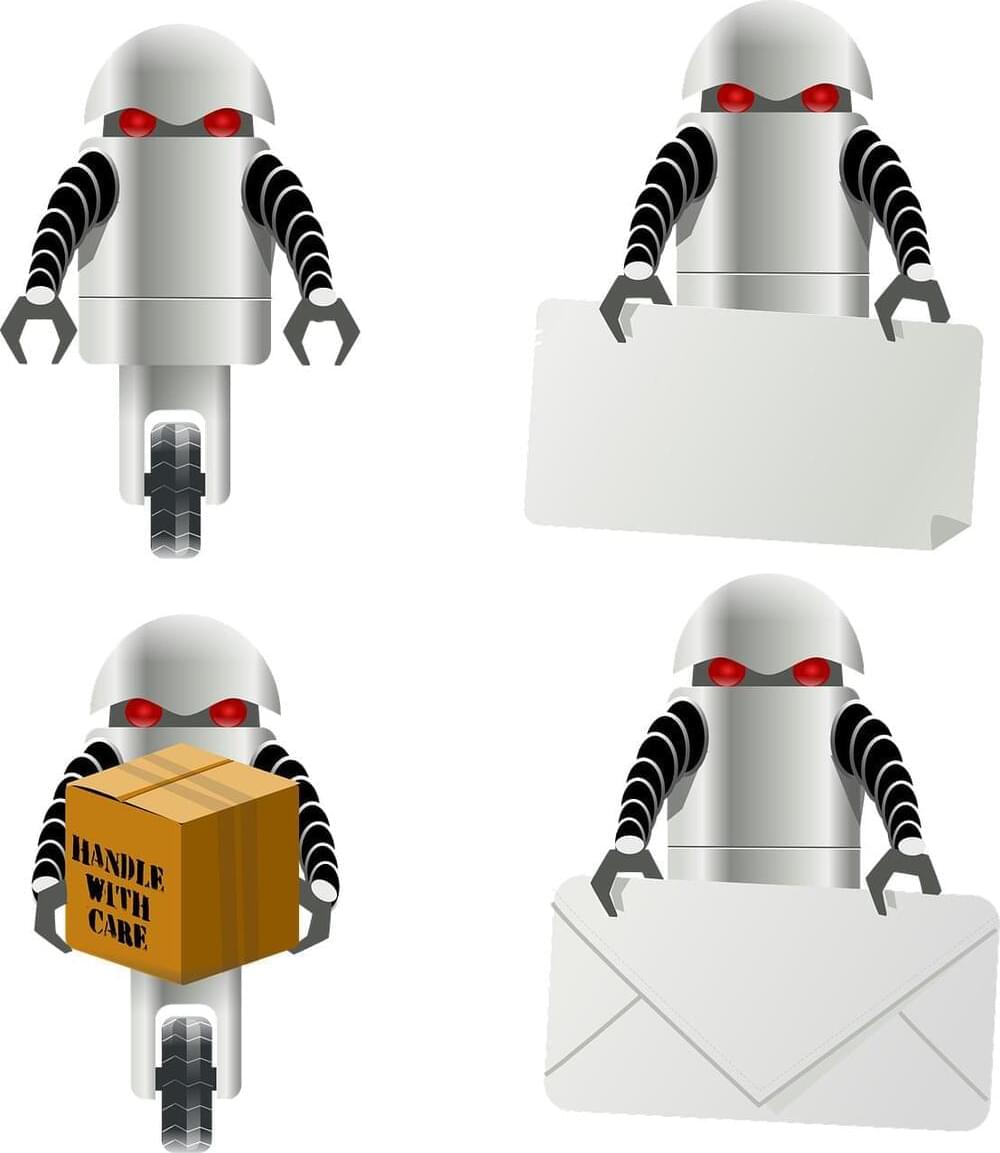
Could food delivery robots with zero carbon emissions influence a customer’s decision to buy food using them instead of robot vehicles that emit carbon into the atmosphere? This is what a recent study published in the International Journal of Hospitality Management hopes to address as a tea of researchers from Washington State University (WSU) investigated how a customer’s knowledge of an automatic delivery robot’s (ADR) environment impact influences their choice regarding which type of robot they want delivering their food. This study holds the potential to help scientists, environmental conservationists, and the public better understand the benefits of eco-friendly delivery robots for both the short and long term.
“Much of the marketing focus has been on the functionality and the convenience of these automatic delivery robots, which is really important, but it would enhance these efforts to promote their green aspects as well,” said Jennifer Han, who is a doctoral student in WSU’s Carson College of Business and lead author of the study.
For the study, the researchers used the Amazon crowdsourcing platform, MTurk, to conduct an online survey comprised of 418 adults who were instructed to watch videos about ADRs followed by a questionnaire regarding the environmental impact and the risk of using ADRs for their food delivery service. In the end, the team discovered a connection between participants who found ADRs were less risky and wanted an eco-friendly ADR compared to participants who thought ADRs were riskier but weren’t concerned about the environmental consequences.