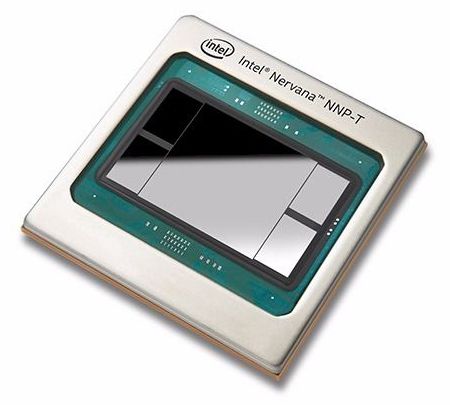
At this yearâs Intel AI Summit, the chipmaker demonstrated its first-generation Neural Network Processors (NNP): NNP-T for training and NNP-I for inference. Both product lines are now in production and are being delivered to initial customers, two of which, Facebook and Baidu, showed up at the event to laud the new chippery.
The purpose-built NNP devices represent Intelâs deepest thrust into the AI market thus far, challenging Nvidia, AMD, and an array of startups aimed at customers who are deploying specialized silicon for artificial intelligence. In the case of the NNP products, that customer base is anchored by hyperscale companies â Google, Facebook, Amazon, and so on â whose businesses are now all powered by artificial intelligence.
Naveen Rao, corporate vice president and general manager of the Artificial Intelligence Products Group at Intel, who presented the opening address at the AI Summit, says that the companyâs AI solutions are expected to generate more than $3.5 billion in revenue in 2019. Although Rao didnât break that out into specific products sales, presumably it includes everything that has AI infused in the silicon. Currently, that encompasses nearly the entire Intel processor portfolio, from the Xeon and Core CPUs, to the Altera FPGA products, to the Movidius computer vision chips, and now the NNP-I and NNP-T product lines. (Obviously, that figure can only include the portion of Xeon and Core revenue that is actually driven by AI.)