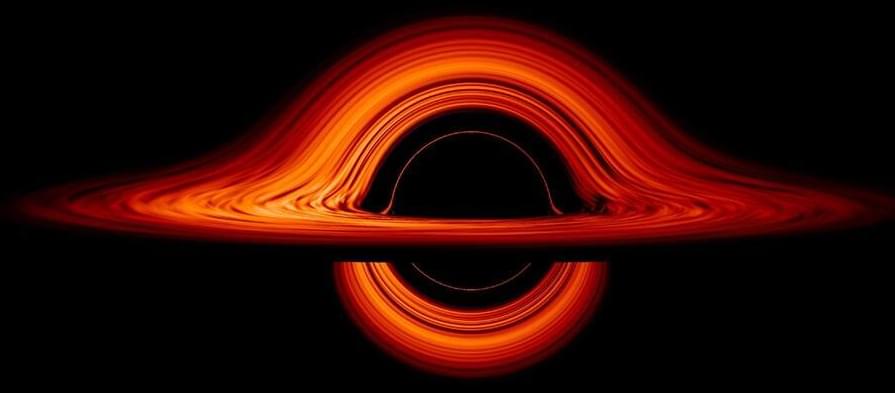
Within a year, Karl Schwarzschild, who was “a lieutenant in the German army, by conscription, but a theoretical astronomer by profession,” as Mann puts it, heard of Einstein’s theory. He was the first person to work out a solution to Einstein’s equations, which showed that a singularity could form–and nothing, once it got too close, could move fast enough to escape a singularity’s pull.
Then, in 1939, physicists Rober Oppenheimer (of Manhattan Project fame, or infamy) and Hartland Snyder tried to find out whether a star could create Schwarzschild’s impossible-sounding object. They reasoned that given a big enough sphere of dust, gravity would cause the mass to collapse and form a singularity, which they showed with their calculations. But once World War II broke out, progress in this field stalled until the late 1950s, when people started trying to test Einstein’s theories again.
Physicist John Wheeler, thinking about the implications of a black hole, asked one of his grad students, Jacob Bekenstein, a question that stumped scientists in the late 1950s. As Mann paraphrased it: “What happens if you pour hot tea into a black hole?”