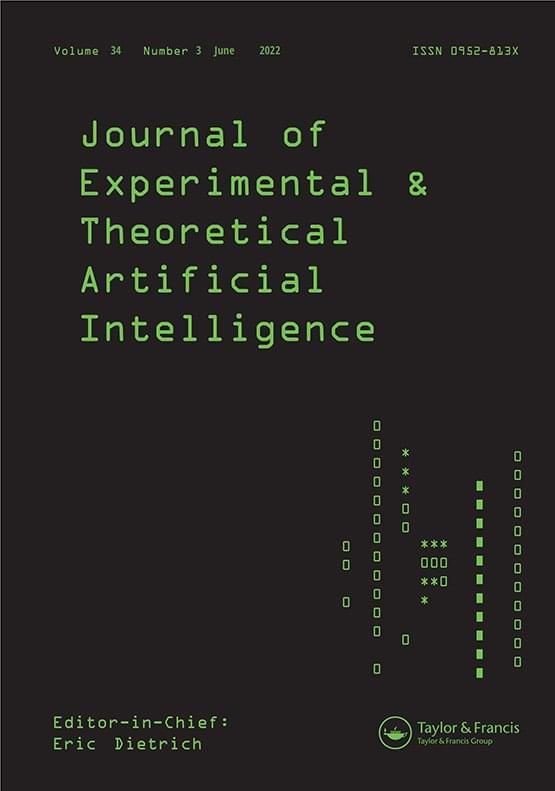
(2022). Journal of Experimental & Theoretical Artificial Intelligence. Ahead of Print.
AI has for decades attempted to code commonsense concepts, e.g., in knowledge bases, but struggled to generalise the coded concepts to all the situations a human would naturally generalise them to, and struggled to understand the natural and obvious consequences of what it has been told. This led to brittle systems that did not cope well with situations beyond what their designers envisaged. John McCarthy (1968) said ‘a program has common sense if it automatically deduces for itself a sufficiently wide class of immediate consequences of anything it is told and what it already knows’; that is a problem that has still not been solved. Dreifus (1998) estimated that ‘Common sense is knowing maybe 30 or 50 million things about the world and having them represented so that when something happens, you can make analogies with others’. Minsky presciently noted that common sense would require the capability to make analogical matches between knowledge and events in the world, and furthermore that a special representation of knowledge would be required to facilitate those analogies. We can see the importance of analogies for common sense in the way that basic concepts are borrowed, e.g., the tail of an animal, or the tail of a capital ‘Q’, or the tail-end of a temporally extended event (see also examples of ‘contain’, ‘on’, in Sec. 5.3.1). More than this, for known facts, such as ‘a string can pull but not push an object’, an AI system needs to automatically deduce (by analogy) that a cloth, sheet, or ribbon, can behave analogously to the string. For the fact ‘a stone can break a window’, the system must deduce that any similarly heavy and hard object is likely to break any similarly fragile material. Using the language of Sec. 5.2.1, each of these known facts needs to be treated as a schema,14 and then applied by analogy to new cases.
Projection is a mechanism that can find analogies (see Sec. 5.3.1) and hence could bridge the gap between models of commonsense concepts (i.e., not the entangled knowledge in word embeddings learnt from language corpora) and text or visual or sensorimotor input. To facilitate this, concepts should be represented by hierarchical compositional models, with higher levels describing relations among elements in the lower-level components (for reasons discussed in Sec. 6.1). There needs to be an explicit symbolic handle on these subcomponents; i.e., they cannot be entangled in a complex network. For visual object recognition, a concept can simply be a set of spatial relations among component features, but higher concepts require a complex model involving multiple types of relations, partial physics theories, and causality. Secs. 5.2 and 5.3 give a hint of what these concepts may look like, but a full example requires a further paper.
Moving beyond the recognition of individual concepts, a complete cognitive system needs to represent and simulate what is happening in a situation, based on some input, e.g., text, visual. This means instantiating concepts in some workspace to flesh out relevant details of a scenario. Sometimes very little data is available for some part of a scenario, and it must be imagined. For example, suppose some machine in a wooden casing moves smoothly across a surface, but the viewer cannot see what mechanism is on the underside, the viewer may conjecture it rolls on wheels, and if it gets stuck one may imagine a wheel hitting a small stone. This type of imagination is another projection: assuming a prior model of a wheeled vehicle is available, then the parts of this can be projected to positions in the simulation (parts unseen in the actual scenario). Similarly for a wheel hitting a stone: a schema abstracted from a previously experienced episode of such an occurrence can serve as a model. Simulation and projection must work together to imagine scenarios, because an unfolding simulation may trigger new projections. If the simulation is of something happening in the present, then sensor data can enter to constrain the possibilities for the simulation. The importance of analogy for this kind of reasoning in a human-level cognitive agent has also been recognised by other AI researchers (K. D. Forbus & Hinrichs, 2006 ; Forbus et al., 2008).