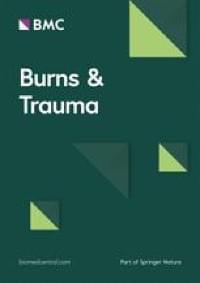
In addition to laser-assisted bioprinting, other light-based 3D bioprinting techniques include digital light processing (DLP) and two-photon polymerization (TPP)-based 3D bioprinting. DLP uses a digital micro-mirror device to project a patterned mask of ultraviolet (UV)/visible range light onto a polymer solution, which in turn results in photopolymerization of the polymer in contact [56, 57]. DLP can achieve high resolution with rapid printing speed regardless of the layerâs complexity and area. In this method of 3D bioprinting, the dynamics of the polymerization can be regulated by modulating the power of the light source, the printing rate, and the type and concentrations of the photoinitiators used. TPP, on the other hand, utilizes a focused near-infrared femtosecond laser of wavelength 800 nm to induce polymerization of the monomer solution [56]. TPP can provide a very high resolution beyond the light diffraction limit since two-photon absorption only happens in the center region of the laser focal spot where the energy is above the threshold to trigger two-photon absorption [56].
The recent development of the integrated tissue and organ printer (ITOP) by our group allows for bioprinting of human scale tissues of any shape [45]. The ITOP facilitates bioprinting with very high precision; it has a resolution of 50 ÎŒm for cells and 2 ÎŒm for scaffolding materials. This enables recapitulation of heterocellular tissue biology and allows for fabrication of functional tissues. The ITOP is configured to deliver the bioink within a stronger water-soluble gel, Pluronic F-127, that helps the printed cells to maintain their shape during the printing process. Thereafter, the Pluronic F-127 scaffolding is simply washed away from the bioprinted tissue. To ensure adequate oxygen diffusion into the bioprinted tissue, microchannels are created with the biodegradable polymer, polycaprolactone (PCL). Stable human-scale ear cartilage, bone, and skeletal muscle structures were printed with the ITOP, which when implanted in animal models, matured into functional tissue and developed a network of blood vessels and nerves [45]. In addition to the use of materials such as Pluronic F-127 and PCL for support scaffolds, other strategies for improving structural integrity of the 3D bioprinted constructs include the use of suitable thickening agents such as hydroxyapatite particles, nanocellulose, and Xanthan and gellan gum. Further, the use of hydrogel mixtures instead of a single hydrogel is a helpful strategy. For example, the use of gelatin-methacrylamide (GelMA)/hyaluronic acid (HA) mixture instead of GelMA alone shows enhanced printability since HA improves the viscosity of mixture while crosslinking of GelMA retains post-printing structural integrity [58].
To date, several studies have investigated skin bioprinting as a novel approach to reconstruct functional skin tissue [44, 59,60,61,62,63,64,65,66,67]. Some of the advantages of fabrication of skin constructs using bioprinting compared to other conventional tissue engineering strategies are the automation and standardization for clinical application and precision in deposition of cells. Although conventional tissue engineering strategies (i.e., culturing cells on a scaffold and maturation in a bioreactor) might currently achieve similar results to bioprinting, there are still many aspects that require improvements in the production process of the skin, including the long production times to obtain large surfaces required to cover the entire burn wounds [67]. There are two different approaches to skin bioprinting: in situ bioprinting and in vitro bioprinting. Both these approaches are similar except for the site of printing and tissue maturation. In situ bioprinting involves direct printing of pre-cultured cells onto the site of injury for wound closure allowing for skin maturation at the wound site. The use of in situ bioprinting for burn wound reconstruction provides several advantages, including precise deposition of cells on the wound, elimination of the need for expensive and time-consuming in vitro differentiation, and the need for multiple surgeries [68]. In the case of in vitro bioprinting, printing is done in vitro and the bioprinted skin is allowed to mature in a bioreactor, after which it is transplanted to the wound site. Our group is working on developing approaches for in situ bioprinting [69]. An inkjet-based bioprinting system was developed to print primary human keratinocytes and fibroblasts on dorsal full-thickness (3 cm Ă 2.5 cm) wounds in athymic nude mice. First, fibroblasts (1.0 Ă 105 cells/cm2) incorporated into fibrinogen/collagen hydrogels were printed on the wounds, followed by a layer of keratinocytes (1.0 Ă 107 cells/cm2) above the fibroblast layer [69]. Complete re-epithelialization was achieved in these relatively large wounds after 8 weeks. This bioprinting system involves the use of a novel cartridge-based delivery system for deposition of cells at the site of injury. A laser scanner scans the wound and creates a map of the missing skin, and fibroblasts and keratinocytes are printed directly on to this area. These cells then form the dermis and epidermis, respectively. This was further validated in a pig wound model, wherein larger wounds (10 cm Ă 10 cm) were treated by printing a layer of fibroblasts followed by keratinocytes (10 million cells each) [69]. Wound healing and complete re-epithelialization were observed by 8 weeks. This pivotal work shows the potential of using in situ bioprinting approaches for wound healing and skin regeneration. Clinical studies are currently in progress with this in situ bioprinting system. In another study, amniotic fluid-derived stem cells (AFSCs) were bioprinted directly onto full-thickness dorsal skin wounds (2 cm Ă 2 cm) of nu/nu mice using a pressure-driven, computer-controlled bioprinting device [44]. AFSCs and bone marrow-derived mesenchymal stem cells were suspended in fibrin-collagen gel, mixed with thrombin solution (a crosslinking agent), and then printed onto the wound site. Two layers of fibrin-collagen gel and thrombin were printed on the wounds. Bioprinting enabled effective wound closure and re-epithelialization likely through a growth factor-mediated mechanism by the stem cells. These studies indicate the potential of using in situ bioprinting for treatment of large wounds and burns.