Oct 28, 2022
Meta what?
Posted by Henrique Jorge in categories: augmented reality, big data, computing, evolution, futurism, information science, innovation, internet, life extension, machine learning, Mark Zuckerberg, posthumanism, singularity, virtual reality
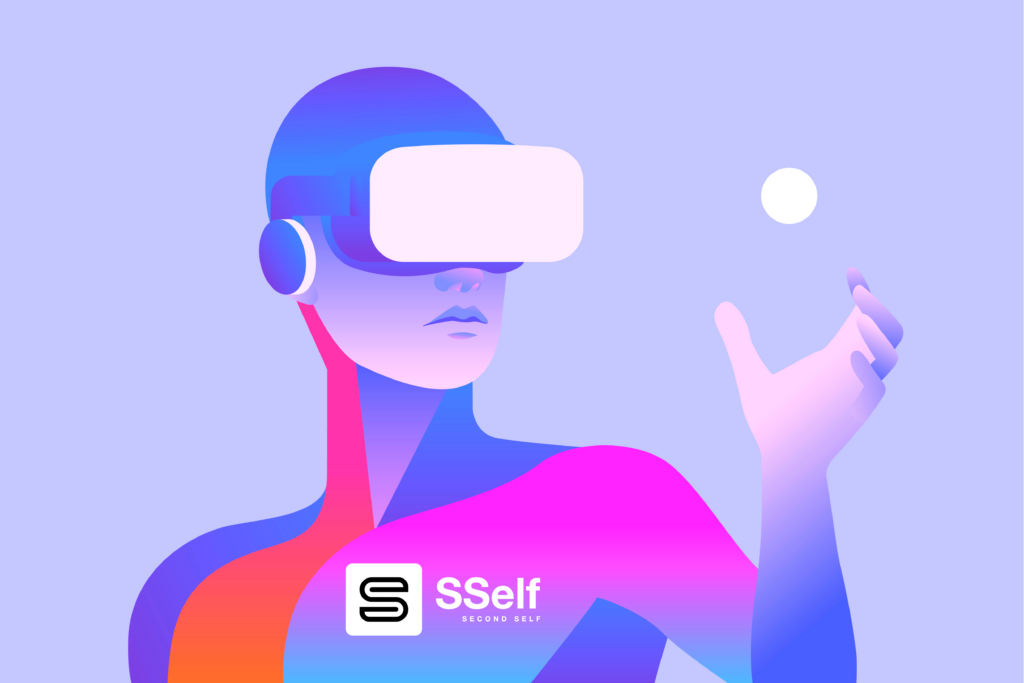
When in 2015, Eileen Brown looked at the ETER9 Project (crazy for many, visionary for few) and wrote an interesting article for ZDNET with the title “New social network ETER9 brings AI to your interactions”, it ensured a worldwide projection of something the world was not expecting.
Someone, in a lost world (outside the United States), was risking, with everything he had in his possession (very little or less than nothing), a vision worthy of the American dream. At that time, Facebook was already beginning to annoy the cleaner minds that were looking for a difference and a more innovative world.
Today, after that test bench, we see that Facebook (Meta or whatever) is nothing but an illusion, or, I dare say, a big disappointment. No, no, no! I am not now bad-mouthing Facebook just because I have a project in hand that is seen as a potential competitor.
I was even a big fan of the “original” Facebook; but then I realized, it took me a few years, that Mark Zuckerberg is nothing more than a simple kid, now a man, who against everything and everyone, gave in to whims. Of him, initially, and now, perforce, of what his big investors, deluded by himself, of what his “metaverse” would be.