Neural changes in pregnancy are not well understood. Here Pritschet et al. present an open-access precision brain imaging resource, mapping neuroanatomical change in an individual from preconception through postpartum.
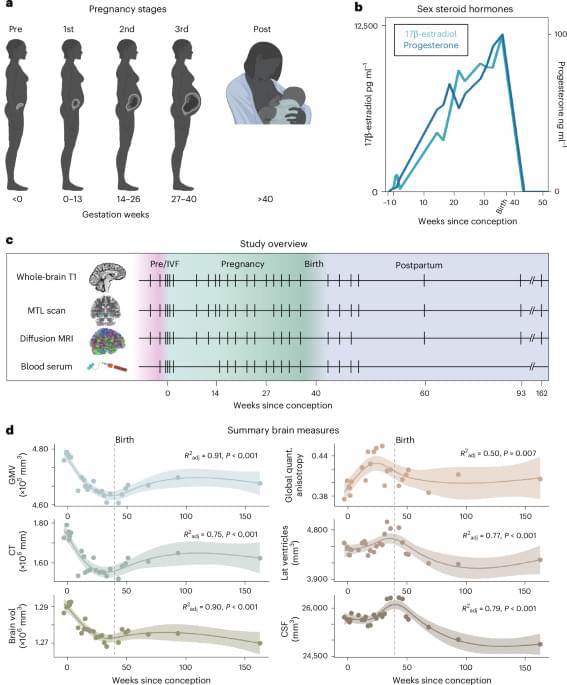
A QUT-led study analyzing data from NASA’s Perseverance rover has uncovered compelling evidence of multiple mineral-forming events just beneath the Martian surface—findings that bring scientists one step closer to answering the profound question: did life ever exist on Mars?
The QUT research team led by Dr. Michael Jones, from the Central Analytical Research Facility and the School of Chemistry and Physics, includes Associate Professor David Flannery, Associate Professor Christoph Schrank, Brendan Orenstein and Peter Nemere, together with researchers from North America and Europe.
The paper, “In-situ crystallographic mapping constrains sulfate precipitation and timing in Jezero crater, Mars” is published in Science Advances.
Trying to grasp the brain’s complexity is a little like trying to comprehend the vastness of space – it feels way beyond our scope of understanding.
By mapping a small part of a mouse brain down to an amazing level of detail, new research could help us grasp the magnitude of the neurological cosmos inside our heads.
Though the volume of brain matter analyzed was barely the size of a grain of sand, the researchers still had to describe the relationships between 84,000 neurons via half a billion synapse connections and 5.4 kilometers (3.4 miles) of neural wiring.
NASA’s new space telescope, just opened its eyes to the universe and delivered its very first images from space. Though not yet fully calibrated, the images already showcase a sweeping view filled with stars and galaxies — over 100,000 sources in each frame.
SPHEREx detects invisible infrared light and splits it into 102 hues to reveal secrets about the origins of water, galaxy distances, and even the physics of the early universe. With all systems working and its ultra-chilled detectors focused and functional, the mission promises to revolutionize cosmic surveys by mapping the entire sky multiple times and complementing more focused telescopes like Hubble and Webb.
“I give you God’s view,” said Toby Cubitt, a physicist turned computer scientist at University College London and part of the vanguard of the current charge into the unknowable, and “you still can’t predict what it’s going to do.”
Eva Miranda, a mathematician at the Polytechnic University of Catalonia (UPC) in Spain, calls undecidability a “next-level chaotic thing.”
Undecidability means that certain questions simply cannot be answered. It’s an unfamiliar message for physicists, but it’s one that mathematicians and computer scientists know well. More than a century ago, they rigorously established that there are mathematical questions that can never be answered, true statements that can never be proved. Now physicists are connecting those unknowable mathematical systems with an increasing number of physical ones and thereby beginning to map out the hard boundary of knowability in their field as well.
The frequency regime lying in the shortwave infrared (SWIR) has very unique properties that make it ideal for several applications, such as being less affected by atmospheric scattering as well as being “eye-safe.” These include Light Detection and Ranging (LIDAR), a method for determining ranges and distances using lasers, space localization and mapping, adverse weather imaging for surveillance and automotive safety, environmental monitoring, and many others.
However, SWIR light is currently confined to niche areas, like scientific instrumentation and military use, mainly because SWIR photodetectors rely on expensive and difficult-to-manufacture materials. In the past few years, colloidal quantum dots —solution-processed semiconducting nanocrystals—have emerged as an alternative for mainstream consumer electronics.
While toxic heavy-metals (like lead or mercury) have typically been used, quantum dots can also be made with environmentally friendly materials such as silver telluride (Ag2Te). In fact, silver telluride colloidal quantum dots show device performance comparable to their toxic counterparts. But they are still in their infancy, and several challenges must be addressed before they can be used in practical applications.
A team of physicists have discovered a new approach that redefines the conception of a black hole by mapping out their detailed structure, as shown in a research study recently published in Journal of High Energy Physics.
The study details new theoretical structures called “supermazes” that offer a more universal picture of black holes to the field of theoretical physics. Based in string theory, supermazes are pivotal to understanding the structure of black holes on a microscopic level.
“General relativity is a powerful theory for describing the large-scale structure of black holes, but it is a very, very blunt instrument for describing black-hole microstructure,” said Nicholas Warner, co-author of the study and professor of physics, astronomy and mathematics at the USC Dornsife College of Letters, Arts and Sciences. In a framework of theories extending beyond Einstein’s equations, supermazes provide a detailed portrait of the microscopic structure of brane black holes.
Network models provide a flexible way of representing objects and their multifaceted relationships. Deriving a network entails mapping hidden structures in inevitably noisy data—a critical task known as reconstruction. Now Gang Yan and Jia-Jie Qin of Tongji University in China have provided a mathematical proof showing what makes some networks easier to reconstruct than others [1].
Complex systems in biology, physics, and social sciences tend to involve a vast number of interacting entities. In a network model, these entities are represented by nodes, linked by connections weighted to describe the strength of each interaction. Yan and Qin took an empirical dataset and used a statistical inference method to calculate the likelihood that any pair of nodes is directly linked. Then, based on the true positive and false positive rates of these inferred connections, they analyzed the fidelity of the reconstructed networks. They found that the most faithful reconstructions are obtained with systems for which the number of connections per node varies most widely across the network. Yan and Qin saw the same tendency when they tested their model on synthetic and real networks, including metabolic networks, plant-pollinator webs, and power grids.
With the rapid increase in available data across research areas, network reconstruction has become an important tool for studying complex systems. Yan and Qin say their new result both solves the problem of what complex systems can be easily mapped into a network and provides a solid foundation for developing methods of doing so.
Researchers at the University of Virginia have created the first comprehensive protein-level atlas of brain development, providing unprecedented insight into how the brain forms and potential implications for understanding neurological disorders. The study, published in Nature Neuroscience, analyzed over 24 million individual cells from mouse brains, revealing detailed molecular pathways that guide brain development from early embryonic stages through early postnatal development.
The research team, led by Professors Christopher Deppmann and Eli Zunder, used an innovative technique called mass cytometry to track 40 different proteins across various brain regions and developmental stages. The approach provided a more detailed view of cellular function than previous studies that primarily examined RNA.
“While RNA studies have given us important insights, proteins are the actual workforce of cells,” explained Deppmann, a professor in the College and Graduate School of Arts & Sciences’ Department of Biology. “By studying proteins directly, we can better understand how cells are functioning and communicating during brain development.”