Elon Musk has made a rare new comment about Tesla’s now not-so-secret ‘Dojo’ program to create an AI-training supercomputer and gave a hint of its capacity.
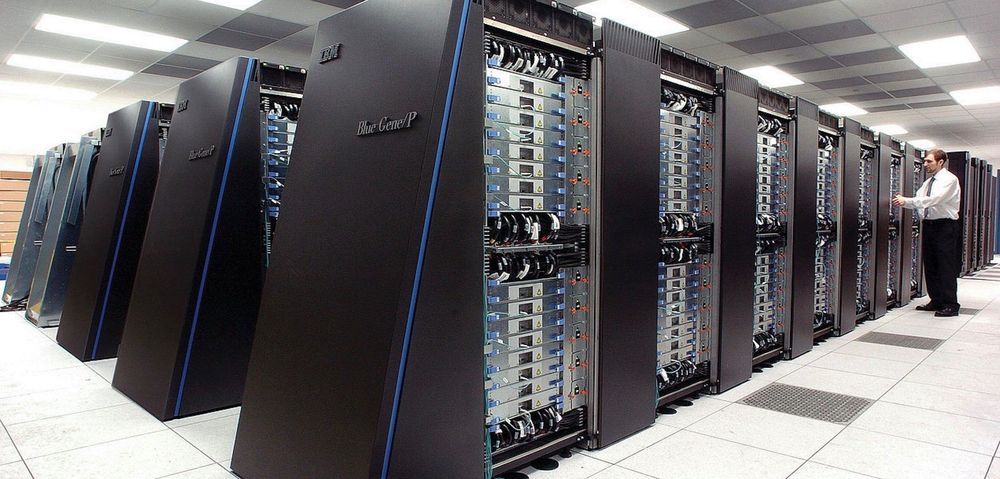
A temperature of 130 degrees Fahrenheit (54.4 degrees Celsius) recorded in California’s Death Valley on Sunday by the US National Weather Service could be the hottest ever measured with modern instruments, officials say.
The reading was registered at 3:41 pm at the Furnace Creek Visitor Center in the Death Valley national park by an automated observation system—an electronic thermometer encased inside a box in the shade.
In 1913, a weather station half an hour’s walk away recorded what officially remains the world record of 134 degrees Fahrenheit (56.7 degrees Celsius). But its validity has been disputed because a superheated sandstorm at the time may have skewed the reading.
Although true “cyborgs”—part human, part robotic beings—are science fiction, researchers are taking steps toward integrating electronics with the body. Such devices could monitor for tumor development or stand in for damaged tissues. But connecting electronics directly to human tissues in the body is a huge challenge. Now, a team is reporting new coatings for components that could help them more easily fit into this environment.
The researchers will present their results today at the American Chemical Society (ACS) Fall 2020 Virtual Meeting & Expo.
“We got the idea for this project because we were trying to interface rigid, inorganic microelectrodes with the brain, but brains are made out of organic, salty, live materials,” says David Martin, Ph.D., who led the study. “It wasn’t working well, so we thought there must be a better way.”
Register at the webinar on AI & Robotics 2020 on August 23, 2020.
Hurry up. Limited Slots are Available to meet our experts & register here: https://meetingsint.com/webinars/ai_robotics/registration
Technology could enable new health diagnostics and achieve Elon Musk’s goal of integrating with artificial intelligence.
The Universe or any other phenomenon or entity contained therein is not objectively real but subjectively real. Patterns of information emerging from the ultimate code are what is more fundamental than particles of matter or space-time continuum itself all of which is levels below the Code. Nature behaves quantum code-theoretically at all levels. It’s hierarchies of quantum networks all the way down and all the way up. Being part of hierarchical quantum neural networks, a conscious observer system possesses a strange quality: collapsing quantum states of entangled conscious entities and having a privileged interpretation of that. From this perspective, entangled conscious agents would be a mirror conscious environment, whereas the quantum observer would be a central node of the entangled network.
“If we accept that the material universe as we know it is not a mechanical system but a virtual reality created by Absolute Consciousness through an infinitely complex orchestration of experiences, what are the practical consequences of this insight?” –Stanislav Grof
Just like absolute idealism, solipsism certainly defies our common sense but the deeper layer of truth is not what first meets the eye. Here’s what Richard Conn Henry and Stephen Palmquist write in their paper “An Experimental Test of Non-local Realism” (2007): “Why do people cling with such ferocity to belief in a mind-independent reality? It is surely because if there is no such reality (as far as we can know) mind alone exists. And if mind is not a product of real matter, but rather is the creator of the illusion of material reality (which has, in fact, despite the materialists, been known to be the case, since the discovery of quantum mechanics in 1925), then a theistic view of our existence becomes the only rational alternative to solipsism.” One can extend their line of reasoning by arriving at pantheistic solipsism as a likely revelation to ponder about.
Our minds operate in the domains of subjectivity, intersubjectivity and supersubjectivity. In the domain of intersubjectivity, minds create a reality by sharing “mindspace,” i.e. shared belief systems and ways of communication, minds then inhabit the reality which they have created. At the level of your individual mind, i.e. local consciousness, you play a multi-level virtual reality game of life but we all invariably converge at the Omega Singularity by forging our own discrete pathways to the ultimate divine. As you’re reading this right now, you’re now in your own subjective reality tunnel leading to the Source and back where you’re now all of which is definable as a parallel evolutionary feedback process within non-local holistic consciousness patterning this virtual multiverse.
We can’t evolve faster than our language does. Evolution is a linguistic, code-theoretic process. Do yourself a humongous favor, look over these 33 transhumanist neologisms. Here’s a fairly comprehensive glossary of thirty three newly-introduced concepts and terms from “The Syntellect Hypothesis: Five Paradigms of the Mind’s Evolution” by futurist, philosopher and evolutionary cyberneticist Alex M. Vikoulov. In parts written as an academic paper, in parts as a belletristic masterpiece, this recent book is an exceptionally easy read for an intellectual reader — a philosophical treatise that is fine-tuned with apt neologisms readily explained by given definitions and contextually… https://medium.com/@alexvikoulov/33-crucial-terms-every-futu…a1c8b993c8
#evolution #consciousness #futurism #transhumanism #philosophy
“A powerful work! As a transhumanist, I especially loved one of the main ideas of the book that the Syntellect Emergence, merging of us into one Global Mind, constitutes the quintessence of the coming Technological Singularity. The novel conceptual visions of mind-uploading and achieving digital immortality are equally fascinating. The Chrysalis Conjecture as a solution to the Fermi Paradox is mind-bending. I would highly recommend The Syntellect Hypothesis to anyone with transhumanist aspirations and exponential thinking!” -Zoltan Istvan, futurist, author, founder of the U.S. Transhumanist Party
Terms such as ‘Artificial Intelligence’ or ‘Neurotechnology’ were new some time not so long ago. We can’t evolve faster than our language does. Evolution is a linguistic, code-theoretic process. Do yourself a humongous favor, look over these 33 transhumanist neologisms. Here’s a fairly comprehensive glossary of thirty three newly-introduced concepts and terms from “The Syntellect Hypothesis: Five Paradigms of the Mind’s Evolution” by futurist, philosopher and evolutionary cyberneticist Alex M. Vikoulov. In parts written as an academic paper, in parts as a belletristic masterpiece, this recent book is an exceptionally easy read for an intellectual reader — a philosophical treatise that is fine-tuned with apt neologisms readily explained by given definitions and contextually:
AGI Naturalization Protocol, AGI(NP) — initiating AGI (Artificial General Intelligence) via human life simulation training program, infusing AGI with a value system, ethics, morality and generally civilized manners to ensure functioning in the best interests of society as a self-aware agent. Read more: http://www.ecstadelic.net/top-stories/how-to-create-friendly…-explosion #AGINaturalizationProtocol #AGINP
As transhumanists, we aim at the so-called continuity of subjectivity by the means of advanced technologies. Death in a common sense of the word becomes optional and cybernetic immortality is within our reach during our lifetimes. By definition, posthumanism (I choose to call it ‘cyberhumanism’) is to replace transhumanism at the center stage circa 2035. By then, mind uploading could become a reality with gradual neuronal replacement, rapid advancements in Strong AI, massively parallel computing, and nanotechnology allowing us to directly connect our brains to the Cloud-based infrastructure of the Global Brain. Via interaction with our AI assistants, the GB will know us better than we know ourselves in all respects, so mind-transfer, or rather “mind migration,” for billions of enhanced humans would be seamless, sometime by mid-century.
By 2040, mind-uploading may become a norm and a fact of life with a “critical mass” of uploads and cybernetic immortality. Any container with a sufficiently integrated network of information patterns, with a certain optimal complexity, especially complex dynamical systems with biological or artificial brains (say, the coming AGIs) could be filled with consciousness at large in order to host an individual “reality cell,” “unit,” or a “node” of consciousness. This kind of individuated unit of consciousness is always endowed with free will within the constraints of the applicable set of rules (“physical laws”), influenced by the larger consciousness system dynamics. Isn’t too naïve to presume that Universal Consciousness would instantiate phenomenality only in the form of “bio”-logical avatars?
Oak Ridge National Laboratory researchers have developed artificial intelligence software for powder bed 3D printers that assesses the quality of parts in real time, without the need for expensive characterization equipment.
The software, named Peregrine, supports the advanced manufacturing “digital thread” being developed at ORNL that collects and analyzes data through every step of the manufacturing process, from design to feedstock selection to the print build to material testing.
“Capturing that information creates a digital ‘clone’ for each part, providing a trove of data from the raw material to the operational component,” said Vincent Paquit, who leads advanced manufacturing data analytics research as part of ORNL’s Imaging, Signals and Machine Learning group. “We then use that data to qualify the part and to inform future builds across multiple part geometries and with multiple materials, achieving new levels of automation and manufacturing quality assurance.”