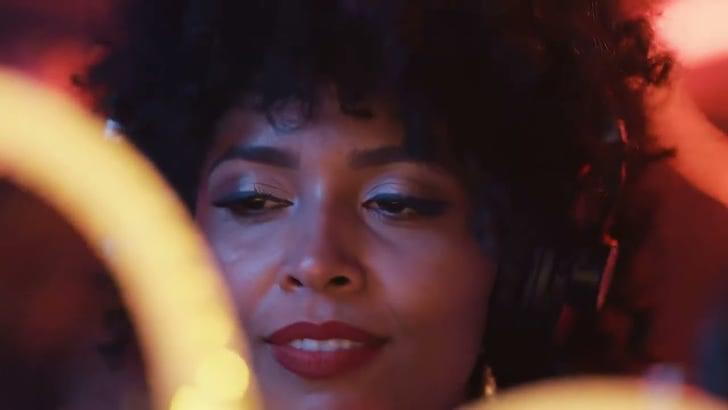
The notion of entropy grew out of an attempt at perfecting machinery during the industrial revolution. A 28-year-old French military engineer named Sadi Carnot set out to calculate the ultimate efficiency of the steam-powered engine. In 1824, he published a 118-page book(opens a new tab) titled Reflections on the Motive Power of Fire, which he sold on the banks of the Seine for 3 francs. Carnot’s book was largely disregarded by the scientific community, and he died several years later of cholera. His body was burned, as were many of his papers. But some copies of his book survived, and in them lay the embers of a new science of thermodynamics — the motive power of fire.
Carnot realized that the steam engine is, at its core, a machine that exploits the tendency for heat to flow from hot objects to cold ones. He drew up the most efficient engine conceivable, instituting a bound on the fraction of heat that can be converted to work, a result now known as Carnot’s theorem. His most consequential statement comes as a caveat on the last page of the book: “We should not expect ever to utilize in practice all the motive power of combustibles.” Some energy will always be dissipated through friction, vibration, or another unwanted form of motion. Perfection is unattainable.
Reading through Carnot’s book a few decades later, in 1865, the German physicist Rudolf Clausius coined a term for the proportion of energy that’s locked up in futility. He called it “entropy,” after the Greek word for transformation. He then laid out what became known as the second law of thermodynamics: “The entropy of the universe tends to a maximum.”
Physicists of the era erroneously believed that heat was a fluid (called “caloric”). Over the following decades, they realized heat was rather a byproduct of individual molecules bumping around. This shift in perspective allowed the Austrian physicist Ludwig Boltzmann to reframe and sharpen the idea of entropy using probabilities.
Boltzmann distinguished the microscopic properties of molecules, such as their individual locations and velocities, from bulk macroscopic properties of a gas like temperature and pressure…