In the age of AI, computer science is no longer the safe major.
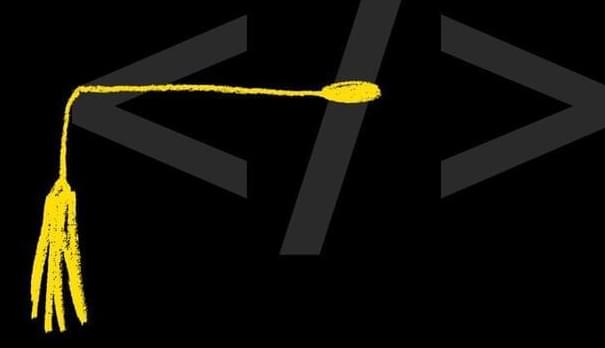
Fascinating 3D electron microscopy study by Parlakgül et al. wherein they compare the subcellular organization of liver tissue from lean and obese mice. The authors found substantial differences in endoplasmic reticulum (ER) organization. These ER differences were shown to directly influence metabolic health in a causal fashion, opening the doors to new ways of treating metabolic dysfunction. #electronmicroscopy #cellbiology #metabolism
Detailed reconstruction using enhanced focused ion beam scanning electron microscopy imaging and deep-learning-based automated segmentation demonstrates that hepatocyte subcellular organelle architecture regulates metabolism.
An extremely important landmark paper by Watson et al. from David Baker’s group. They developed RFdiffusion, a generative AI for protein engineering. With this tool, scientists can generate new protein designs. The software can generate novel monomers, multimers, and symmetric protein cages. It can generate proteins that bind to desired targets as well as enzymes that position active sites at a desired location on the structure. I would say that this technology has the potential to dramatically alter how biology and biotechnology is done. I’ve been waiting for something like this since middle school! So exciting to see protein engineering reaching this point!! #computationalbiology #proteinengineering #syntheticbiology #biotechnology #biochemistry #ai #generativeai
Fine-tuning the RoseTTAFold structure prediction network on protein structure denoising tasks yields a generative model for protein design that achieves outstanding performance on a wide range of protein structure and function design challenges.
This article was written by Grant Aylward, product manager – warehouse robotics, and Lauren Miller, director of autonomy & behavior for Stretch.
We reached a big milestone with Stretch at the end of August—since shipping to customers in January 2023, Stretch robots have moved more than 1 million customer boxes. We designed Stretch to automate the strenuous work of unloading trailers and containers for greater safety and efficiency, and the demand has been tremendous. Stretch takes on the labor of repetitive lifting and lowering of heavy loads, and keeps the flow of goods moving so warehouses can meet demand. Stretch robots are tackling that work every day with customers like DHL and Maersk.
Stretch remains a very early-stage product. Our first prototype Stretch robot powered on in 2019, and we kicked off our first long-term installation at a customer site just this year. So, how did we get to 1 million boxes with such a young robot? As we developed Stretch over the past few years, we worked closely with potential customers to understand warehouse environments and operations and to refine our product to meet their need. As we shifted from Boston Dynamics’ long legacy of R&D work to commercializing robots, we also put certain structures and practices in place to get the robot out of the lab and into the real world. This involved three major efforts: increasing performance, robustness, and reliability of our robot; focusing on safety; and building amazing partner relationships.
Amazon is buying itself and its customers’ priority access to Anthropic’s foundational models.
As Amazon announced in a press release, the online retailer and cloud provider plans to invest up to four billion US dollars in the AI startup Anthropic. Amazon has thus secured an important partner in the field of generative AI. Anthropic is best known for its chatbot Claude, which competes with OpenAI’s ChatGPT.
Anthropic moves to the AWS cloud.
Summary: Neuroscientists have achieved a groundbreaking feat by mapping the early visual system of a parasitic wasp, smaller than a grain of salt.
Utilizing advanced imaging technologies, they reconstructed the entire system at the synaptic level, a first for any animal. Despite its miniature size, the wasp’s brain exhibited immense complexity, with functions and neural circuits paralleling larger brains.
This research not only deepens understanding of neural principles but also holds potential for enhancing artificial intelligence.
India became only the fourth nation ever to land a spacecraft on the Moon earlier this summer. The Chandrayaan-3 mission is still technically underway, but its days may be numbered. After waiting several weeks for the lunar night to end, the Indian Space Research Organisation (ISRO) reports that the mission’s Vikram lander and Pragyan rover remain offline.
Chandrayaan-3 arrived in orbit of the Moon in July, right alongside Russia’s Luna-25 spacecraft. The uncrewed missions were both angling to be the first to touch down in the Moon’s southern polar region, an area where NASA hopes to send astronauts in the coming years. Russia was on course to land first, but a system error caused the vehicle to crash instead. That left India to land at its leisure, which it did on Aug. 23.
According to the Chandrayaan-3 team, they’ve attempted to contact the lander and rover now that the sun is shining again. However, no signals have been received from the surface. It’s possible Vikram (see above) and Pragyan are well and truly dead after several weeks in the frigid night. However, the ISRO hasn’t given up hope. Even if the batteries are empty, the hardware may still be working. Given some time to soak in the rays, the robots could still come back online.
It encourages wearers to take more steps, covering distances more quickly than they could without it.
A wearable exoskeleton can help runners increase their speed by encouraging them to take more steps, allowing them to cover short distances more quickly.
While previous studies have focused on how wearable exoskeletons can help people reduce the energy they expend while running, the new study, published today in Science Robotics, examines how wearable robots can assist runners as they sprint.
AI? VR? The term might just refer to whatever Meta is doing now.
Almost two years ago, Mark Zuckerberg rebranded his company Facebook to Meta — and since then, he has been focused on building the “metaverse,” a three-dimensional virtual reality. But the metaverse has lost some of its luster since 2021. Companies like Disney have closed down their metaverse divisions and deemphasized using the word, while crypto-based startup metaverses have quietly languished or imploded. In 2022, Meta’s Reality Labs division reported an operational loss of $13.7 billion.
But at Meta Connect 2023, Zuckerberg still hasn’t given up on the metaverse — he’s just shifted how he talks about it. He once focused on… More.
Meta launches Quest 3 and continues to focus on the metaverse — even though the market isn’t as positive. Zuckerberg shows a shift in his thinking about the concept.