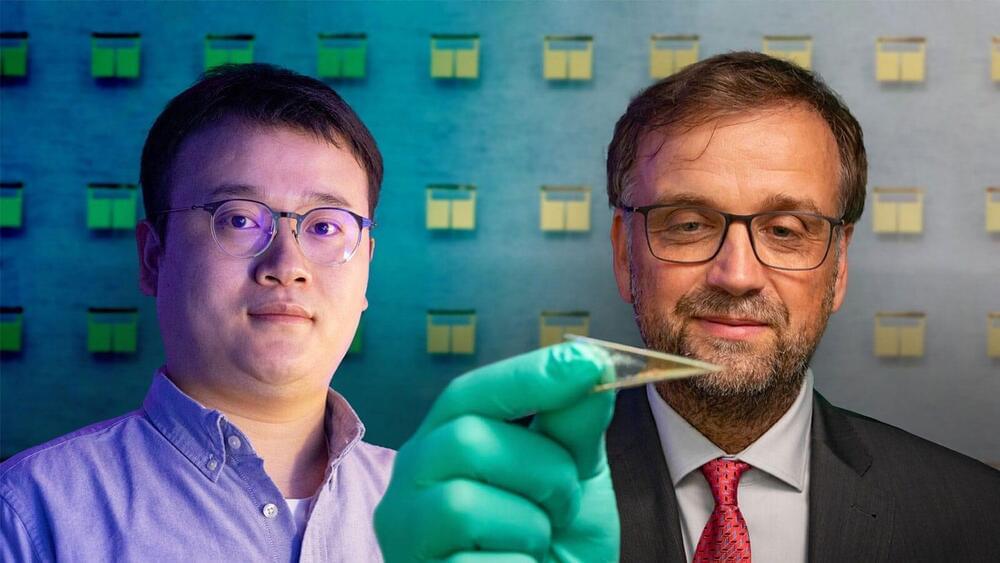
The new microbattery is roughly the size of a gain of dust – less than one square millimeter – and has a minimum energy density of 100 microwatt hours per square centimeter. To achieve this, the team winded up current collectors and electrode strips made of polymeric, metallic, and dielectric materials at the microscale. The researchers used Swiss-roll or micro-origami process.
The layered system with inherent tension is created by consecutively coating thin layers of polymeric, metallic, and dielectric materials onto a wafer surface. The mechanical tension is released by peeling off the thin layers, which then automatically snap back to roll up into a Swiss-Roll architecture to create a self-wound cylinder microbattery. The method is compatible with established chip manufacturing technologies and capable of producing high throughput microbatteries on a wafer surface.
The team behind the world’s smallest battery says it could be used in the human body, where tiny sensors and actuators require a continuous power supply. They also claim that the rechargeable microbatteries could also power the world’s smallest computer chips for about ten hours – for example, to measure the local ambient temperature continuously. In addition, it has great potential in future micro-and nanoelectronic sensorics and actuator technologies, in the Internet of Things, miniaturized medical implants, microrobotic systems, and ultra–flexible electronics.