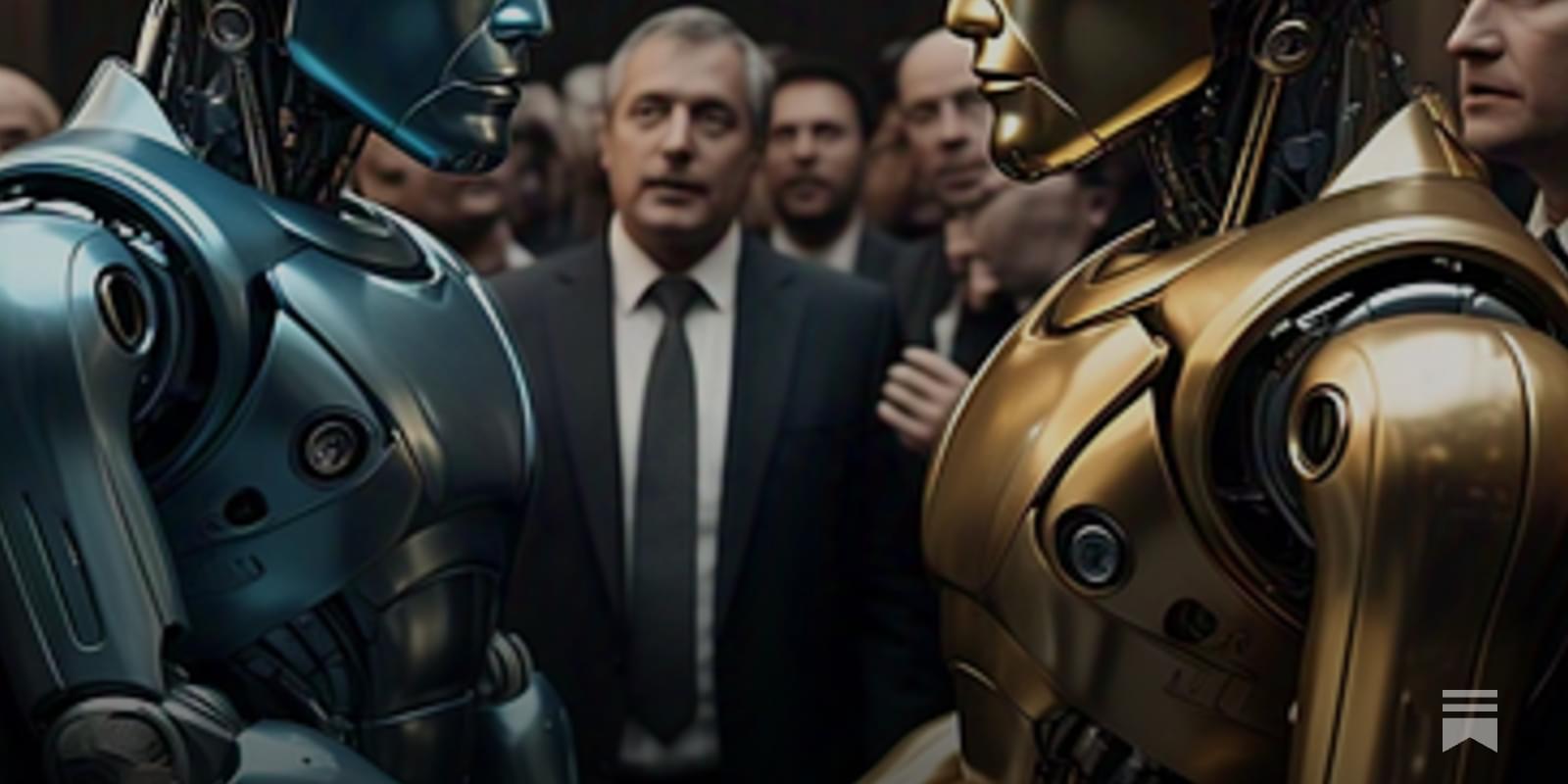
In today’s AI news, CoreWeave has acquired AI developer platform Weights & Biases. According to The Information, CoreWeave spent $1.7 Billion on the transaction. Weights & Biases was valued at $1.25 Billion in 2023. The acquisition extends CoreWeave’s purpose-built cloud platform by enabling an end-to-end experience for customers, enhancing functionality for the world’s leading AI labs and enterprises to build, tune and deploy AI applications.
S Turing Award — often called the Nobel Prize of computer science — is going to Andrew Barto and Richard Sutton, the pioneers of a key approach that underlies much of today Then, a team of researchers at Zoom has developed a breakthrough technique that could dramatically reduce the cost and computational resources needed for AI systems to tackle complex reasoning problems, Chain of draft (CoD), enables large language models (LLMs) to solve problems with minimal words — using as little as 7.6% of the text required by current methods while maintaining or even improving accuracy.
And soon, all businesses will be able to use Meta’s AI to power live, 24/7 customer service that can interact with customers on behalf of businesses on Instagram, Facebook, and WhatsApp. Meta announced advancements in business AI—including the customer service AI agent that will make purchases and can respond to voice prompts from a user.
In videos, say goodbye to manual contract processing! IBM’s Brandon Swink explores how Generative AI, ECM, and Orchestration Hubs streamline document management and improve efficiency. Discover how these technologies transform your approach to complex documents.
And, in this episode of Top of Mind, Gartner Distinguished VP Analyst Daryl Plummer explores the emerging world of guardian agents — AI designed to monitor other AI. Learn how guardian agents will become critical for organizations deploying AI agents for quality control, system observation and security from rogue AI behavior.
Then, how far are we from a true one-person unicorn and what does this mean for the future of employment and capital? Panelists Benjamine Liu, Kanjun Qiu, Dan Murphy, Mitchell Green, Sarah Franklin, and Richard Socher discuss this engaging topic during the recent World Economic Forum in Davos.
S Julia Boorstin sitting down Clara Shih, Meta Thats all for today, but AI is moving fast — like, comment, follow, and subscribe for more Neural News!
Continue reading “CoreWeave Acquires AI Developer Platform Weights & Biases For Reported
In today’s AI news, CoreWeave has acquired AI developer platform Weights & Biases. According to The Information, CoreWeave spent $1.7 Billion on the transaction. Weights & Biases was valued at $1.25 Billion in 2023. The acquisition extends CoreWeave’s purpose-built cloud platform by enabling an end-to-end experience for customers, enhancing functionality for the world’s leading AI labs and enterprises to build, tune and deploy AI applications.
S Turing Award — often called the Nobel Prize of computer science — is going to Andrew Barto and Richard Sutton, the pioneers of a key approach that underlies much of today Then, a team of researchers at Zoom has developed a breakthrough technique that could dramatically reduce the cost and computational resources needed for AI systems to tackle complex reasoning problems, Chain of draft (CoD), enables large language models (LLMs) to solve problems with minimal words — using as little as 7.6% of the text required by current methods while maintaining or even improving accuracy.
And soon, all businesses will be able to use Meta’s AI to power live, 24/7 customer service that can interact with customers on behalf of businesses on Instagram, Facebook, and WhatsApp. Meta announced advancements in business AI—including the customer service AI agent that will make purchases and can respond to voice prompts from a user.
In videos, say goodbye to manual contract processing! IBM’s Brandon Swink explores how Generative AI, ECM, and Orchestration Hubs streamline document management and improve efficiency. Discover how these technologies transform your approach to complex documents.
And, in this episode of Top of Mind, Gartner Distinguished VP Analyst Daryl Plummer explores the emerging world of guardian agents — AI designed to monitor other AI. Learn how guardian agents will become critical for organizations deploying AI agents for quality control, system observation and security from rogue AI behavior.
Then, how far are we from a true one-person unicorn and what does this mean for the future of employment and capital? Panelists Benjamine Liu, Kanjun Qiu, Dan Murphy, Mitchell Green, Sarah Franklin, and Richard Socher discuss this engaging topic during the recent World Economic Forum in Davos.
S Julia Boorstin sitting down Clara Shih, Meta Thats all for today, but AI is moving fast — like, comment, follow, and subscribe for more Neural News!.7 Billion” | >