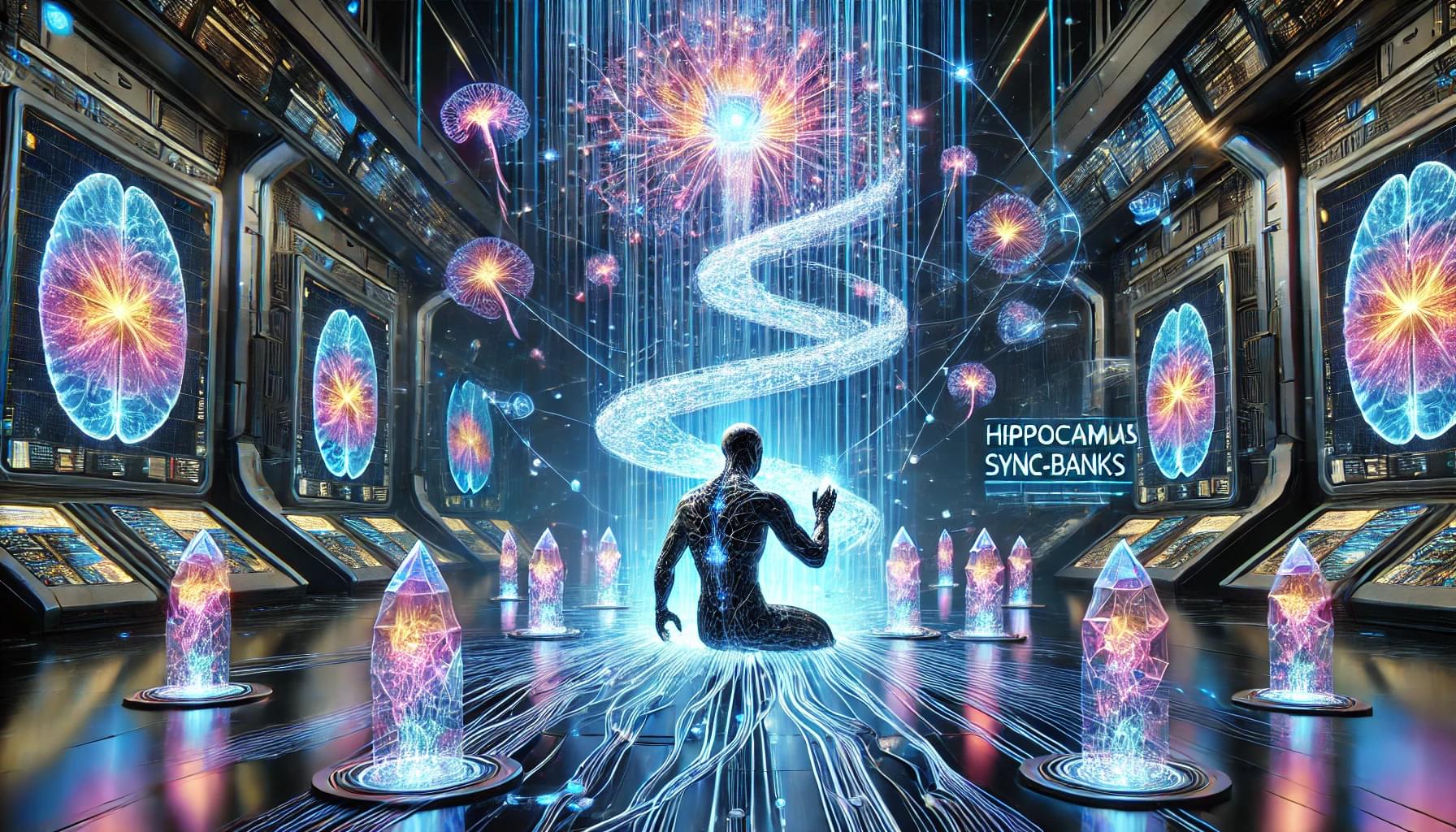
Once the detection mechanism is refined, the next milestone would be to interface that optical signal with a small experimental crystal. The choice of crystal is not arbitrary. Labs might experiment with rare-earth-ion-doped crystals like praseodymium-doped yttrium silicate, known for their capacity to store quantum information for microseconds to milliseconds, or possibly even seconds, under specialized conditions. At an early stage, the device would not store large swaths of complex data but might capture discrete bursts of neural activity corresponding to short-term memory formation. By demonstrating that these bursts can be reliably “written” into the crystal and subsequently “read” out at a later time, researchers would confirm the fundamental principle behind Hippocampus Sync-Banks: that ephemeral neural codes can be transcribed into a stable external medium.
Of course, storing a fleeting pattern is just one half of the puzzle. To realize the Sync-Bank concept fully, the same pattern must be reintroduced into the brain in a way that the hippocampus recognizes. Here, scientists would leverage neural stimulation techniques. In theory, the crystal would “release” the stored patterns in the form of carefully modulated optical or electrical signals. Specialized interfaces near or within the hippocampus—perhaps using microLED arrays or sophisticated electrode grids—would then convert those signals back into the language of the neurons. If the signals are replayed with the correct timing and intensity, the hippocampus might treat them as though they are its own native memory patterns, thereby reactivating the memory. Experimental validation could involve training an animal to associate a particular stimulus with a reward, capturing the neural trace, and then seeing if artificially stimulating that trace at a later time recalls the memory even in the absence of the original stimulus.
Such experiments would inevitably confront thorny technical issues. Neurons and synapses adapt or “rewire” themselves as learning progresses, and the hippocampus is far from static. Overlapping memory traces often share neurons, meaning that reintroducing one memory trace might partially interfere with or activate another. To address this, scientists would need real-time feedback loops that track how the hippocampus responds to artificial signals. Machine learning algorithms might adjust the reintroduced signal to better fit the updated neural state, ensuring that the stored pattern does not clash with changes in the memory landscape. In other words, a second or third generation of prototypes could incorporate adaptive feedback, not just a one-way feed of recorded data. This type of refinement would be crucial to the user’s experience, because we do not simply recall memories as static snapshots; each time we remember something, our brains incorporate subtle new contexts and associations.