Brighter with Herbert.
Category: robotics/AI – Page 393
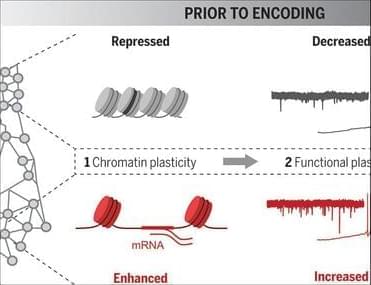
Chromatin plasticity predetermines neuronal eligibility for memory trace formation
It is clear that specific memories are not stored in individual specific neurons.
But the epigenetic state of neurons influences whether they become part of memory…
Memories are encoded by sparse populations of neurons but how such sparsity arises remains largely unknown. We found that a neuron’s eligibility to be recruited into the memory trace depends on its epigenetic state prior to encoding. Principal neurons in the mouse lateral amygdala display intrinsic chromatin plasticity, which when experimentally elevated favors neuronal allocation into the encoding ensemble. Such chromatin plasticity occurred at genomic regions underlying synaptic plasticity and was accompanied by increased neuronal excitability in single neurons in real time. Lastly, optogenetic silencing of the epigenetically altered neurons prevented memory expression, revealing a cell-autonomous relationship between chromatin plasticity and memory trace formation. These results identify the epigenetic state of a neuron as a key factor enabling information encoding.
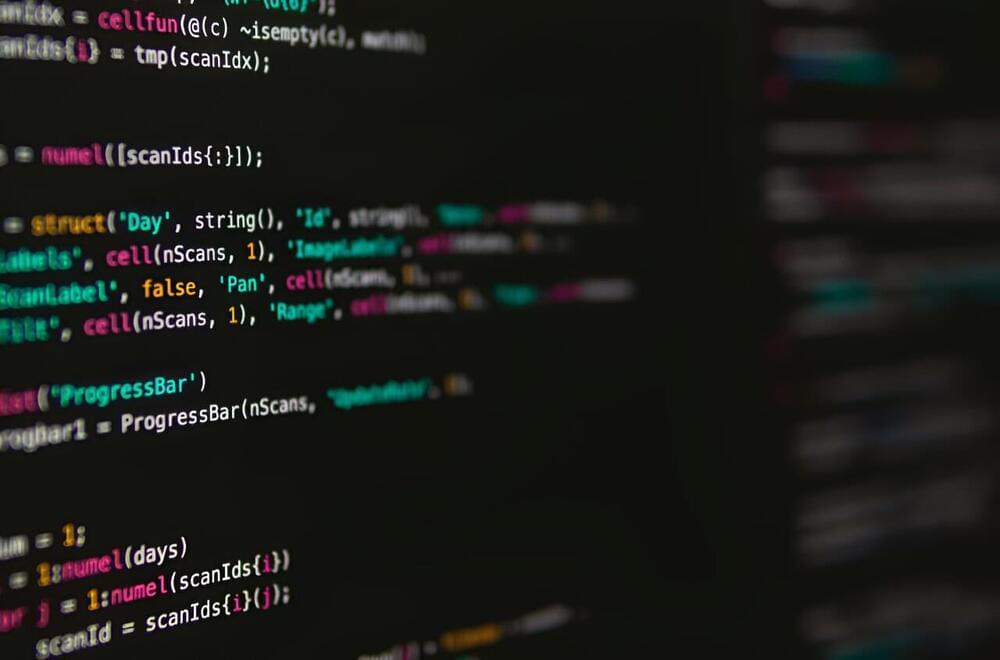
Novel algorithm for discovering anomalies in data outperforms current software
An algorithm developed by Washington State University researchers can better find data anomalies than current anomaly-detection software, including in streaming data.
The work, reported in the Journal of Artificial Intelligence Research, makes fundamental contributions to artificial intelligence (AI) methods that could have applications in many domains that need to quickly find anomalies in large amounts of data, such as in cybersecurity, power grid management, misinformation, and medical diagnostics.
Being able to better find the anomalies would mean being able to more easily discover fraud, disease in a medical setting, or important unusual information, such as an asteroid whose signals overlap with the light from other stars.
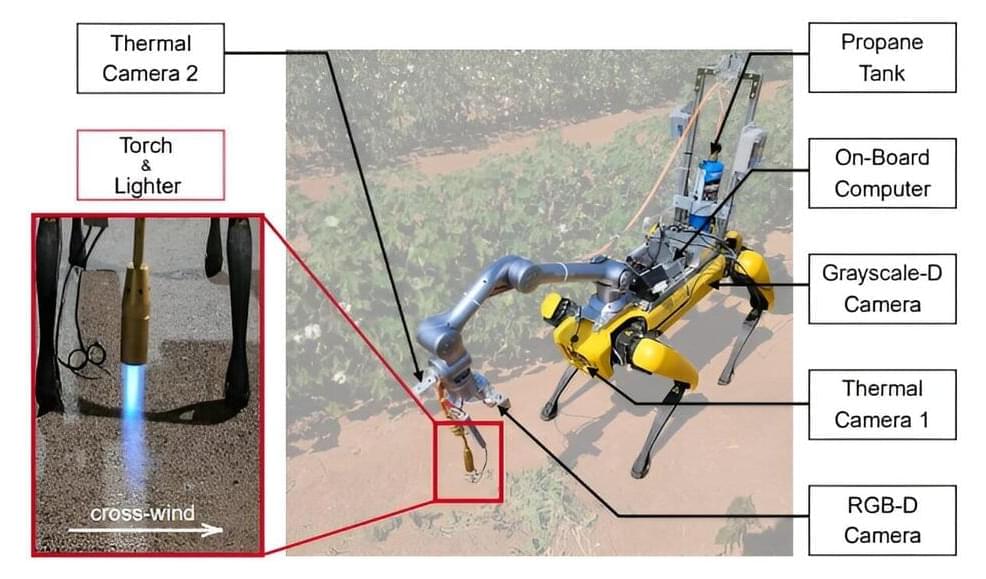
Robot Spot configured to find and stun weeds using a blowtorch
A team of computer scientists and roboticists with members from Texas A&M University in the U.S., and the Mohamed Bin Zayed University of Artificial Intelligence in Abu Dhabi, working with a colleague from Boston Dynamics, has configured a robot made by Boston Dynamics to seek out and stun weeds using a small blowtorch. The team has posted a paper describing their efforts to the arXiv preprint server.
Boston Dynamics, maker of the well-known quadruped Big Dog, has been working on technology to improve both the robot’s agility and processing ability. Its latest quadruped is Spot, a robot with increased agility, highly accurate sensors and a brain that includes AI capabilities. In this new effort, the research team used some of Spot’s abilities to tame weeds growing on cropland.
The researchers trained Spot to recognize weeds among a field of regular crops. They also strapped a small tank filled with propane to its back that is used to fuel a small blow torch held by the robot’s arm. The idea is for Spot to wander around cropland looking for weeds and upon finding them, stun them by blasting their central parts with burning gas. The blowtorch is not used to incinerate the weed, but to heat its core to such an extent that the growth of the weed is stunted for several weeks.
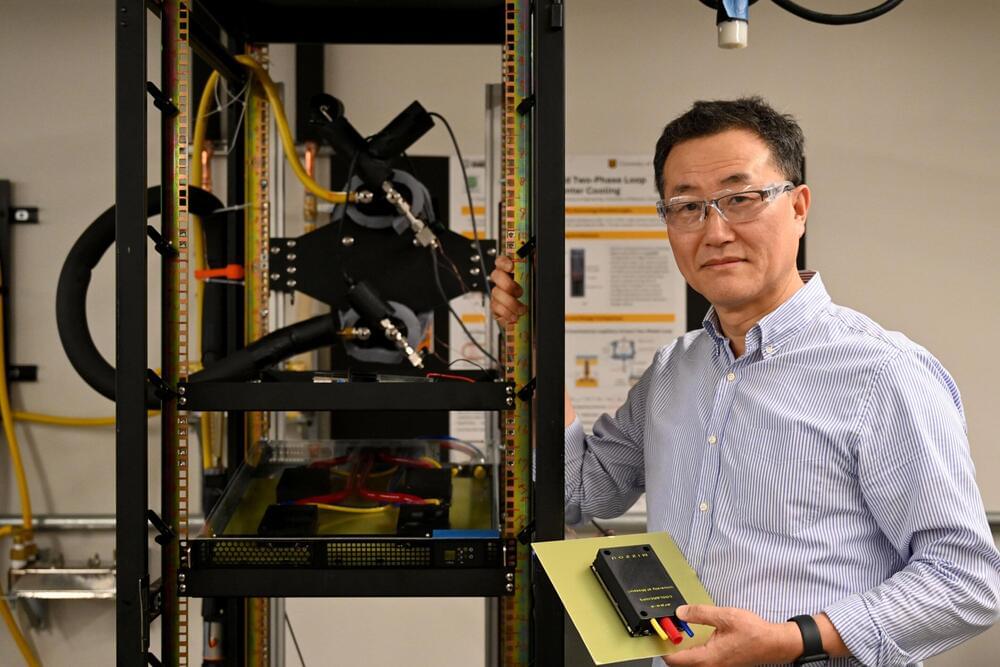
A cool solution: Designing a next-generation cooling system to help data centers become more energy efficient
Artificial intelligence (AI) is hot right now. Also hot: the data centers that power the technology. And keeping those centers cool requires a tremendous amount of energy.
The problem is only going to grow as high-powered AI-based computers and devices become commonplace. That’s why University of Missouri researcher Chanwoo Park is devising a new type of cooling system that promises to dramatically reduce energy demands.
The work is published in the journal Applied Thermal Engineering.
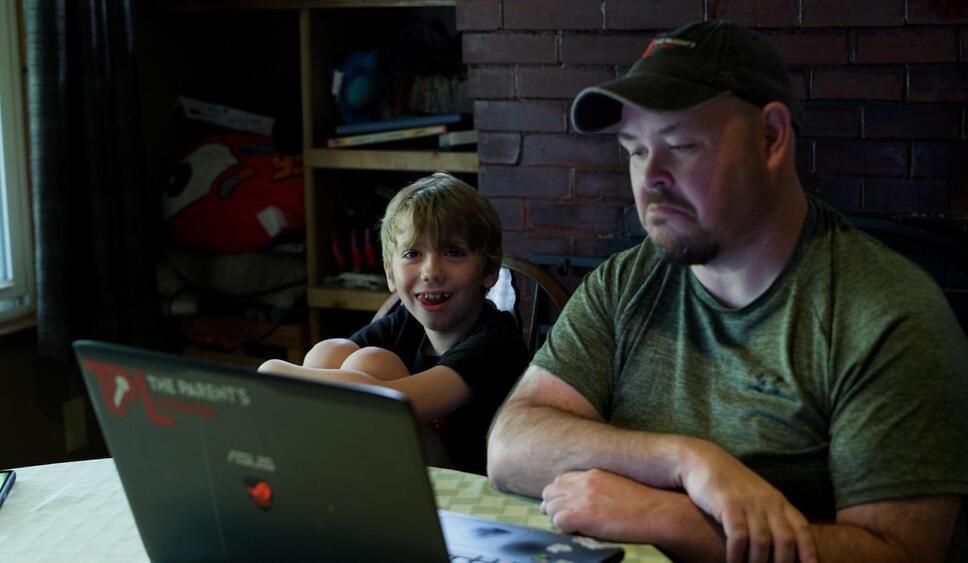
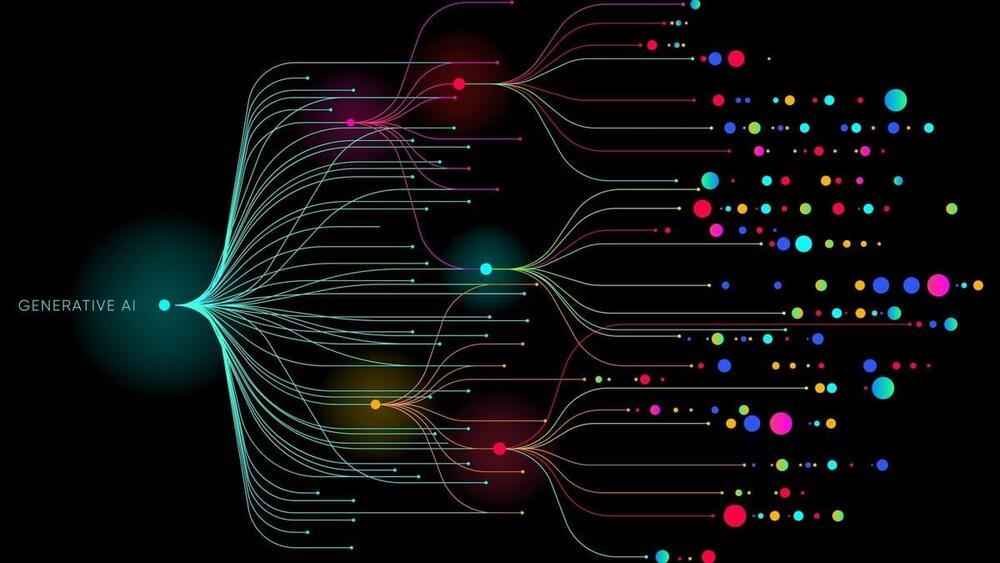
Chinese AI built off open-source code matches American tech in chatbot benchmark tests
These advancements in AI technologies are happening in China despite the stringent measures that the U.S. is placing against its technological ambitions. The bans and embargoes on high-end chips Washington has placed on Beijing meant that Chinese companies have difficulty sourcing Nvidia’s latest, most potent AI processors. While China is hard at work trying to catch up on the hardware side of things, many experts believe it’s still at least a decade away from achieving parity with the United States.
It’s evident, though, that Chinese tech firms can catch up on the software side despite the hardware bans. Nevertheless, the White House wants to change that with a bill in the U.S. Congress that would give the American government more control over limiting the export of American AI code. Some parties are trying to limit the development of open-source AI tech to stop Chinese companies from using it, but doing so might do more harm than good for the U.S.
Clément Delangue, the CEO of Hugging Face, a firm that lets its users share its open-source AI projects, said, “Open-source AI is the foundation of AI development,” referring to how the collaboration of researchers and organizations in the U.S. allowed it to become the world leader in AI, “and it looks like China could do the same thing.”
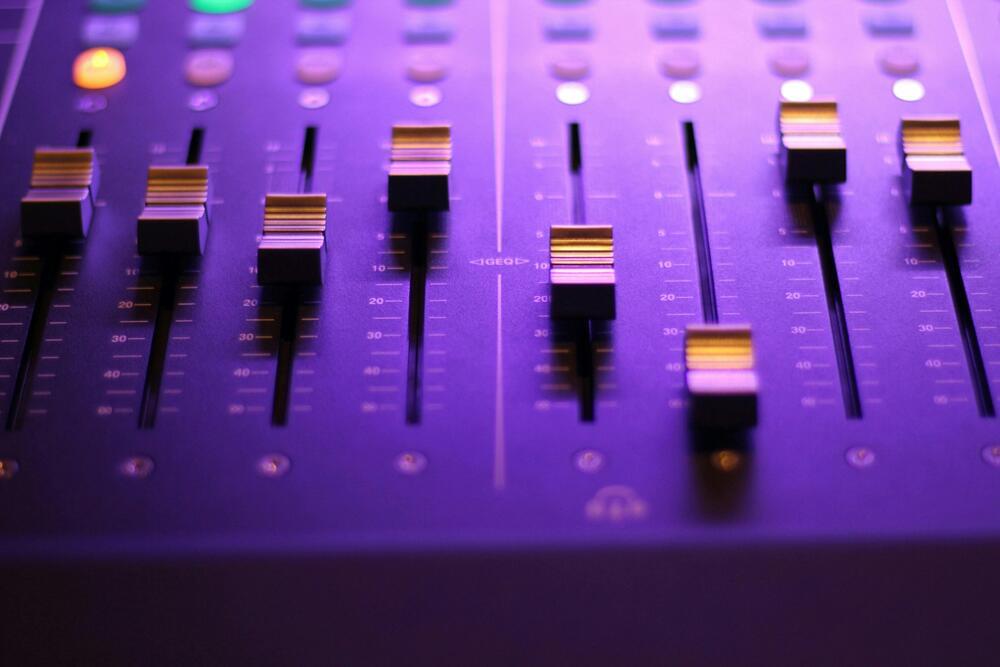
Artificial Intelligence in Sound Design: A Revolution or a Threat?
In this article, Roman Ponomarenko, sound designer and composer with 20+ years of professional experience, explores the transformative potential of AI in sound design and what this means for the future of music.
Artificial intelligence (AI) is already making significant progress in music and sound design. However, will the sophisticated AI of the future eventually replace human professionals in these fields? Navigating such a complex issue proves to be quite challenging, as AI brings forth a mix of exciting opportunities and daunting challenges.
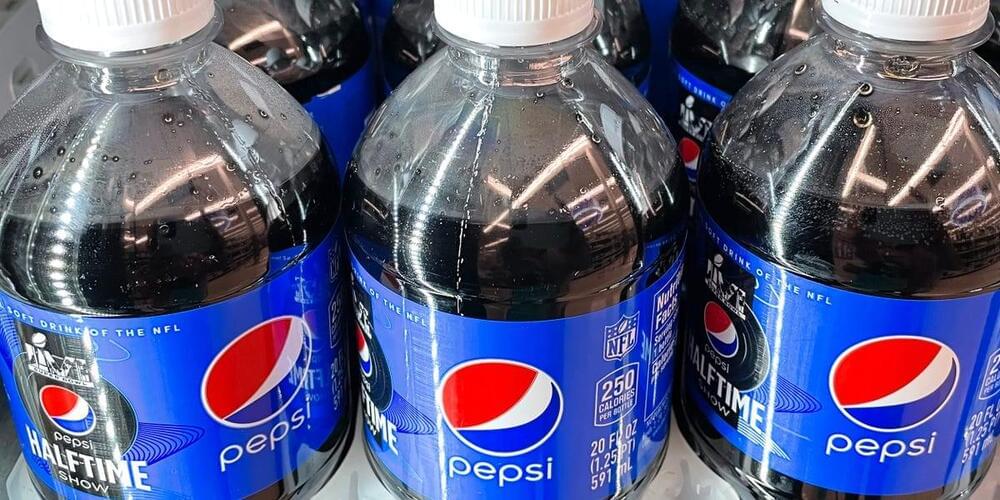
New AI Robot with Human Brain Shocks the World! (They’ve Crossed the Line)
Recent advancements in AI and robotics have led to significant breakthroughs, including a robot with a brain-on-a-chip in China and Skild AI’s development of a universal robot brain for complex tasks. Meanwhile, Zen Technologies in India has introduced Prahasta, a four-legged robot equipped with advanced LIDAR and AI for defense purposes, capable of navigating difficult terrains and carrying heavy loads. These innovations highlight the rapid evolution of robotics technology, blending artificial intelligence with physical capabilities in unprecedented ways, poised to transform industrial, defense, and healthcare sectors.
#ai #robot