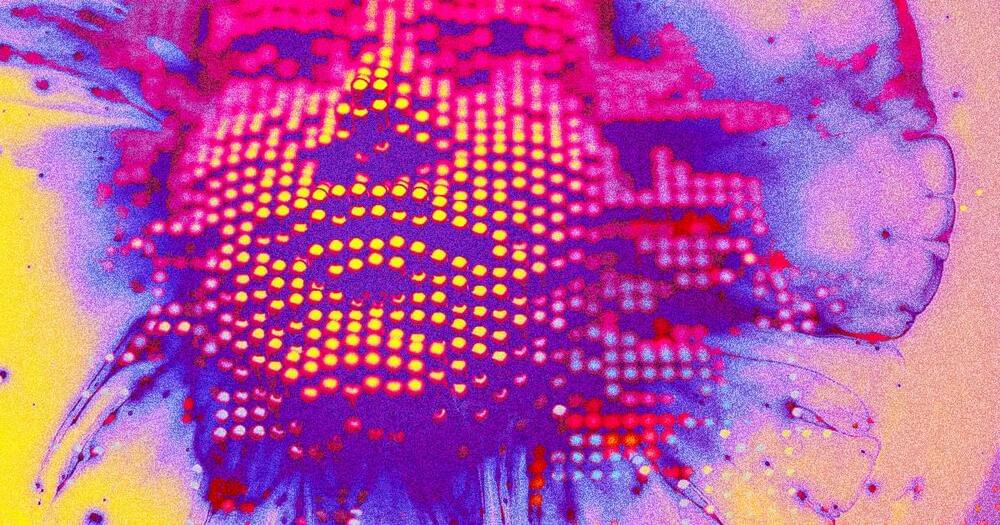
Awkward.
“The prevalence and harms of online misinformation is a perennial concern for internet platforms, institutions and society at large,” reads the paper. “The rise of generative AI-based tools, which provide widely-accessible methods for synthesizing realistic audio, images, video and human-like text, have amplified these concerns.”
The study, first caught by former Googler Alexios Mantzarlis and flagged in the newsletter Faked Up, focused on media-based misinformation, or bad information propagated through visual mediums like images and videos. To narrow the scope of the research, the study focused on media that was fact-checked by the service ClaimReview, ultimately examining a total of 135,838 fact-check-tagged pieces of online media.
As the researchers write in the paper, AI is effective for producing realistic synthetic content quickly and easily, at “a scale previously impossible without an enormous amount of manual labor.” The availability of AI tools, per the researchers’ findings, has led to hockey stick-like growth in AI-generated media online since 2023. Meanwhile, other types of content manipulation decreased in popularity, though “the rise” of AI media “did not produce a bump in the overall proportion” of image-dependant misinformation claims.