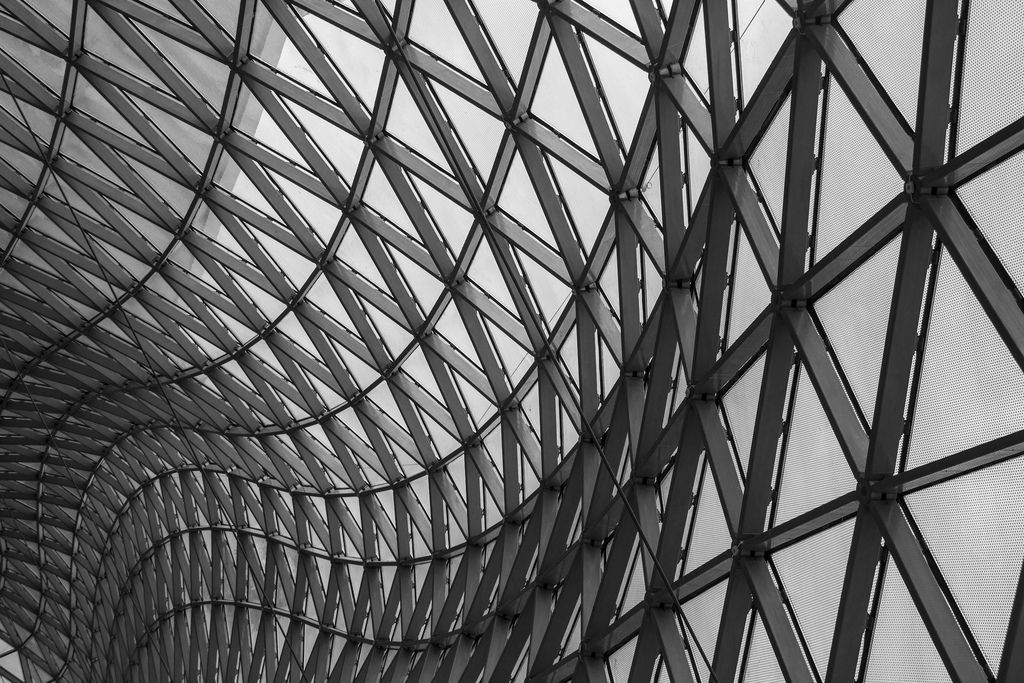
Intel has planted some solid stakes in the ground for the future of deep learning over the last month with its acquisition of deep learning chip startup, Nervana Systems, and most recently, mobile and embedded machine learning company, Movidius.
These new pieces will snap into Intel’s still-forming puzzle for capturing the supposed billion-plus dollar market ahead for deep learning, which is complemented by its own Knights Mill effort and software optimization work on machine learning codes and tooling. At the same time, just down the coast, Nvidia is firming up the market for its own GPU training and inference chips as well as its own hardware outfitted with the latest Pascal GPUs and requisite deep learning libraries.
While Intel’s efforts have garnered significant headlines recently with that surprising pair of acquisitions, a move which is pushing Nvidia harder to demonstrate how GPU acceleration (thus far the dominant compute engine for model training), they still have some work to do to capture mindshare for this emerging market. Further complicating this is the fact that the last two years have brought a number of newcomers to the field—deep learning chip upstarts touting the idea that general purpose architectures (including GPUs) cannot compare to a low precision, fixed point, specialized approach. In fact, we could be moving into a “Cambrian explosion” for computer architecture–one that is brought about by the new requirements of deep learning. Assuming, of course, there are really enough applications and users in a short enough window that the chip startups don’t fall over waiting for their big bang.
Read more