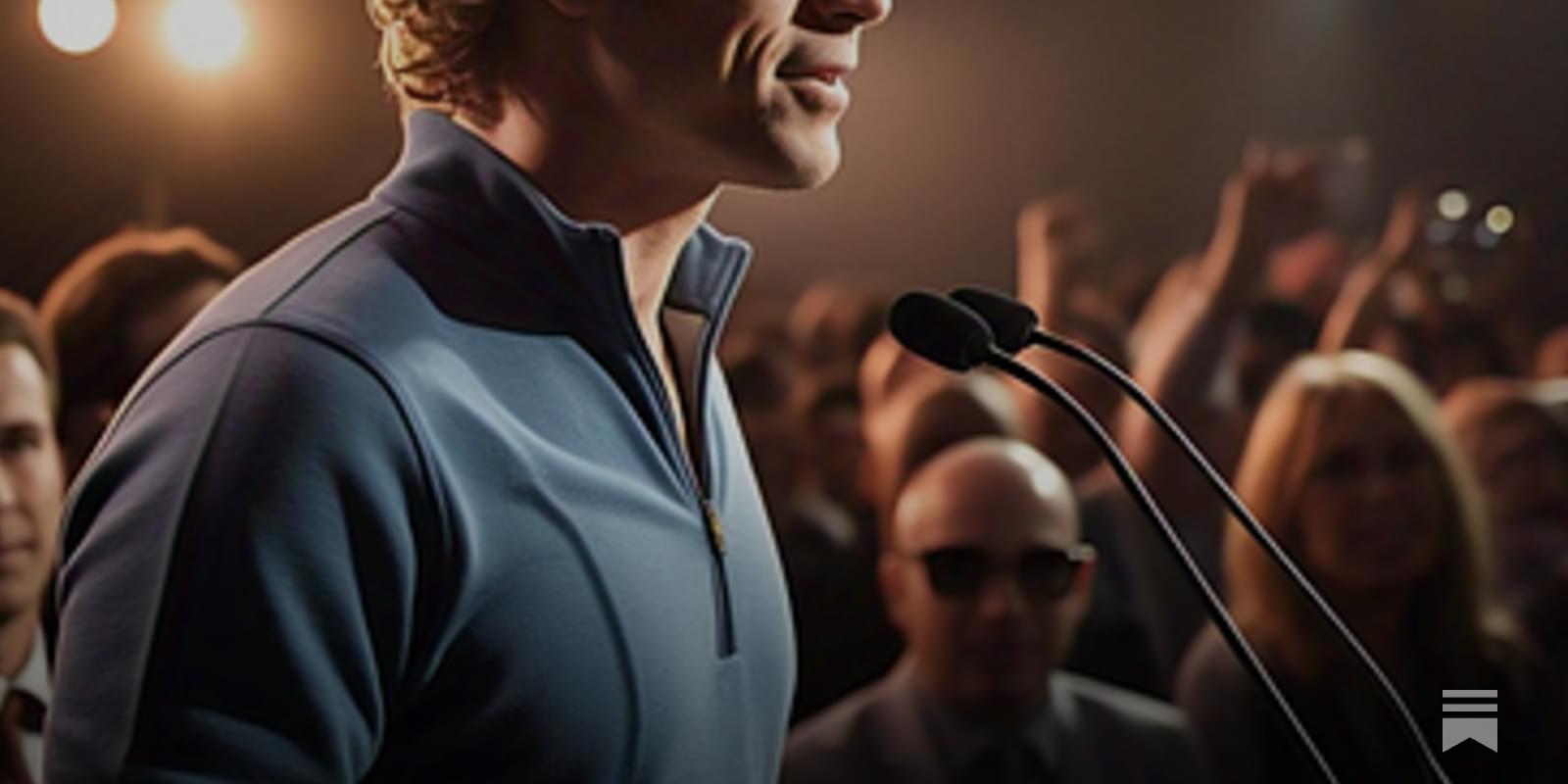
In today’s AI news, for those who were thinking Meta Platforms Inc. might back down from its heavy-spending ways in the wake of the DeepSeek news, think again. On the earnings call, Zuckerberg spoke of “the hundreds of billions of dollars” Meta will invest in AI infrastructure over the long term.
S ChatGPT and the newly ascending DeepSeek. “Qwen 2.5-Max outperforms… almost across the board GPT-4o, DeepSeek-V3 and Llama-3.1-405B,” Alibaba Meanwhile, amid the market turbulence and breathless headlines, Dario Amodei, co-founder of Anthropic and one of the pioneering researchers behind today’s large language models, published a detailed analysis that offers a more nuanced perspective on DeepSeek’s achievements.
And, Microsoft is bringing Chinese AI company DeepSeek’s R1 model to its Azure AI Foundry platform and GitHub. The R1 model is now part of a model catalog on Azure AI Foundry and GitHub — allowing Microsoft’s customers to integrate it into their AI applications.
In videos, Reid Hoffman is the co-founder of LinkedIn, a legendary Silicon Valley investor, and author of the new book Superagency: What Could Possibly Go Right with Our AI Future. Hoffman joins Big Technology Podcast to discuss his optimistic case for AI, the massive investments flooding into the field, and whether they can pay off.
And, in the new episode of Moonshots, Emad Mostaque, Salim Ismail, and Peter Diamandis discuss recent DeepSeek news, the China vs. USA AI race, and more. Emad is the founder of Intelligent Internet and former CEO of Stability AI. Salim is a serial entrepreneur and strategist well known for his expertise in exponential organizations.
Then, reverse the diffusion process, and unlock the secrets of AI-generated images. Isaac Ke explores how to harness the power of diffusion models to create stunning, high-quality images from text prompts. From text-to-image generation to image-to-image editing, learn how diffusion models are being applied in various fields.
We close out with, Nate B. Jones sharing the strategy behind DeepSeek strategically launching free AI products to disrupt OpenAI’s dominance, including an Operator clone, voice AI, and a likely Sora competitor. This aggressive move threatens OpenAI’s paid model and forces competitors to rethink pricing.