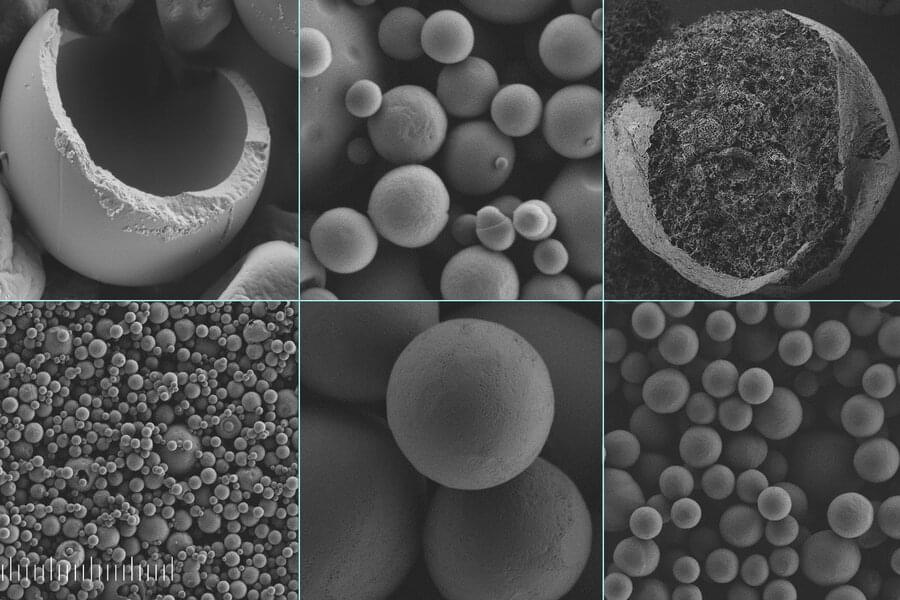
Microplastics, tiny particles of plastic that are now found worldwide in the air, water, and soil, are increasingly recognized as a serious pollution threat, and have been found in the bloodstream of animals and people around the world.
Some of these microplastics are intentionally added to a variety of products, including agricultural chemicals, paints, cosmetics, and detergents—amounting to an estimated 50,000 tons a year in the European Union alone, according to the European Chemicals Agency. The EU has already declared that these added, nonbiodegradable microplastics must be eliminated by 2025, so the search is on for suitable replacements, which do not currently exist.
Now, a team of scientists at MIT and elsewhere has developed a system based on silk that could provide an inexpensive and easily manufactured substitute. The new process is described in a paper in the journal Small, written by MIT postdoc Muchun Liu, MIT professor of civil and environmental engineering Benedetto Marelli, and five others at the chemical company BASF in Germany and the U.S.