A complete guide to conversational AI in 2023 that help machines understand, process, and respond to human language.
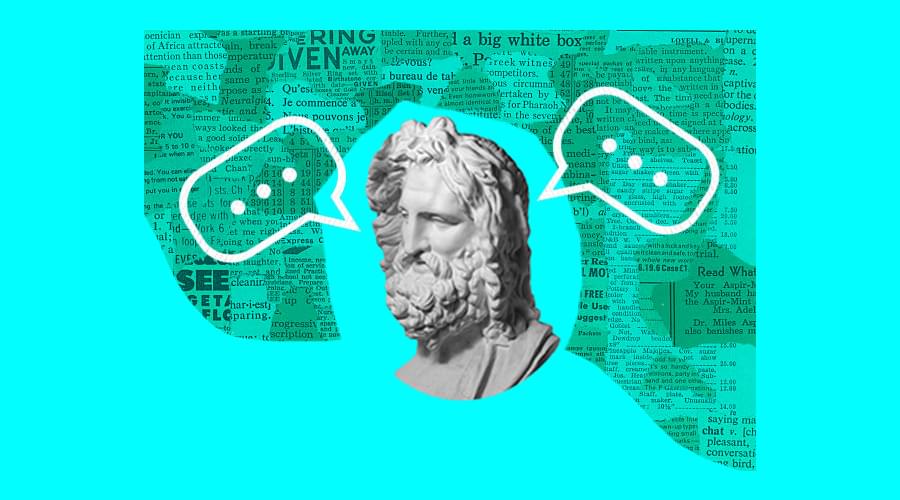
Shares of Alphabet slid during the event, suggesting that investors were hoping for more in light of growing competition from Microsoft.
Google’s event took place just one day after Microsoft hosted its own AI event at its headquarters in Redmond, Washington. Microsoft’s event centered around new AI-powered updates to the company’s Bing search engine and Edge browser. Bing, which is a distant second to Google in search, will now allow users get more conversational responses to questions.
Alphabet shares tumbled Wednesday after a Reuters report said an advertisement for Google’s newly unveiled AI chatbot Bard contained an inaccurate answer to a question aimed at showing the newly unveiled tool’s capability.
Shares of the company fell as much as 8.9% to $98.04 the lowest price since January 31 and barely pared the decline heading into afternoon trade.
Reuters reported an ad published by Google on Twitter featuring a GIF video of Bard — which Google CEO Sundar Pichai on Monday introduced as its “experimental AI service” — offered an incorrect response to a question about NASA’s James Webb Space Telescope.
In a recent study published in the Radiology, researchers performed a single-center, open-label randomized controlled trial (RCT) to investigate whether commercial artificial intelligence (AI)-debased computer-aided design (CAD) system could improve the detection rate of actionable lung nodules on chest radiographs.
Actionable nodules are Lung Imaging Reporting and Data System (Lung-RADS), Category 4 nodules. These might be solid nodules larger than eight mm or subsolid nodules with a solid area spanning over six mm.
Studies have not prospectively explored the impact of AI–based CAD software in real-world settings.
The bar seems to be pretty high. But it’s not like ChatGPT is perfect.
Artificial intelligence technology has drawn massive fanfare from investors this year amid the growing popularity of ChatGPT, which launched in November and has helped its maker, OpenAI, nab a staggering $29 billion valuation. Alphabet’s Bard announcement came one day before Microsoft held a press conference to tout an investment in OpenAI that has helped shares of the Silicon Valley staple surge nearly 20% over the past month. “This is just the first step on the AI front,” Ives told clients in a note after the event, reiterating an outperform rating for shares.
Despite the apparent flub, Bank of America analysts have said they’re bullish on Google’s AI strategy, writing in a note to clients that Google is “well prepared with years of investment” in the technology to capture a significant part of the market, particularly since its search engine has a large distribution advantage, as compared to Microsoft. Nevertheless, the analysts warn safety issues including result inaccuracy or bias, disinformation and the potential use of models for harm are key risks.
“AI is the most profound technology we are working on today,” Alphabet CEO Sundar Pichai said as he announced the new chatbot this week.
Humans are innately able to reason about the behaviors of different physical objects in their surroundings. These physical reasoning skills are incredibly valuable for solving everyday problems, as they can help us to choose more effective actions to achieve specific goals.
Some computer scientists have been trying to replicate these reasoning abilities in artificial intelligence (AI) agents, to improve their performance on specific tasks. So far, however, a reliable approach to train and assess the physical reasoning capabilities of AI algorithms has been lacking.
Cheng Xue, Vimukthini Pinto, Chathura Gamage, and colleagues, a team of researchers at the Australian National University, recently introduced Phy-Q, a new testbed designed to fill this gap in the literature. Their testbed, introduced in a paper in Nature Machine Intelligence, includes a series of scenarios that specifically assess an AI agent’s physical reasoning capabilities.
With a big assist from artificial intelligence and a heavy dose of human touch, Tim Cernak’s lab at the University of Michigan has made a discovery that dramatically speeds up the time-consuming chemical process of building molecules that will be tomorrow’s medicines, agrichemicals or materials.
The discovery, published in the Feb. 3 issue of Science, is the culmination of years of chemical synthesis and data science research by the Cernak Lab in the College of Pharmacy and Department of Chemistry.
The goal of the research was to identify key reactions in the synthesis of a molecule, ultimately reducing the process to as few steps as possible. In the end, Cernak and his team achieved the synthesis of a complex alkaloid found in nature in just three steps. Previous syntheses had taken between seven and 26 steps.
Seminar summary: https://foresight.org/summary/bioelectric-networks-taming-th…-medicine/
Program & apply to join: https://foresight.org/biotech-health-extension-program/
Foresight Biotech & Health Extension Meeting sponsored by 100 Plus Capital.
Michael Levin, Tufts Center for Regenerative and Developmental Biology.
Bioelectric Networks: Taming the Collective Intelligence of Cells for Regenerative Medicine.
Michael Levin, Distinguished Professor in the Biology department and Vannevar Bush Chair, serves as director of the Tufts Center for Regenerative and Developmental Biology. Recent honors include the Scientist of Vision award and the Distinguished Scholar Award. His group’s focus is on understanding the biophysical mechanisms that implement decision-making during complex pattern regulation, and harnessing endogenous bioelectric dynamics toward rational control of growth and form. The lab’s current main directions are:
• Understanding how somatic cells form bioelectrical networks for storing and recalling pattern memories that guide morphogenesis;
• Creating next-generation AI tools for helping scientists understand top-down control of pattern regulation (a new bioinformatics of shape); and.
• Using these insights to enable new capabilities in regenerative medicine and engineering.
Prior to college, Michael Levin worked as a software engineer and independent contractor in the field of scientific computing. He attended Tufts University, interested in artificial intelligence and unconventional computation. To explore the algorithms by which the biological world implemented complex adaptive behavior, he got dual B.S. degrees, in CS and in Biology and then received a PhD from Harvard University. He did post-doctoral training at Harvard Medical School (1996−2000), where he began to uncover a new bioelectric language by which cells coordinate their activity during embryogenesis. His independent laboratory (2000−2007 at Forsyth Institute, Harvard; 2008-present at Tufts University) develops new molecular-genetic and conceptual tools to probe large-scale information processing in regeneration, embryogenesis, and cancer suppression.