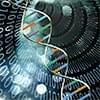
Researchers from North Carolina State University and Johns Hopkins University have demonstrated a technology capable of a suite of data storage and computing functions – repeatedly storing, retrieving, computing, erasing or rewriting data – that uses DNA rather than conventional electronics. Previous DNA data storage and computing technologies could complete some but not all of these tasks.
“In conventional computing technologies, we take for granted that the ways data are stored and the way data are processed are compatible with each other,” says project leader Albert Keung, co-corresponding author of a paper on the work (Nature Nanotechnology, “A Primordial DNA Store and Compute Engine”). “But in reality, data storage and data processing are done in separate parts of the computer, and modern computers are a network of complex technologies,” Keung is an associate professor of chemical and biomolecular engineering and a Goodnight Distinguished Scholar at NC State.
“DNA computing has been grappling with the challenge of how to store, retrieve and compute when the data is being stored in the form of nucleic acids,” Keung says. “For electronic computing, the fact that all of a device’s components are compatible is one reason those technologies are attractive. But, to date, it’s been thought that while DNA data storage may be useful for long-term data storage, it would be difficult or impossible to develop a DNA technology that encompassed the full range of operations found in traditional electronic devices: storing and moving data; the ability to read, erase, rewrite, reload or compute specific data files; and doing all of these things in programmable and repeatable ways.