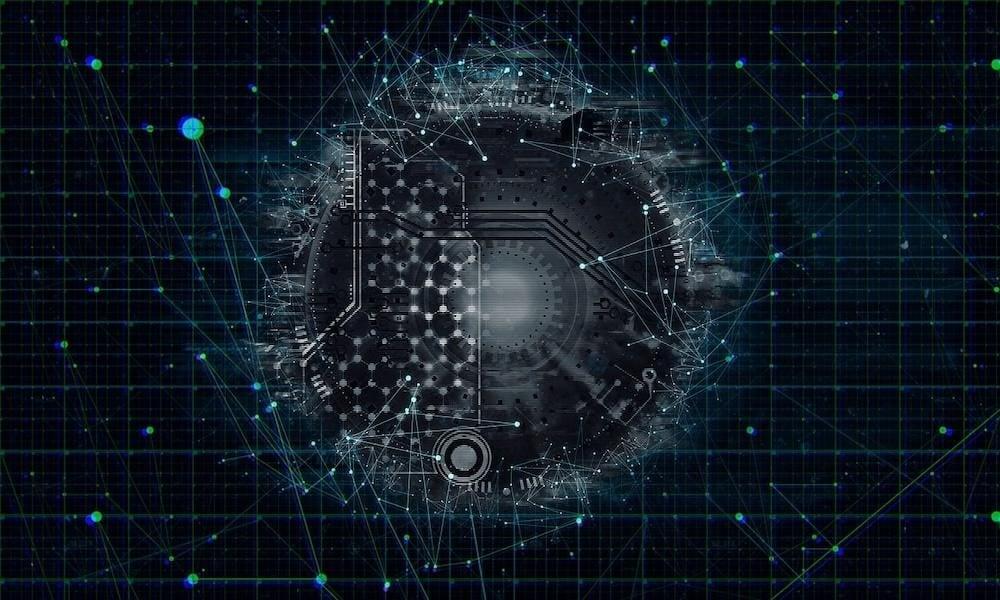
With an emphasis on AI-first strategy and improving Google Cloud databases’ capability to support GenAI applications, Google announced developments in the integration of generative AI with databases.
AWS offers a broad range of services for vector database requirements, including Amazon OpenSearch Service, Amazon Aurora PostgreSQL-Compatible Edition, Amazon RDS for PostgreSQL, Amazon Neptune ML, and Amazon MemoryDB for Redis. AWS emphasizes the operationalization of embedding models, making application development more productive through features like data management, fault tolerance, and critical security features. AWS’s strategy focuses on simplifying the scaling and operationalization of AI-powered applications, providing developers with the tools to innovate and create unique experiences powered by vector search.
Azure takes a similar approach by offering vector database extensions to existing databases. This strategy aims to avoid the extra cost and complexity of moving data to a separate database, keeping vector embeddings and original data together for better data consistency, scale, and performance. Azure Cosmos DB and Azure PostgreSQL Server are positioned as services that support these vector database extensions. Azure’s approach emphasizes the integration of vector search capabilities directly alongside other application data, providing a seamless experience for developers.
Google’s move towards native support for vector storage in existing databases simplifies building enterprise GenAI applications relying on data stored in the cloud. The integration with LangChain is a smart move, enabling developers to instantly take advantage of the new capabilities.