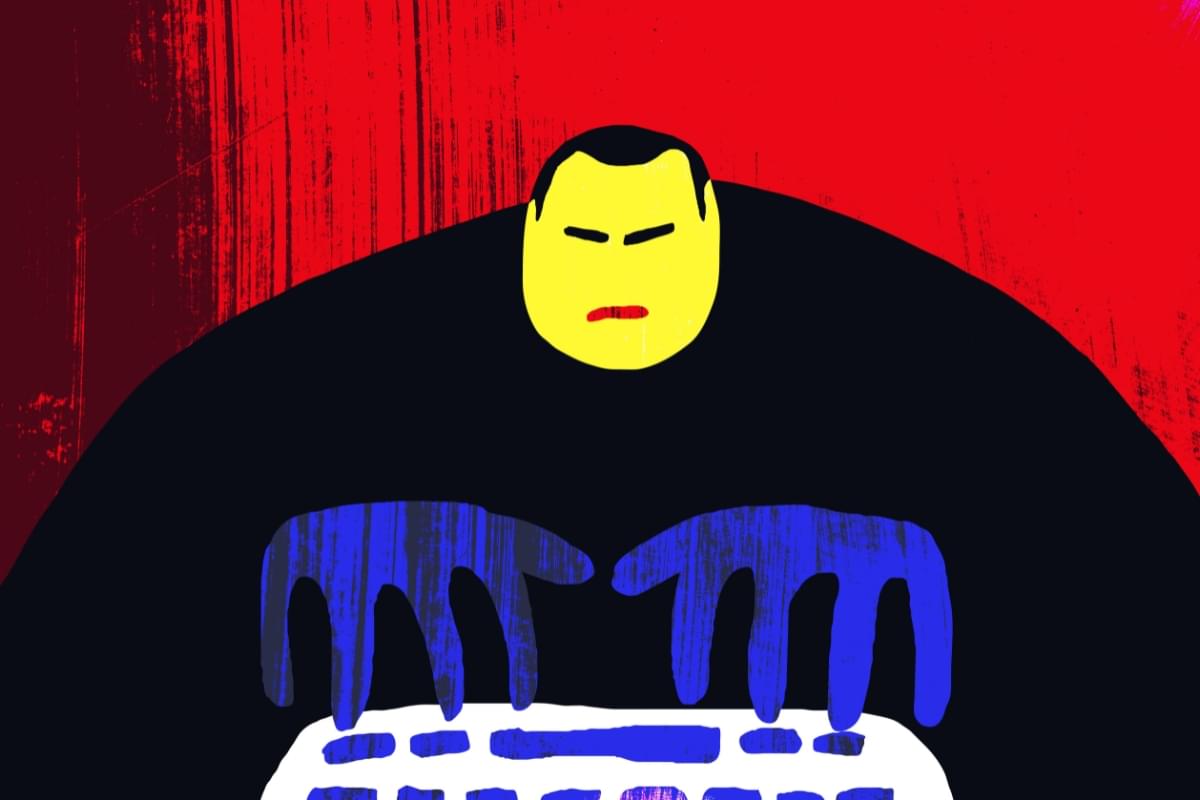
That statement, now signed by twice as many concerned citizens, warned about the risk of human extinction from AI, which was perhaps a bit of an overreach, because … well, extinction? Come on! That’s just a movie with Arnold Schwarzenegger.
What they should have warned about was jobs — the redundancy and destitution of most of humanity, unless there’s some kind of universal income funded by taxes on robots.
What no-one talks about, as the AI revolution unfolds in stock market hype and scientific gung-ho, is what they’re all really trying to do.
For decades there has been near constant progress in reducing the size, and increasing the performance, of the circuits that power computers and smartphones. But Moore’s Law is ending as physical limitations – such as the number of transistors that can fit on a chip and the heat that results from packing them ever more densely – are slowing the rate of performance increases. Computing capacity is gradually plateauing, even as artificial intelligence, machine learning and other data-intensive applications demand ever greater computational power.
Novel technologies are needed to address this challenge. A potential solution comes from photonics, which offers lower energy consumption and reduced latency than electronics.
One of the most promising approaches is in-memory computing, which requires the use of photonic memories. Passing light signals through these memories makes it possible to perform operations nearly instantaneously. But solutions proposed for creating such memories have faced challenges such as low switching speeds and limited programmability.
Crowdsourcing treatments that work — yael elish — CEO & founder, stuffthatworks.
Yael Elish is CEO and Founder of StuffThatWorks (https://www.stuffthatworks.health/), a company that offers an online platform where people suffering from chronic diseases can share information to learn which treatments work best for their specific condition, based on the experience of their peers combined with a smart, AI-based crowdsourcing system.
A passionate entrepreneur with expertise in crowdsourcing and consumer-facing products, Yael was on the Waze founding team, where she drove the overall product strategy that led the company from User One to one of the world’s most notable crowdsourcing endeavours. She also co-founded eSnips and NetSnippet, and was part of the senior management team that took Commtouch to its successful NASDAQ IPO in 2000.
Prior to Commtouch, Yael led the sales and marketing efforts for various start-ups in Israel.
Yael holds a first degree in Foreign Relations from the Hebrew University of Jerusalem, Israel.
Electric sparks are used for welding, powering electronics, killing germs or for igniting the fuel in some car engines. Despite their usefulness, they are hard to control in open space—they split into chaotic branches that tend to go toward the closest metallic objects.
A recent study published in Science Advances uncovers a way of transporting electricity through air by ultrasonic waves. The level of control of the electric sparks allows guidance of the spark around obstacles, or guiding it to hit specific spots, even in non-conductive materials.
“We observed this phenomenon more than one year ago, then it took us months to control it, and even longer to find an explanation,” says Dr. Asier Marzo from the Public University of Navarre, lead researcher of the work.
Scientists have developed a novel tool designed to protect and conserve coral reefs by providing them with an abundance of feeding opportunities.
The device, dubbed the Underwater Zooplankton Enhancement Light Array (UZELA), is an autonomous, programmable underwater light that works to draw in nearby zooplankton, microscopic organisms that coral feed on.
After testing the submersible on two species of coral native to Hawaii over six months, researchers found that UZELA could greatly enhance local zooplankton density and increase the feeding rates of both healthy and bleached coral. Importantly, providing coral with greater amounts of food makes them stronger and more likely to be resilient against certain environmental threats, like heat stress or ocean acidification.
An interesting paper where Schuette et al. develop a generative diffusion-based AI model for predicting the 3D structure of chromatin. Their model takes chromatin accessibility sequence data as input and outputs a statistical distribution of predicted 3D chromatin structures. Remarkably, their model generalizes across cell types, making it broadly useful! #computationalbiology #ai #generativeai
Computational approaches for predicting chromatin conformations de novo using only sequencing data remain scarce. Compared to existing polymer simulation–based prediction approaches, ChromoGen maintains unique advantages. The generative nature of ChromoGen enables efficient production of statistically independent samples, thus avoiding the inefficient navigation of state space that polymer simulations require to produce a diverse set of conformations. Moreover, ChromoGen’s transformer-based front end provides additional advantages, extracting features from sequencing data and placing the information in low-dimensional embeddings that the diffusion model handles efficiently. This powerful design markedly reduces the computational cost of each diffusion step, providing a practical means to achieve cell type–specific de novo predictions with the full benefit of DNA sequence and chromatin accessibility data. In contrast, incorporating DNA sequence information into polymer models has long been a challenging task that is often indirectly addressed by incorporating various histone marks.
In its current form, ChromoGen can be immediately applied to any cell type with available DNAse-seq data, enabling a vast number of studies into the heterogeneity of genome organization both within and between cell types to proceed. However, several improvements could enhance its utility. Notably, the current model exclusively predicts chromatin conformations in 1.28-Mb regions at 20-kb resolution, the latter restriction primarily stemming from our decision to maximize resolution within the constraints imposed by the available Dip-C data. However, higher-resolution single-cell datasets are becoming available, such as those at 5-kb resolution (50), and we anticipate that ChromoGen will require no modifications to perform well after training on these improved datasets. Similarly, we anticipate that ChromoGen can be directly applied to longer genomic regions if using a lower resolution, e.g.
For decades, end users and systems designers have valued radar technology for its reliability. Especially in adverse weather conditions in which sensors based on other modalities are apt to fail, radar is a dependable technique offering broad application potential.
As a result of this robustness and widespread applicability, radar today is established as a standard sensing system in several high-growth technology sectors. The automotive industry, for example, has been a key driver of radar sensor miniaturization and overall performance improvements. The commercialization of radar for passenger vehicles predates the turn of the century, and radar sensors are also now commonly deployed in advanced driver-assistance systems, including for adaptive cruise control, autonomous emergency braking, and blind-spot assist.