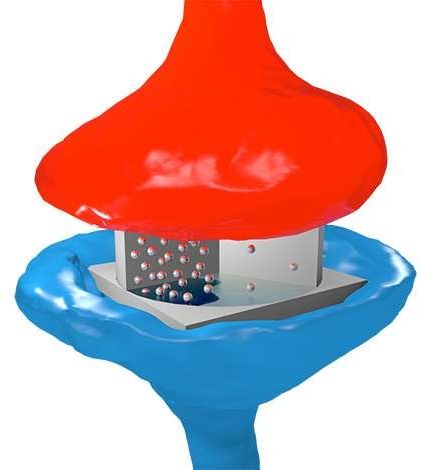
One of the goals of biomimetics is to take inspiration from the functioning of the brain in order to design increasingly intelligent machines. This principle is already at work in information technology, in the form of the algorithms used for completing certain tasks, such as image recognition; this, for instance, is what Facebook uses to identify photos. However, the procedure consumes a lot of energy. Vincent Garcia (Unité mixte de physique CNRS/Thales) and his colleagues have just taken a step forward in this area by creating directly on a chip an artificial synapse that is capable of learning. They have also developed a physical model that explains this learning capacity. This discovery opens the way to creating a network of synapses and hence intelligent systems requiring less time and energy.
Our brain’s learning process is linked to our synapses, which serve as connections between our neurons. The more the synapse is stimulated, the more the connection is reinforced and learning improved. Researchers took inspiration from this mechanism to design an artificial synapse, called a memristor. This electronic nanocomponent consists of a thin ferroelectric layer sandwiched between two electrodes, and whose resistance can be tuned using voltage pulses similar to those in neurons. If the resistance is low the synaptic connection will be strong, and if the resistance is high the connection will be weak. This capacity to adapt its resistance enables the synapse to learn.
Although research focusing on these artificial synapses is central to the concerns of many laboratories, the functioning of these devices remained largely unknown. The researchers have succeeded, for the first time, in developing a physical model able to predict how they function. This understanding of the process will make it possible to create more complex systems, such as a series of artificial neurons interconnected by these memristors.
Read more