For decades, extremal black holes were considered mathematically impossible. A new proof reveals otherwise.
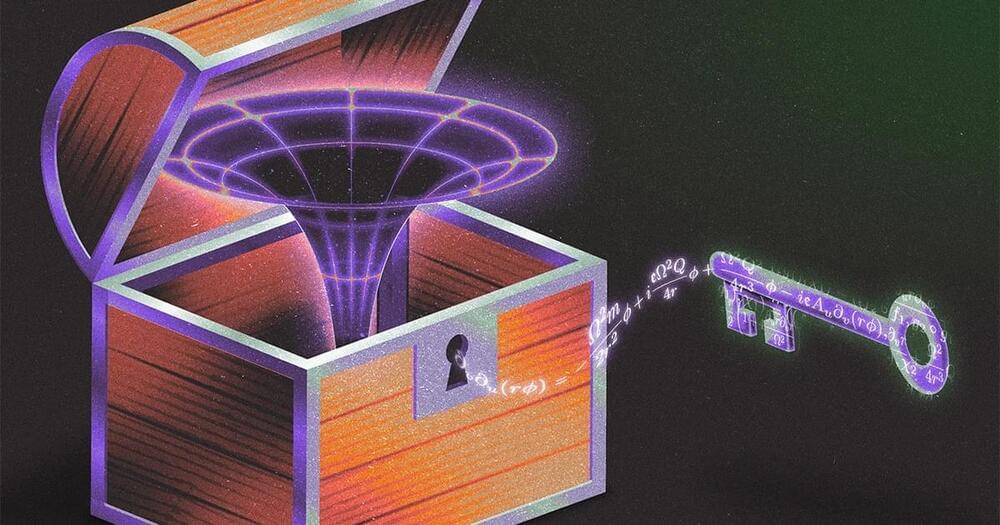
A new theory suggests time travel might be possible without creating paradoxes.
TL;DR:
A physics student from the University of Queensland, Germain Tobar, has developed a groundbreaking theory that could make time travel possible without creating paradoxes. Tobar’s calculations suggest that space-time can adjust itself to avoid inconsistencies, meaning that even if a time traveler were to change the past, the universe would correct itself to prevent any disruptions to the timeline. This theory offers a new perspective on time loops and free will, aligning with Einstein’s predictions. While the math is sound, actual time travel remains a distant possibility.
“This is going to be the fastest AI computer ever launched to space,” Yanni Barghouty, CSC’s cofounder and CEO, told Space.com. “The goal of this mission is simply to demonstrate the successful operation of an AI-capable Nvidia GPU on orbit with minimal to no errors while operating.”
The GPU will fly aboard a cubesat built by San Francisco-based company Aethero, a maker of high-performance, space-rated computers. The GPU’s only task during its four-month orbital mission will be to make mathematical calculations, the results of which will be beamed to Earth and carefully checked.
The Geometric Langlands Correspondence. Edward Frenkel is a renowned mathematician and professor at the University of California, Berkeley, known for his work in representation theory, algebraic geometry, and mathematical physics. Edward is also the author of the bestselling book “Love and Math: The Heart of Hidden Reality”, which bridges the gap between mathematics and the broader public.
Listen on Spotify: https://open.spotify.com/show/4gL14b9…
Become a YouTube Member Here:
/ @theoriesofeverything.
Patreon: / curtjaimungal (early access to ad-free audio episodes!)
Join TOEmail at https://www.curtjaimungal.org.
LINKS:
Terry Tao is one of the world’s leading mathematicians and winner of many awards including the Fields Medal. He is Professor of Mathematics at the University of California, Los Angeles (UCLA). Following his talk, Terry is in conversation with fellow mathematician Po-Shen Loh.
The Oxford Mathematics Public Lectures are generously supported by XTX Markets.
Mathematical solutions to thorny quantum problems can be found more quickly by exploiting the correspondence between the statistical methods used in deep learning and techniques for implementing quantum simulations, a team led by a RIKEN researcher has shown in a new study published in the Journal of High Energy Physics.
Professor Donald Hoffman is a cognitive neuroscientist and the author of more than 90 scientific papers and three books, including Visual Intelligence and The Case Against Reality.
He is best known for his theory of consciousness, which combines evolutionary theory with mathematics to make a compelling case that the reality we see every day is an illusion created by our minds.
In this conversation, we explore:
— The groundbreaking scientific research being conducted by physicists into the “structures” beyond spacetime.
— Donald’s theory of conscious agents.
— The implications his theory of consciousness has for our understanding of the purpose of life.
Dr. Asela Abeya, of SUNY Poly faculty in the Department of Mathematics and Physics, has collaborated with peers at the University at Buffalo and Rensselaer Polytechnic Institute on a research paper titled “On Maxwell-Bloch systems with inhomogeneous broadening and one-sided nonzero background,” which has been published in Communications in Mathematical Physics.