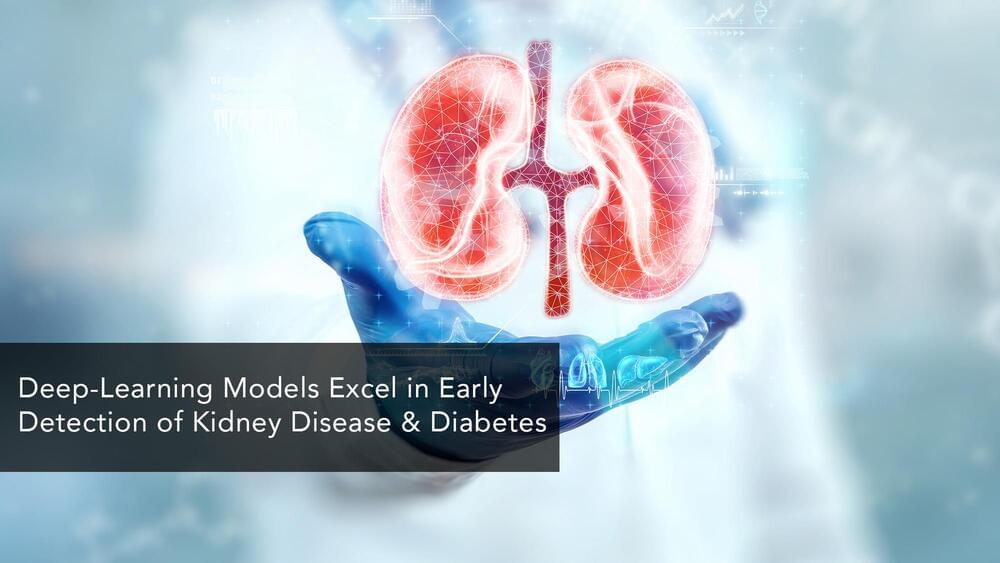
The creation of an artificial intelligence (AI) system that can analyze retinal fundus images to detect chronic kidney disease (CKD) and type 2 diabetes mellitus (T2DM) represents a groundbreaking advancement in medical technology. This AI model, developed using a substantial dataset of retinal images and advanced convolutional neural networks, has demonstrated exceptional accuracy in identifying these conditions. Its capability extends beyond mere detection, as it also shows promise in predicting the progression of these diseases based on retinal imaging and clinical metadata.
A notable innovation of this AI system is its ability to analyze smartphone images. This feature significantly enhances the accessibility of sophisticated diagnostic tools, especially in regions with limited healthcare resources. The AI model paves the way for more widespread and convenient health screenings by enabling ubiquitous smartphone technology for medical imaging. This development is particularly impactful in enhancing healthcare delivery and access, as it brings critical diagnostic capabilities into the hands of more people, even in remote or underserved areas.
The AI’s proficiency in predicting the future development of CKD and T2DM is another aspect of its novelty. This predictive ability is crucial for timely intervention, potentially altering the trajectory of these chronic illnesses. Early detection and management are vital in battling CKD and T2DM, and this AI model’s predictive power could significantly improve patient outcomes.