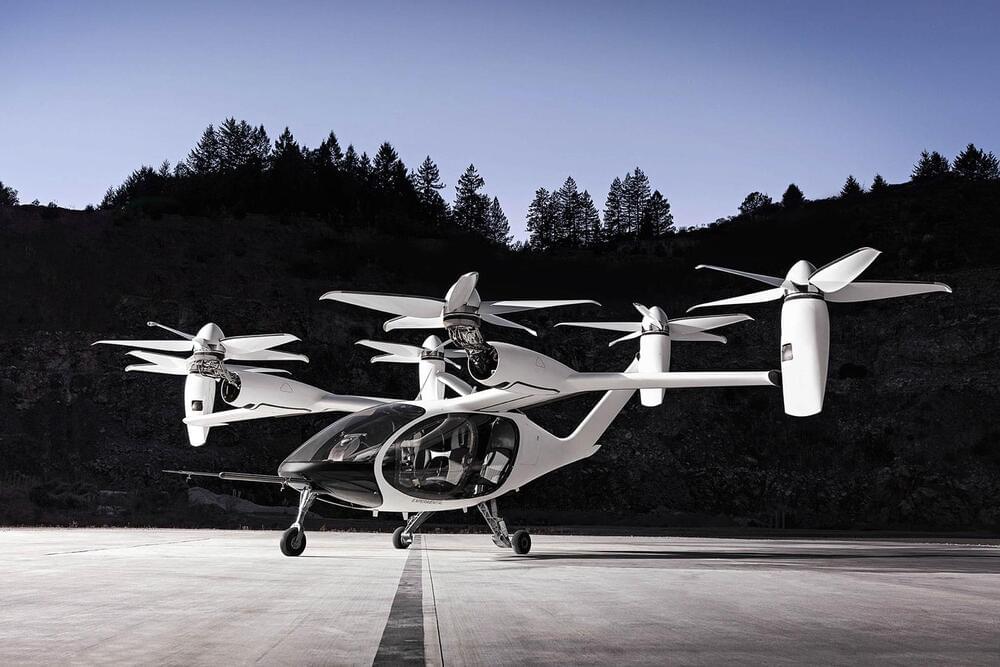
The automotive electric vehicle revolution paves the way for urban air mobility, but people must not be naive to believe that electric vehicle batteries are enough for electric flight. The need for fast charging, 30 times the energy throughput, and three times the power demand requires a new generation of batteries.
Engineers at Penn State have now demonstrated two energy-dense lithium-ion batteries that can recharge with enough energy for a 50-mile eVTOL trip in five to ten minutes. These batteries could sustain more than 2,000 fast charges over their lifetime.
In the last couple of years, several prototypes have emerged – including from companies like Volocopter, Boeing, Lilium, SkyDrive. While some prototypes have included wheels, they all incorporate spinning rotors to facilitate takeoff and landing, including the air taxi shown off last year by Hyundai, which is basically a small helicopter.