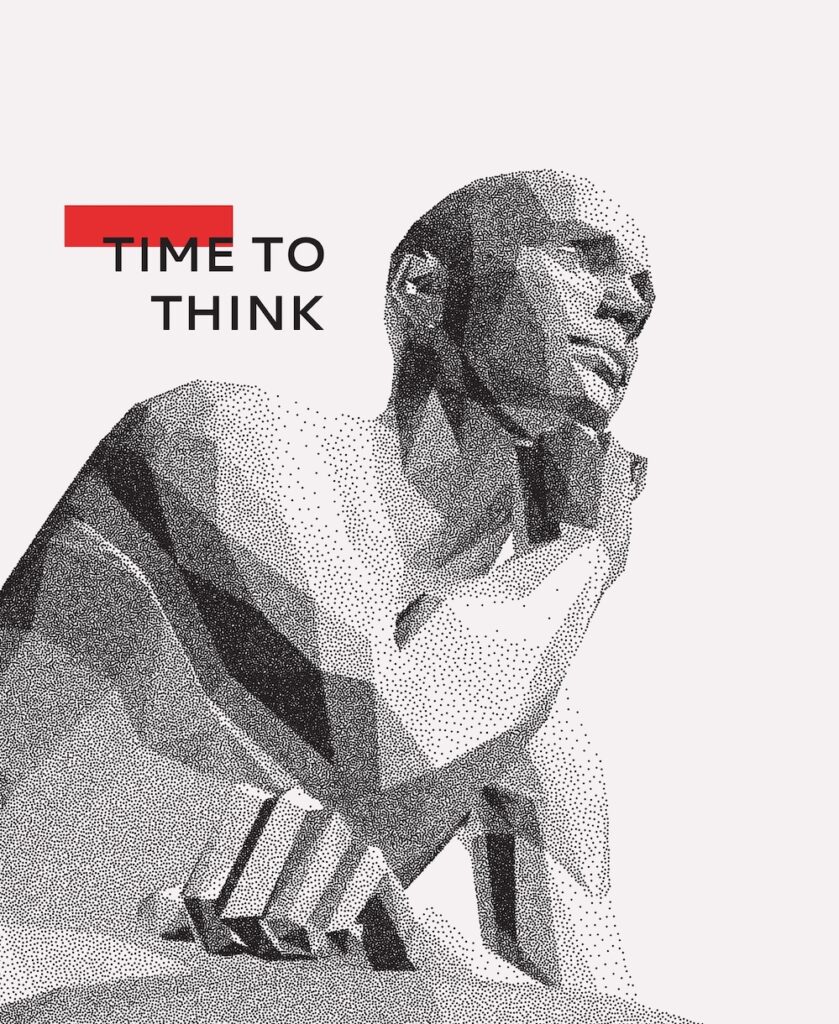
Elon Musk doesn’t follow the same standards that most entrepreneurs do. He’s different, he likes to be different!
And when you’re different, and you’re not afraid to be, it’s okay to test a cigar (or should I say ‘joint’?) of tobacco mixed with marijuana, on Joe Rogan’s famous podcast. But if you look closely, Elon was just nice (polite) and followed Rogan’s elaborate script. Before trying it, Musk even asked him if it was legal.
Then all those facial expressions of Musk, which photojournalists love to catch, go viral as if he’s there promoting some soft drug or passing abroad that his office at Tesla (or SpaceX) is enveloped in a large cloud of smoke.
Quite the opposite. The expressions themselves spoke for themselves, as if to say, “This is nothing special, Joe. Why do you waste my time with these scenes”? Musk even claimed that weed is not good for productivity at all, but it has nothing against (as I do, by the way).