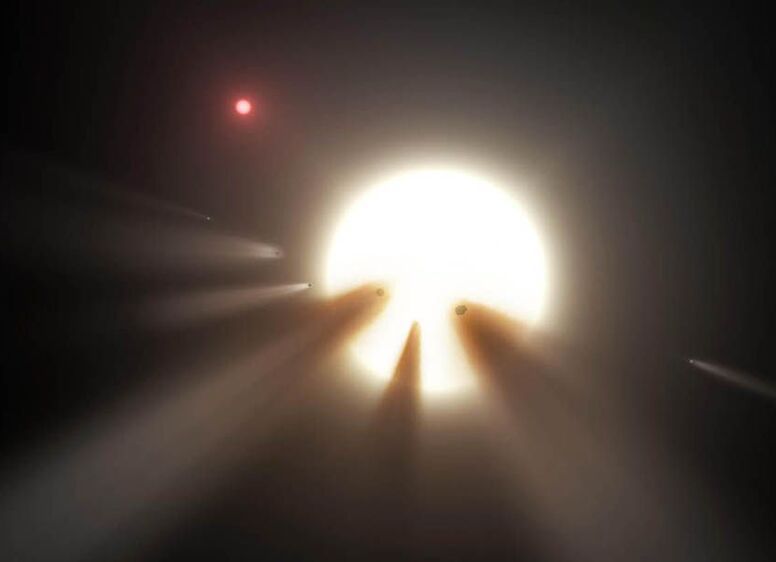
Within the Milky Way, there are an estimated 200 to 400 billion stars, all of which orbit around the center of our galaxy in a coordinated cosmic dance. As they orbit, stars in the galactic disk (where our Sun is located) periodically shuffle about and get closer to one another. At times, this can have a drastic effect on the star that experience a close encounter, disrupting their systems and causing planets to be ejected.
Knowing when stars will make a close encounter with our Solar System, and how it might shake-up objects within it, is therefore a concern to astronomers. Using data collected by the Gaia Observatory, two researchers with the Russian Academy of Sciences (RAS) determined that a handful of stars will be making close passes by our Solar System in the future, one of which will stray pretty close!
The study was conducted by Vadim V. Bobylev and Anisa T. Bajkova, two researchers from the Pulkovo Observatory’s Laboratory of Galaxy Dynamics in St. Petersburg, Russia. As they indicated, they relied on astrometric data from the Gaia mission’s Early Data Release 3 (EDR3), which revealed kinematic characteristics of stars that are expected to pass within 3.26 light-years (1 Parsec) with the Solar System in the future.