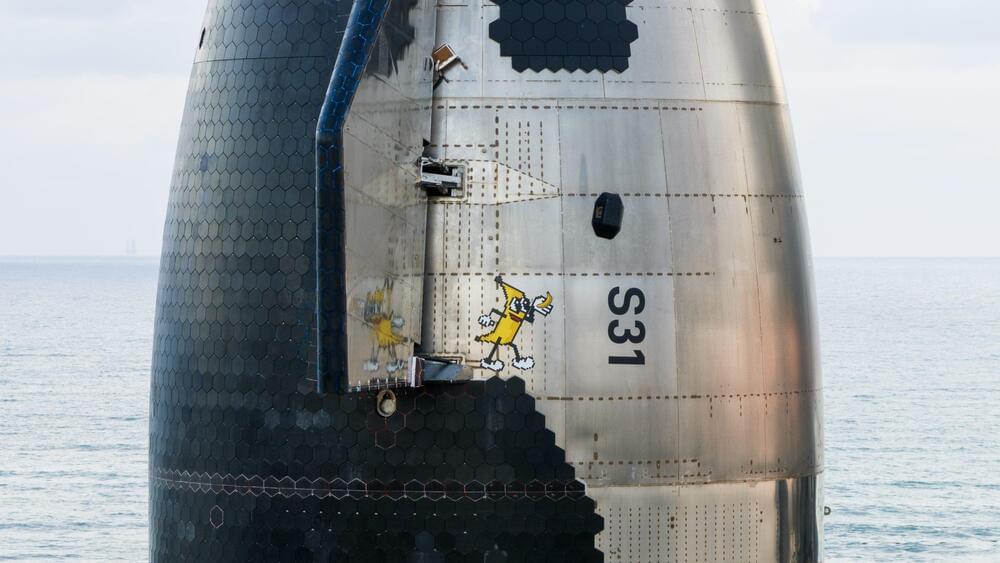
Called the ship’s nosecone, footage from local media spotted this piece being welded by robots at SpaceX’s facilities in Boca Chica, Teas. These facilities are part of a sprawling complex called Starbase, and they include manufacturing, assembly and testing facilities for the world’s largest rockets.
SpaceX has already started operations at its massive Starfactory. Some operations at the plant include inspecting the thousands of heatshield tiles on the nosecone after they are installed. For Starship Flight 7 and beyond, SpaceX will use upgraded heatshield tiles and a new design for the upper stage to improve its reliability during reentry.
Footage from local media in Texas shows workers and robots working on the Starship nosecone for what is presumably a component for a rocket destined for a future flight. SpaceX’s welding robot is clearly visible as it makes small changes to the nosecone, leading to barely visible sparks. Technicians, on the other hand, work on the nosecone with heatshield tiles installed.