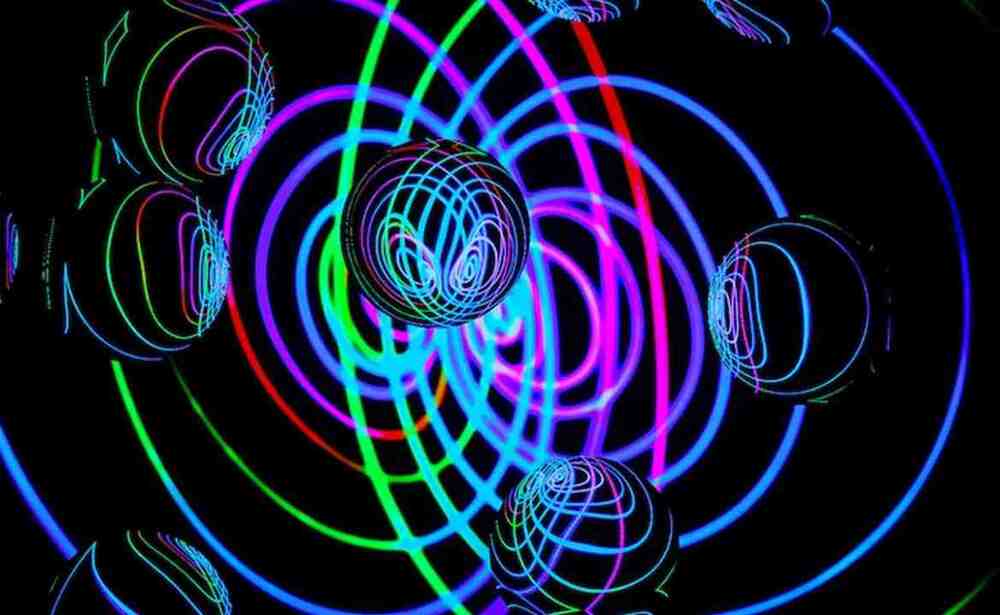
A team of physicists, including University of Massachusetts assistant professor Tigran Sedrakyan, recently announced in the journal Nature that they have discovered a new phase of matter. Called the “chiral Bose-liquid state,” the discovery opens a new path in the age-old effort to understand the nature of the physical world.
Under everyday conditions, matter can be a solid, liquid or gas. But once you venture beyond the everyday—into temperatures approaching absolute zero, things smaller than a fraction of an atom or which have extremely low states of energy—the world looks very different. “You find quantum states of matter way out on these fringes,” says Sedrakyan, “and they are much wilder than the three classical states we encounter in our everyday lives.”
Sedrakyan has spent years exploring these wild quantum states, and he is particularly interested in the possibility of what physicists call “band degeneracy,” “moat bands” or “kinetic frustration” in strongly interacting quantum matter.