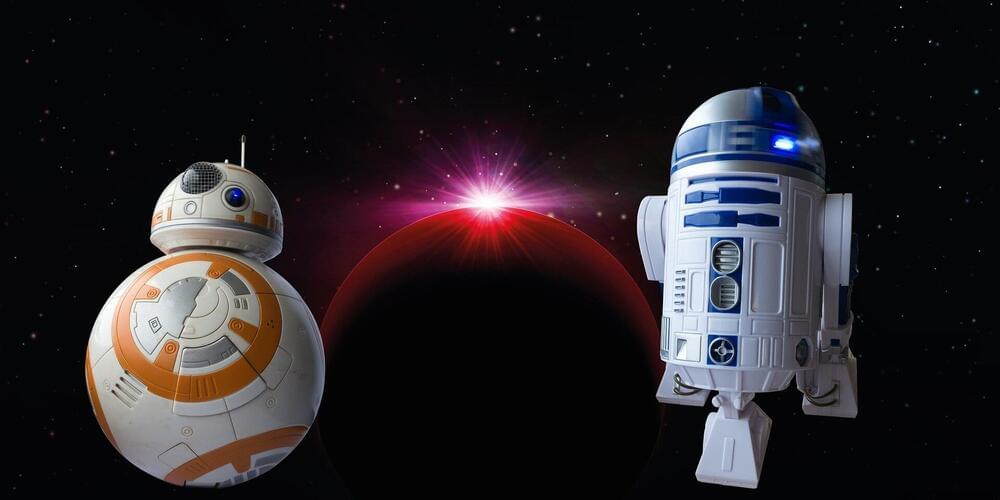
Robin Murphy, a roboticist at Texas A&M University has published a Focus piece in the journal Science Robotics outlining her views on the robots portrayed in “Star Wars,” most particularly those featured in “The Mandalorian” and “The Book of Boba Fett.” In her article, she says she believes that the portrayals of robots in both movies are quite creative, but suggests they are not wild enough to compete with robots that are made and used in the real world today.
Murphy begins by noting that one robot in particular, IG-11 in the Mandalorian, makes for good viewing with a rotating head that allows for shooting at targets in any direction, but she also notes that such a robot would very likely be overly susceptible to joint failure and would be saddled with huge computational demands. She suggests a more practical design would involve the use of fixed-array sensors.
Murphy also notes that robots in “Star Wars” movies do fail on occasion, generally during suspenseful scenes, which she further notes might explain why the empire met with its demise. As just one example, she wonders why the stormtroopers so often miss their targets. She also notes that in some ways, droids in “Star Wars” movies tend to be far more advanced than droids in the real world, allowing them to hold human-like jobs such as bartending, teaching or translating. In so doing, she points out, producers of the movies have shied away from showing them doing more mundane work, like mining.