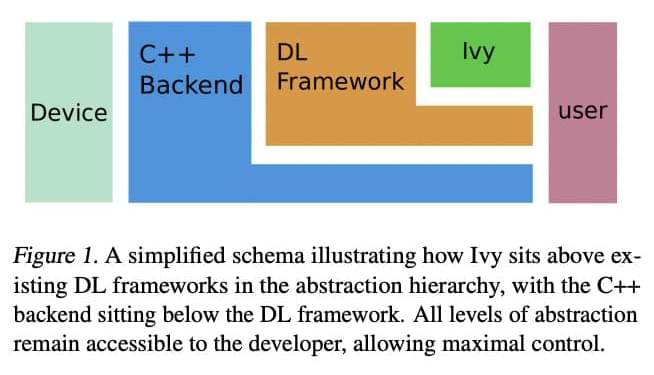
As ML aficionados, we’ve all come across interesting projects on GitHub only to discover that they are not in the framework we want and are familiar with. It can be tedious at times to reimplement the whole codebase in our framework, let alone deal with any errors that may arise throughout the process. It is a tedious chore that no one wants to do. Isn’t it good to have something that doesn’t care what framework you’re using? It will provide you with code in your desired framework, whether it is JAX, PyTorch, MXNet, Numpy, or TensorFlow. This is what IVY is attempting to do by unifying all ML frameworks.
The number of open-source machine learning projects has surged significantly over the past. This is evident by the fast-growing number of Github repositories using the keyword Deep learning. Because of different frameworks, code sharability has been considerably hampered. Aside from that, many frameworks become obsolete in comparison to newer frameworks. For software development where collaboration is vital, this is a significant bottleneck. As newer frameworks come into the scene framework-specific code quickly becomes obsolete, and transferring code across frameworks is akin to reinventing the wheel.
In today’s collaborative environment, it is vital to find a common level of abstraction. The development of IVY began with the language, with Python emerging as the clear choice we go further into Python frameworks, and we see that they all operate on the same fundamental principles. A tensor can be manipulated in a variety of ways, but the core tensor operations are constant across frameworks. As a result, IVY was formed as a basic abstraction layer.