An exact solution of the Einstein—Maxwell equations yields a general relativistic picture of the tachyonic phenomenon, suggesting a hypothesis on the tachyon creation. The hypothesis says that the tachyon is produced when a neutral and very heavy (over 75 GeV/c^2) subatomic particle is placed in electric and magnetic fields that are perpendicular, very strong (over 6.9 × 1017 esu/cm^2 or oersted), and the squared ratio of their strength lies in the interval (1,5]. Such conditions can occur when nonpositive subatomic particles of high energy strike atomic nuclei other than the proton. The kinematical relations for the produced tachyon are given. Previous searches for tachyons in air showers and some possible causes of their negative results are discussed.
Category: information science – Page 241
Can we study AI the same way we study lab rats? Researchers at DeepMind and Harvard University seem to think so. They built an AI-powered virtual rat that can carry out multiple complex tasks. Then, they used neuroscience techniques to understand how its artificial “brain” controls its movements.
Today’s most advanced AI is powered by artificial neural networks —machine learning algorithms made up of layers of interconnected components called “neurons” that are loosely inspired by the structure of the brain. While they operate in very different ways, a growing number of researchers believe drawing parallels between the two could both improve our understanding of neuroscience and make smarter AI.
Now the authors of a new paper due to be presented this week at the International Conference on Learning Representations have created a biologically accurate 3D model of a rat that can be controlled by a neural network in a simulated environment. They also showed that they could use neuroscience techniques for analyzing biological brain activity to understand how the neural net controlled the rat’s movements.
The main idea of artificial neural networks (ANN) is to build up representations for complicated functions using compositions of relatively simple functions called layers.
A deep neural network is one that has many layers, or many functions composed together.
Although layers are typically simple functions(e.g. relu(Wx + b)) in general they could be any differentiable functions.
Quantitative biologists David McCandlish and Juannan Zhou at Cold Spring Harbor Laboratory have developed an algorithm with predictive power, giving scientists the ability to see how specific genetic mutations can combine to make critical proteins change over the course of a species’ evolution.
Described in Nature Communications, the algorithm called “minimum epistasis interpolation” results in a visualization of how a protein could evolve to either become highly effective or not effective at all. They compared the functionality of thousands of versions of the protein, finding patterns in how mutations cause the protein to evolve from one functional form to another.
“Epistasis” describes any interaction between genetic mutations in which the effect of one gene is dependent upon the presence of another. In many cases, scientists assume that when reality does not align with their predictive models, these interactions between genes are at play. With this in mind, McCandlish created this new algorithm with the assumption that every mutation matters. The term “Interpolation” describes the act of predicting the evolutionary path of mutations a species might undergo to achieve optimal protein function.
Robots could soon assist humans in a variety of fields, including in manufacturing and industrial settings. A robotic system that can automatically assemble customized products may be particularly desirable for manufacturers, as it could significantly decrease the time and effort necessary to produce a variety of products.
To work most effectively, such a robot should integrate an assembly planner, a component that plans the sequence of movements and actions that a robot should perform to manufacture a specific product. Developing an assembly planner that can rapidly plan the sequences of movements necessary to produce different customized products, however, has so far proved to be highly challenging.
Researchers at the German Aerospace Center (DLR) have recently developed an algorithm that can transfer knowledge acquired by a robot while assembling products in the past to the assembly of new items. This algorithm, presented in a paper published in IEEE Robotics and Automation Letters, can ultimately reduce the amount of time required by an assembly planner to come up with action sequences for the manufacturing of new customized products.
Stephen Wolfram is a cult figure in programming and mathematics. He is the brains behind Wolfram Alpha, a website that tries to answer questions by using algorithms to sift through a massive database of information. He is also responsible for Mathematica, a computer system used by scientists the world over.
Last week, Wolfram launched a new venture: the Wolfram Physics Project, an ambitious attempt to develop a new physics of our Universe.
The new physics, he declares, is computational. The guiding idea is that everything can be boiled down to the application of simple rules to fundamental building blocks.
A marimba-playing robot with four arms and eight sticks is writing and playing its own compositions in a lab at the Georgia Institute of Technology. The pieces are generated using artificial intelligence and deep learning.
Researchers fed the robot nearly 5,000 complete songs — from Beethoven to the Beatles to Lady Gaga to Miles Davis — and more than 2 million motifs, riffs and licks of music. Aside from giving the machine a seed, or the first four measures to use as a starting point, no humans are involved in either the composition or the performance of the music.
The first two compositions are roughly 30 seconds in length. The robot, named Shimon, can be seen and heard playing them here and here.
Using machine learning three groups, including researchers at IBM and DeepMind, have simulated atoms and small molecules more accurately than existing quantum chemistry methods. In separate papers on the arXiv preprint server the teams each use neural networks to represent wave functions of electrons that surround the molecules’ atoms. This wave function is the mathematical solution of the Schrödinger equation, which describes the probabilities of where electrons can be found around molecules. It offers the tantalising hope of ‘solving chemistry’ altogether, simulating reactions with complete accuracy. Normally that goal would require impractically large amounts of computing power. The new studies now offer a compromise of relatively high accuracy at a reasonable amount of processing power.
Each group only simulates simple systems, with ethene among the most complex, and they all emphasise that the approaches are at their very earliest stages. ‘If we’re able to understand how materials work at the most fundamental, atomic level, we could better design everything from photovoltaics to drug molecules,’ says James Spencer from DeepMind in London, UK. ‘While this work doesn’t achieve that quite yet, we think it’s a step in that direction.’
Two approaches appeared on arXiv just a few days apart in September 2019, both combining deep machine learning and Quantum Monte Carlo (QMC) methods. Researchers at DeepMind, part of the Alphabet group of companies that owns Google, and Imperial College London call theirs Fermi Net. They posted an updated preprint paper describing it in early March 2020.1 Frank Noé’s team at the Free University of Berlin, Germany, calls its approach, which directly incorporates physical knowledge about wave functions, PauliNet.2
An artificial neural network can reveal patterns in huge amounts of gene expression data, and discover groups of disease-related genes. This has been shown by a new study led by researchers at Linköping University, published in Nature Communications. The scientists hope that the method can eventually be applied within precision medicine and individualised treatment.
It’s common when using social media that the platform suggests people whom you may want to add as friends. The suggestion is based on you and the other person having common contacts, which indicates that you may know each other. In a similar manner, scientists are creating maps of biological networks based on how different proteins or genes interact with each other. The researchers behind a new study have used artificial intelligence, AI, to investigate whether it is possible to discover biological networks using deep learning, in which entities known as “artificial neural networks” are trained by experimental data. Since artificial neural networks are excellent at learning how to find patterns in enormous amounts of complex data, they are used in applications such as image recognition. However, this machine learning method has until now seldom been used in biological research.
“We have for the first time used deep learning to find disease-related genes. This is a very powerful method in the analysis of huge amounts of biological information, or ‘big data’,” says Sanjiv Dwivedi, postdoc in the Department of Physics, Chemistry and Biology (IFM) at Linköping University.
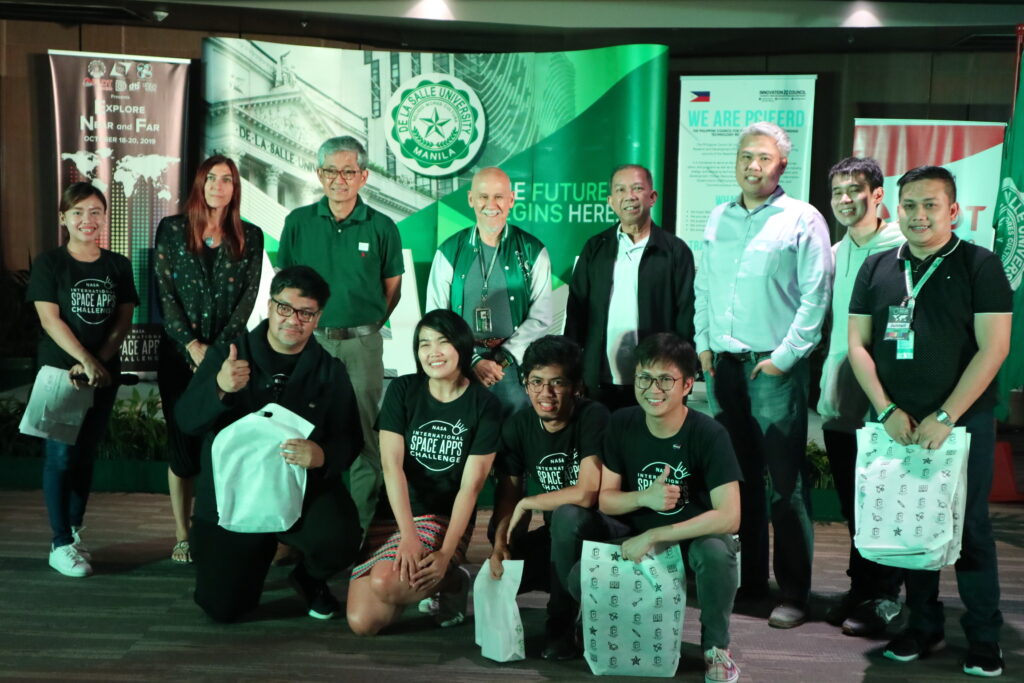
MANILA, Philippines — A dengue case forecasting system using space data made by Philippine developers won the 2019 National Aeronautics and Space Administration’s International Space Apps Challenge. Over 29,000 participating globally in 71 countries, this solution made it as one of the six winners in the best use of data, the solution that best makes space data accessible, or leverages it to a unique application.
Dengue fever is a viral, infectious tropical disease spread primarily by Aedes aegypti female mosquitoes. With 271,480 cases resulting in 1,107 deaths reported from January 1 to August 31, 2019 by the World Health Organization, Dominic Vincent D. Ligot, Mark Toledo, Frances Claire Tayco, and Jansen Dumaliang Lopez from CirroLytix developed a forecasting model of dengue cases using climate and digital data, and pinpointing possible hotspots from satellite data.
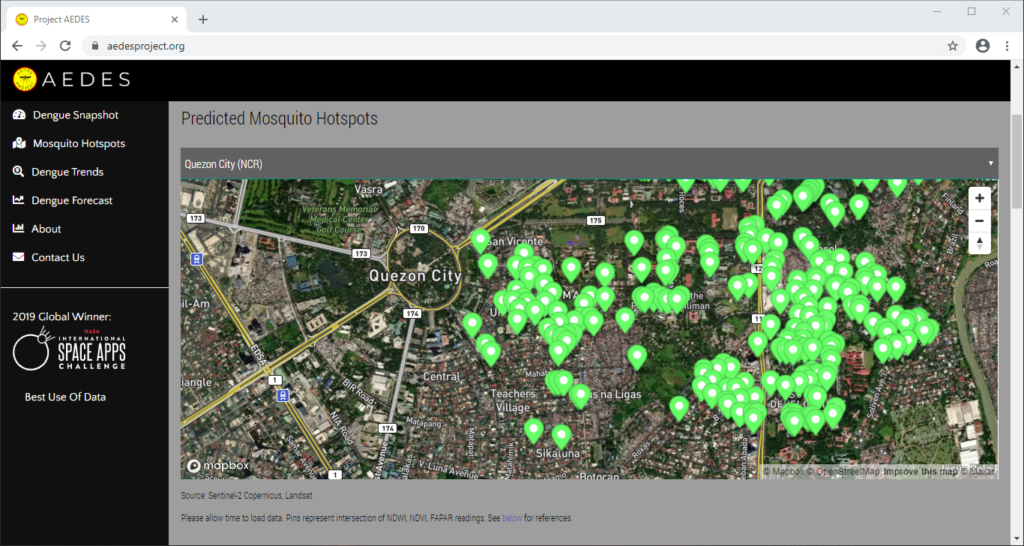
Correlating information from Sentinel-2 Copernicus and Landsat 8 satellites, climate data from the Philippine Atmospheric, Geophysical and Astronomical Services Administration of the Department of Science and Technology (DOST-PAGASA) and trends from Google search engines, potential dengue hotspots will be shown in a web interface.