It outmatches GPT-4 in almost all ways—but only by a little. Was the buzz worth it?
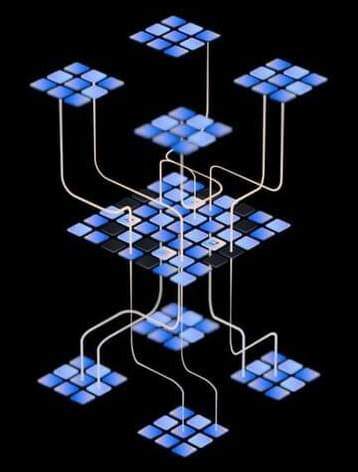
Summary: Researchers developed an AI-based method to track neurons in moving and deforming animals, a significant advancement in neuroscience research. This convolutional neural network (CNN) method overcomes the challenge of tracking brain activity in organisms like worms, whose bodies constantly change shape.
By employing ‘targeted augmentation’, the AI significantly reduces the need for manual image annotation, streamlining the neuron identification process. Tested on the roundworm Caenorhabditis elegans, this technology has not only increased analysis efficiency but also deepened insights into complex neuronal behaviors.
After months of teasing us, Google is starting to roll out its generative artificial intelligence model, Gemini.
The new model, which will be launched in phases, is Google’s chance to thwart the narrative that it’s fallen behind rivals such as OpenAI.
But while users will have access to Gemini this month, the most advanced version of the model won’t arrive until early next year.
Scientists have created tiny, self-assembling robots made from human cells that could one day repair damaged skin and tissue.
These tiny biological machines, called Anthrobots, are made from human tracheal cells without any genetic modification. Lab dish experiments revealed they can encourage neurons, or nerve cells, to grow in damaged tissue.
The development of artificial intelligence poses potential risks to society and requires a shift in goal definitions, consideration of the motivational landscape, and wisdom to prevent self-extinction and promote sustainability.
On this episode, Daniel Schmachtenberger returns to discuss a surprisingly overlooked risk to our global systems and planetary stability: artificial intelligence.
Through a systems perspective, Daniel and Nate piece together the biophysical history that has led humans to this point, heading towards (and beyond) numerous planetary boundaries and facing geopolitical risks all with existential consequences.
Recent technological advancements have opened invaluable opportunities for assisting people who are experiencing impairments or disabilities. For instance, they have enabled the creation of tools to support physical rehabilitation, to practice social skills, and to provide daily assistance with specific tasks.
Researchers at Meta AI recently developed a promising and non-invasive method to decode speech from a person’s brain activity, which could allow people who are unable to speak to relay their thoughts via a computer interface. Their proposed method, presented in Nature Machine Intelligence, merges the use of an imaging technique and machine learning.
“After a stroke, or a brain disease, many patients lose their ability to speak,” Jean Remi King, Research Scientist at Meta, told Medical Xpress. “In the past couple of years, major progress has been achieved to develop a neural prosthesis: a device, typically implanted on the motor cortex of the patients, which can be used, through AI, to control a computer interface. This possibility, however, still requires brain surgery, and is thus not without risks.”
X.AI, Elon Musk’s artificial intelligence startup, has filed with the SEC to raise up to $1 billion in an equity offering. The company has raised nearly $135 million from four investors, with the first sale occurring on Nov. 29, according to the filing. The AI startup, which Musk announced in July,…
X.AI, an artificial intelligence startup founded by Elon Musk, has filed with the SEC to raise up to $1 billion in an equity offering.
The company has already brought in nearly $135 million from four investors, with the first sale occurring on Nov. 29, and has a “binding and enforceable agreement” for the purchase of the remaining shares, the filing says.
The AI startup, which Musk announced in July, seeks to “understand the true nature of the universe,” according to its website. Last month, X.AI released a chatbot called Grok, which the company says is modeled after “The Hitchhiker’s Guide to the Galaxy.” The chatbot debuted with two months of training and has real-time knowledge of the internet, the company claims.