Chinese tech experts have introduced a groundbreaking humanoid robot prototype, setting the stage for its integration into homes.
Category: robotics/AI – Page 120
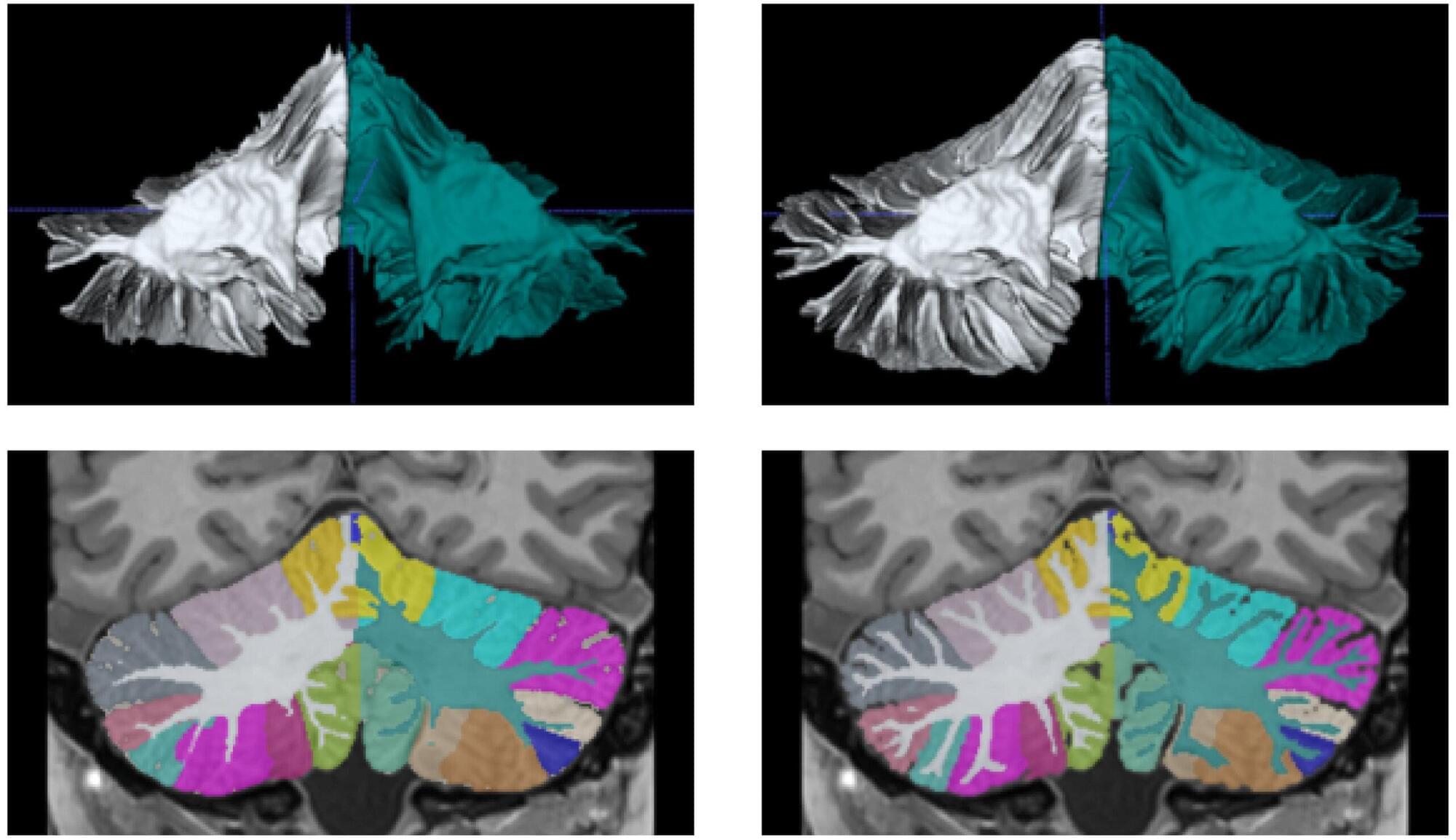
DeepCeres: AI-driven software redefines cerebellum research with detailed imaging
A team of researchers from the Universitat Politècnica de València (UPV) and the French National Center for Scientific Research (CNRS) has developed the world’s most advanced software to study the human cerebellum using high-resolution NMR images.
Called DeepCeres, this software will help in the research and diagnosis of diseases such as ALS, schizophrenia, autism and Alzheimer’s, among others. The work of the Spanish and French researchers has been published in the journal NeuroImage.
Despite its small size compared to the rest of the brain, the cerebellum contains approximately 50% of all brain neurons and plays a fundamental role in cognitive, emotional and motor functions.
Scientists Create Microscopic Algae Robots With Incredible Swimming Abilities
Magnetized algae micro swimmers retain speed and maneuverability, showing promise for targeted drug delivery in confined biological environments. A team of researchers at the Max Planck Institute for Intelligent Systems (MPI-IS) in Stuttgart has developed a biohybrid microswimmer coated with magn
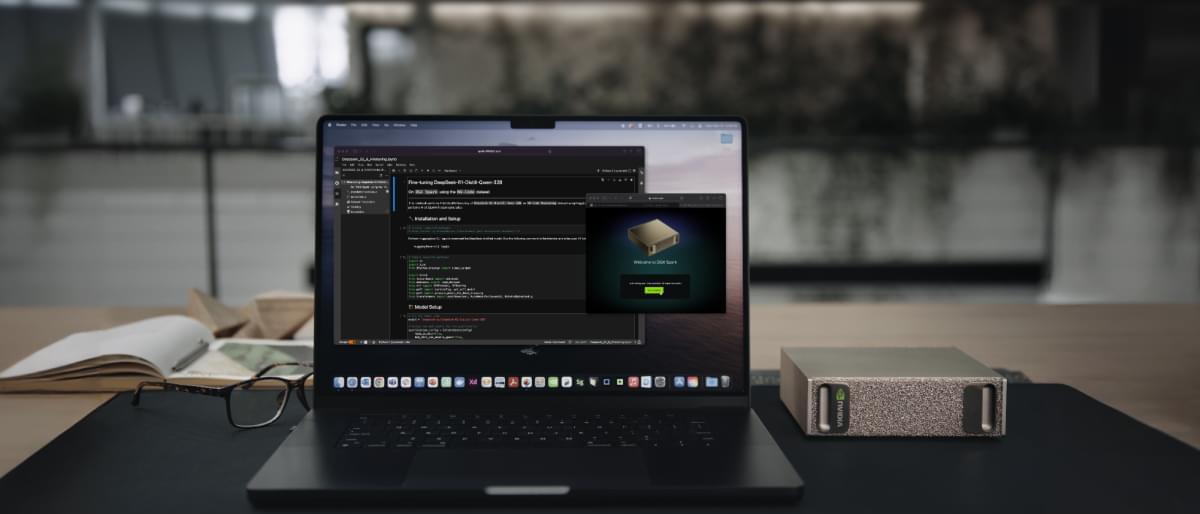
Firefly’s Blue Ghost Captures the First-Ever HD Video of a Stunning Lunar Sunset
Firefly’s Blue Ghost Mission 1 set a new benchmark for commercial lunar exploration, lasting longer than any previous private mission and delivering 10 NASA instruments to the Moon. The mission achieved several firsts, including the deepest robotic thermal probe on another planetary body and the
Boston Dynamics Atlas AI Robot’s New Shocking Capabilities and Unitree G1 Humanoid’s Latest Record
The world-famous Atlas humanoid robot by Boston Dynamics is showing off its new look and capabilities.
According to the Massachusetts-based robotics leader, Atlas achieved its smooth movements and human-like walking gait with reinforcement learning and motion capture. Boston Dynamics released new demo footage as the company shared its progress at Nvidia’s GTC 2025 conference.
The AI-powered humanoid robot became a pop culture icon in the twenty tens. Viral videos showcasing its increasingly agile and human-like capabilities amazed and horrified millions of people. Others worried the AI robot would evolve to resent humanity for its harsh treatment from its creators.
Since becoming fully electric in 2024, Atlas has been more low-key, demonstrating industrial tasks rather than parkour.
Now it’s learning at an accelerated rate and it’s showing off. It has a new look, a more powerful brain, and it’s doing stunts again. But the humanoid robotics market is in a very different place, with dozens of firms, mostly from China and the United States, boasting new capabilities nearly every day.
Can Atlas come out ahead as it competes with Tesla, Figure AI, Agility Robotics and a growing array of Chinese firms racing to mass deploy their humanoids?
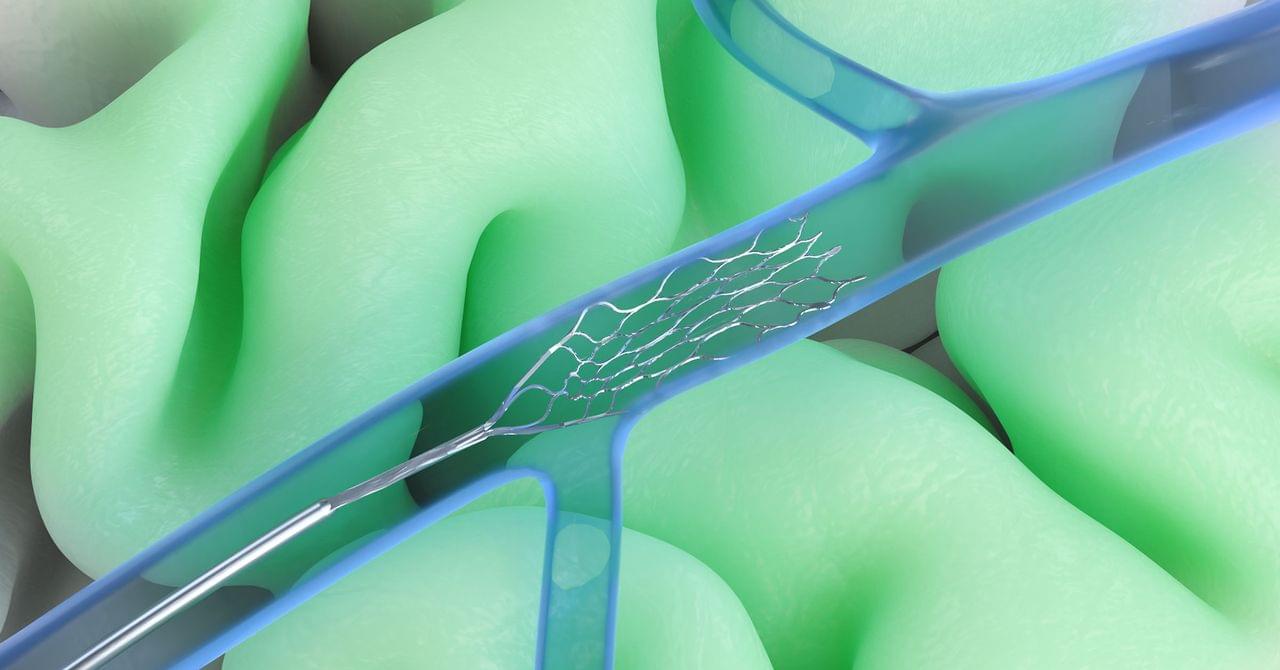
A marine robot that can swim, crawl and glide untethered in the deepest parts of the ocean
A team of mechanical engineers at Beihang University, working with a deep-sea diving specialist from the Chinese Academy of Sciences and a mechanic from Zhejiang University, all in China, have designed, built, and tested a marine robot that can swim, crawl, and glide untethered in the deepest parts of the ocean.
In their paper published in the journal Science Robotics, the group describes the factors that went into their design and how well their robot performed when tested.
Over the past several decades, underwater robots have become very important tools for studying the various parts of the world’s oceans and the creatures that live in them. More recently, it has been noted that most such craft, especially those that are sent to very deep parts of the sea, are cumbersome and not very agile.
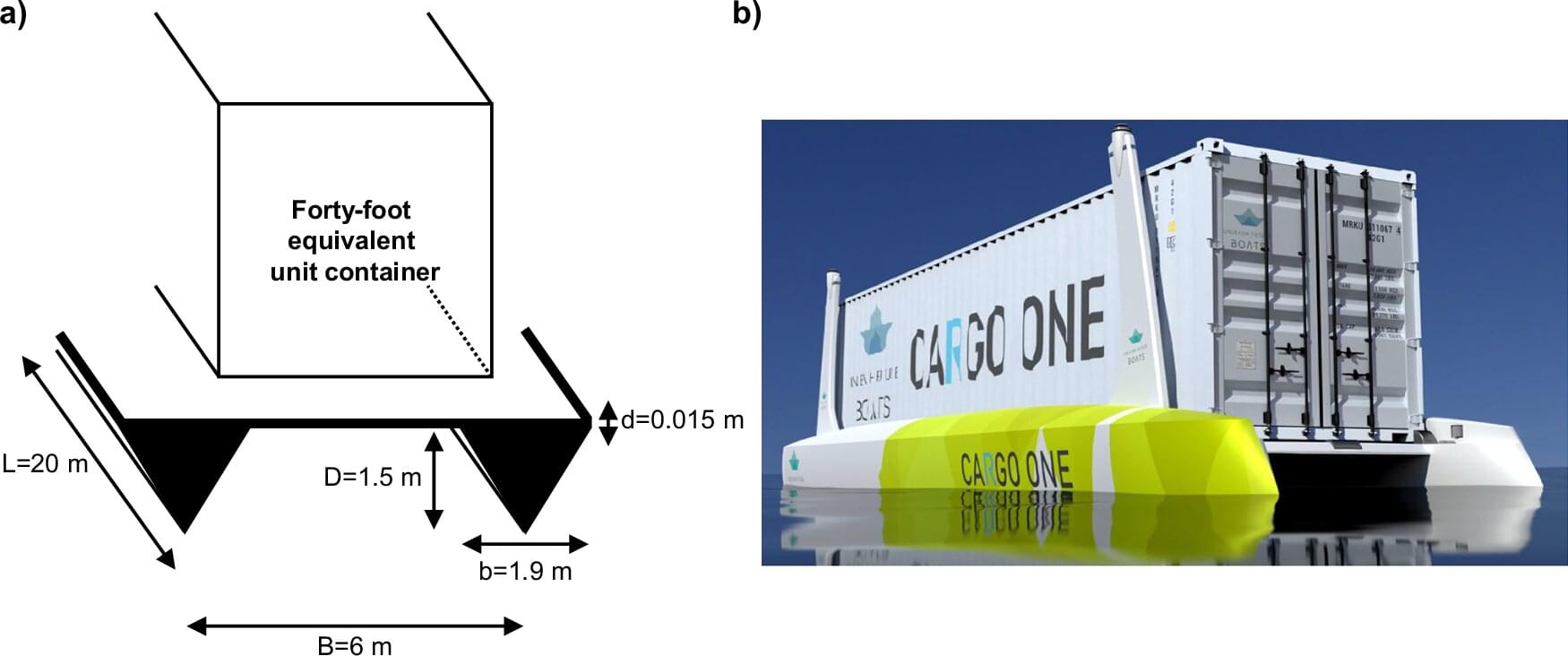
Hydrogen-powered boats offer climate-friendly alternative to road transport
Cargo transport is responsible for an enormous carbon footprint. Between 2010 and 2018, the transport sector generated about 14% of global greenhouse gas emissions. To address this problem, experts are looking for alternative, climate-friendly solutions—not only for road transport, but also for shipping, a sector in which powering cargo ships with batteries has proved especially difficult.
One promising but under-researched solution involves small, autonomous, hydrogen-powered boats that can partially replace long-haul trucking. A research team led by business chemist Prof Stephan von Delft from the University of Münster has now examined this missing link in a new study published in Communications Engineering.
The team has mathematically modeled such a boat for the first time and carried out a life cycle- and cost analysis. “Our calculations show in which scenarios hydrogen-powered boats are not only more sustainable but also more economical compared to established transport solutions,” explains von Delft. “They are therefore relevant for policymakers and industry.”
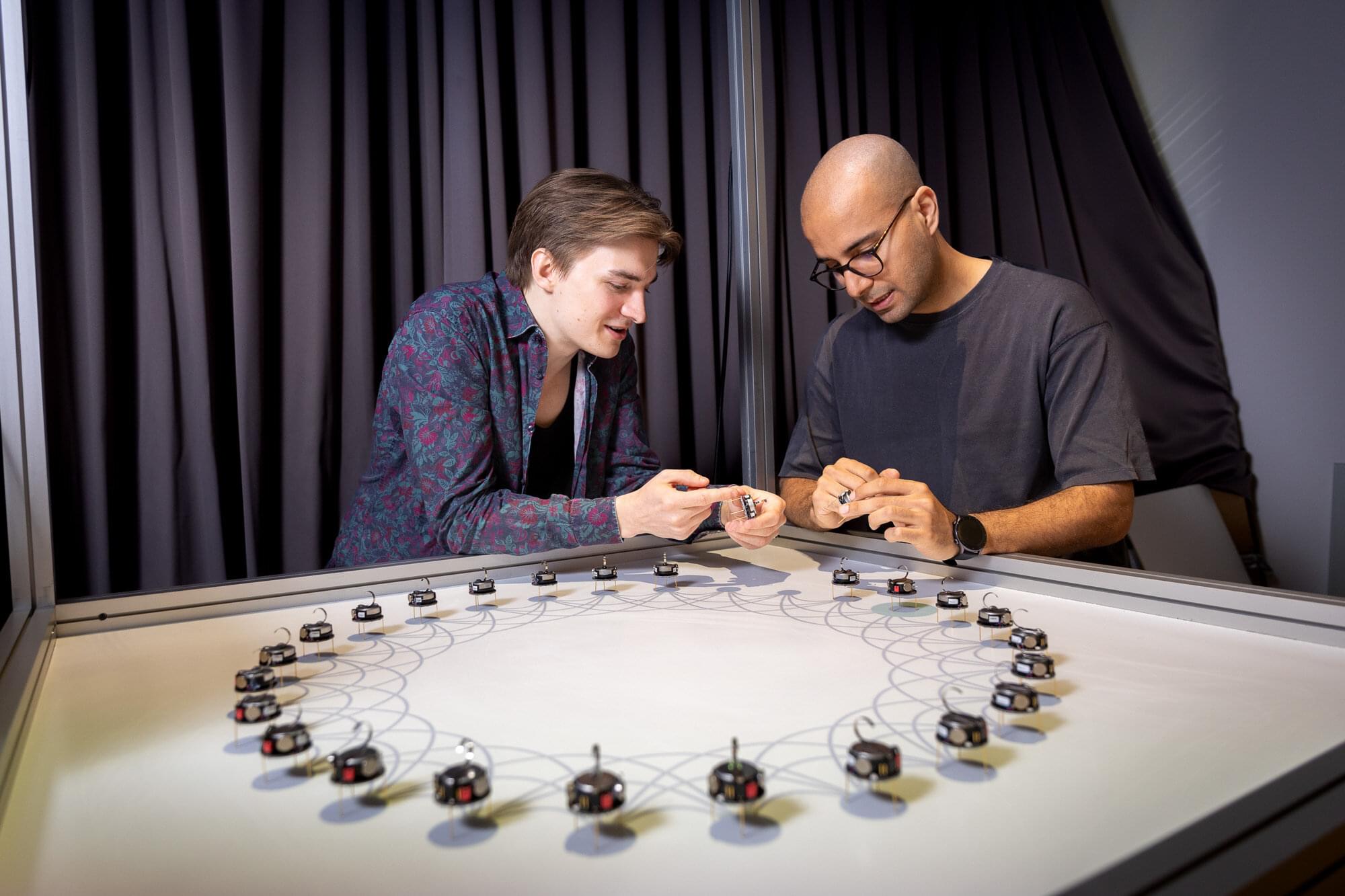
From robot swarms to human societies, good decisions rely on the right mix of perspectives
When groups make decisions—whether it’s humans aligning on a shared idea, robots coordinating tasks, or fish deciding where to swim—not everyone contributes equally. Some individuals have more reliable information, whereas others are more connected and have higher social influence.
A new study by researchers at the Cluster of Excellence Science of Intelligence shows that a combination of uncertainty and heterogeneity plays a crucial role in how groups reach consensus.
The findings, published in Scientific Reports by Vito Mengers, Mohsen Raoufi, Oliver Brock, Heiko Hamann, and Pawel Romanczuk, show that groups make faster and more accurate decisions when individuals factor in not only the opinions of their neighbors but also their confidence about these opinions and how connected those others are within the group.