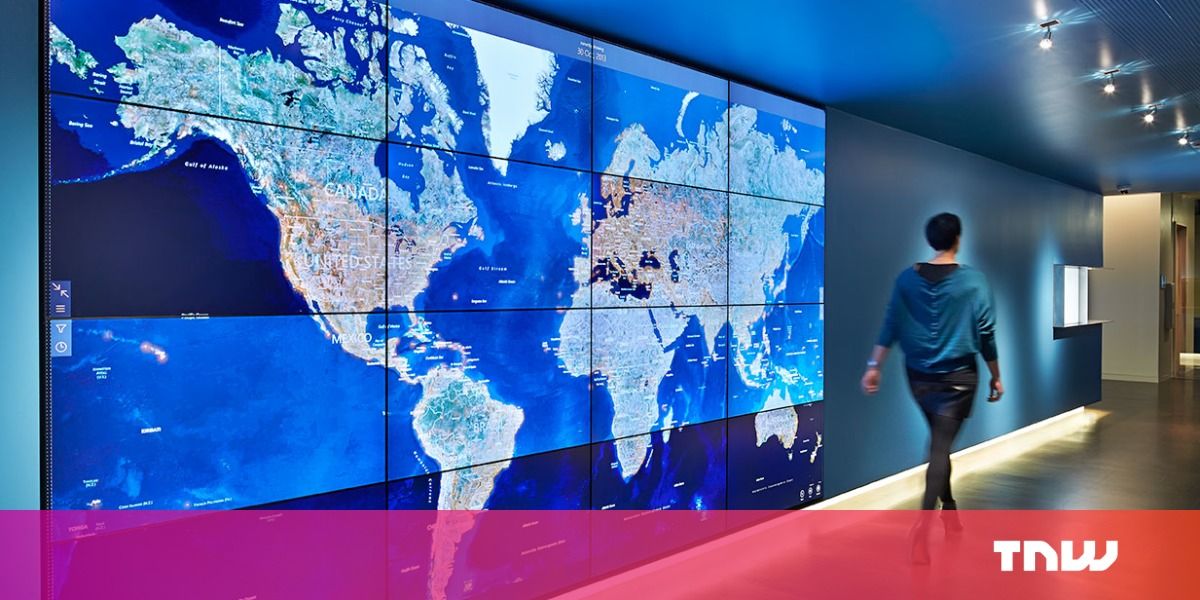
We are now a connected global community where many digital natives cannot remember a time before the iPhone. The rise of smart homes means that we are increasingly attaching our lighting, door locks, cameras, thermostats, and even toasters to our home networks. Managing our home automation through mobile apps or our voice illustrates how far we have evolved over the last few years.
However, in our quest for the cool and convenient, many have not stopped to consider their cybersecurity responsibilities. The device with the weakest security could allow hackers to exploit vulnerabilities on our network and access our home. But this is just the tip of the proverbial iceberg.
Businesses and even governments are starting to face up to the vulnerabilities of everything being online. Sophisticated and disruptive cyberattacks are continuing to increase in complexity and scale across multiple industries. Areas of our critical infrastructure such as energy, nuclear, water, aviation, and critical manufacturing have vulnerabilities that make them a target for cybercriminals and even a state-sponsored attack.
Read more