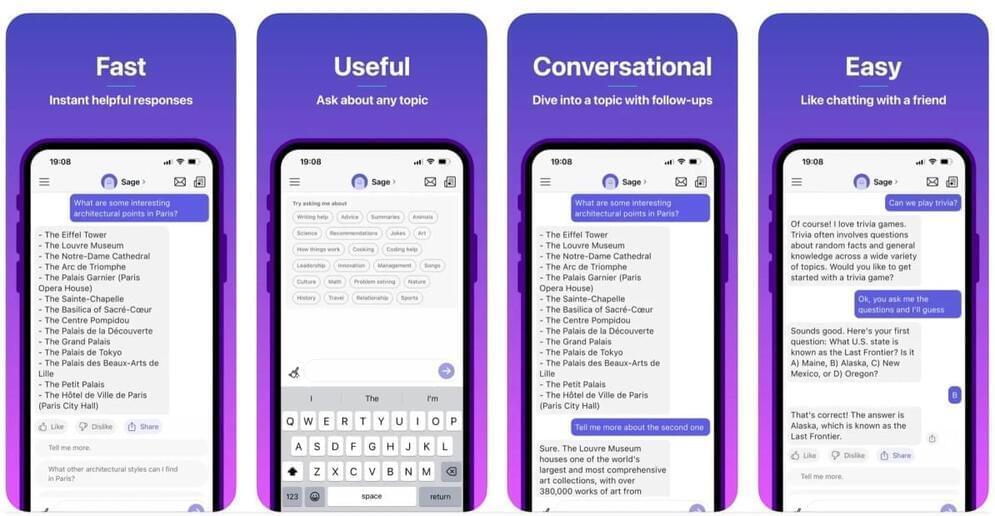
Q&A platform Quora has opened up public access to its new AI chatbot app, Poe, which lets users ask questions and get answers from a range of AI chatbots, including those from ChatGPT maker, OpenAI, and other companies like Anthropic. Beyond allowing users to experiment with new AI technologies, Poe’s content will ultimately help to evolve Quora itself, the company says.
Quora first announced Poe’s mobile app in December, but at the time, it required an invite to try it out. With the public launch on Friday, anyone can now use Poe’s app. For now, it’s available only to iOS users, but Quora says the service will arrive on other platforms in a few months.
In an announcement, the company explained it decided to launch Poe as a standalone product that’s independent of Quora itself because of how quickly AI developments and changes are now taking place. However, there will be some connections between the Q&A site and Poe. If and when Poe’s content meets a high enough quality standard, it will be distributed on Quora’s site itself, where it has the ability to reach Quora’s 400 million monthly visitors, the company noted.