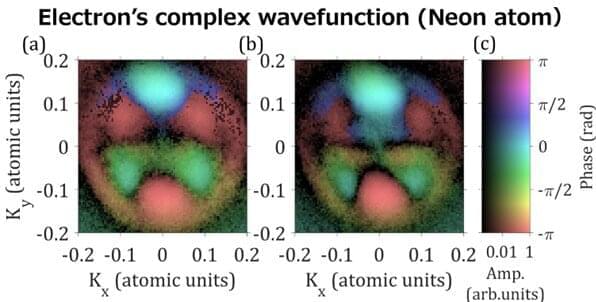
The early 20th century saw the advent of quantum mechanics to describe the properties of small particles, such as electrons or atoms. Schrödinger’s equation in quantum mechanics can successfully predict the electronic structure of atoms or molecules. However, the “duality” of matter, referring to the dual “particle” and “wave” nature of electrons, remained a controversial issue. Physicists use a complex wavefunction to represent the wave nature of an electron.
“Complex” numbers are those that have both “real” and “imaginary” parts—the ratio of which is referred to as the “phase.” However, all directly measurable quantities must be “real”. This leads to the following challenge: when the electron hits a detector, the “complex” phase information of the wavefunction disappears, leaving only the square of the amplitude of the wavefunction (a “real” value) to be recorded. This means that electrons are detected only as particles, which makes it difficult to explain their dual properties in atoms.
The ensuing century witnessed a new, evolving era of physics, namely, attosecond physics. The attosecond is a very short time scale, a billionth of a billionth of a second. “Attosecond physics opens a way to measure the phase of electrons. Achieving attosecond time-resolution, electron dynamics can be observed while freezing molecular motion,” explains Professor Hiromichi Niikura from the Department of Applied Physics, Waseda University, Japan, who, along with Professor D. M. Villeneuve—a principal research scientist at the Joint Attosecond Science Laboratory, National Research Council, and adjunct professor at University of Ottawa—pioneered the field of attosecond physics.